The examine investigates the emergence of clever conduct in synthetic methods by inspecting how the complexity of rule-based methods influences the capabilities of fashions skilled to foretell these guidelines. Historically, AI growth has centered on coaching fashions utilizing datasets that mirror human intelligence, similar to language corpora or expert-annotated knowledge. This methodology assumes that intelligence can solely emerge from publicity to inherently clever knowledge. Nonetheless, this examine explores an alternate idea, suggesting that intelligence may emerge from fashions skilled on easy methods that generate advanced behaviors, even when the underlying course of lacks inherent intelligence.
The idea of complexity rising from easy methods has been explored in foundational research on mobile automata (CA), the place even minimal guidelines can produce intricate patterns. Analysis by Wolfram and others demonstrated that methods working on the fringe of chaos—the place order and dysfunction meet—exhibit increased computational capabilities. Research have proven that advanced behaviors can come up from easy guidelines, offering a framework for understanding how intelligence may develop from publicity to complexity somewhat than clever knowledge alone. Latest developments in LLMs additionally spotlight the significance of coaching on advanced knowledge for the emergence of recent capabilities, underscoring that each mannequin measurement and the complexity of the information play a major function in intelligence growth.
Researchers from Yale, Columbia, Northwestern, and Idaho State Universities explored how complexity in rule-based methods influences the intelligence of fashions skilled to foretell these guidelines. Utilizing elementary mobile automata (ECA), easy one-dimensional methods with various levels of complexity, they skilled separate GPT-2 fashions on knowledge generated by ECAs. The examine revealed a robust hyperlink between the complexity of ECA guidelines and the fashions’ intelligence, demonstrated by means of improved efficiency on reasoning and chess prediction duties. Their findings counsel that intelligence could emerge from the power to foretell advanced methods, notably these on the “fringe of chaos.”
The examine explored the hyperlink between system complexity and intelligence by coaching modified GPT-2 fashions on binary knowledge generated from ECA. The ECAs have been simulated over 1,000 time steps, producing sequences of binary vectors. The fashions have been pretrained on next-token prediction for as much as 10,000 epochs, utilizing a modified structure to deal with binary inputs and outputs. Coaching sequences have been randomly sampled, and the Adam optimizer with gradient clipping and studying charge scheduling was used to make sure environment friendly coaching. After pretraining, the fashions have been evaluated on reasoning and chess transfer prediction duties.
The examine examines how system complexity impacts the intelligence of LLMs. Outcomes point out that fashions pretrained on extra advanced ECA guidelines carry out higher on duties like reasoning and chess transfer prediction, however extreme complexity, similar to chaotic guidelines, can scale back efficiency. Fashions skilled on advanced guidelines combine previous data for forecasts, as their consideration patterns present. Surprisingly, fashions predicting the subsequent state outperformed these predicting 5 steps, suggesting that advanced fashions study nontrivial patterns. General, there seems to be an optimum stage of complexity that enhances mannequin intelligence and generalization skills.
In conclusion, the examine explores how intelligence emerges in LLMs skilled on ECA with various rule complexity. The outcomes present that fashions skilled on guidelines with average complexity—neither too easy nor too chaotic—carry out higher on duties like reasoning and chess predictions. This helps the “fringe of chaos” idea, the place intelligence develops in methods balancing predictability and complexity. The examine means that fashions study higher by leveraging historic data in advanced duties and that intelligence could emerge from publicity to methods with simply the suitable stage of complexity.
Try the Paper. All credit score for this analysis goes to the researchers of this venture. Additionally, don’t neglect to observe us on Twitter and be part of our Telegram Channel and LinkedIn Group. For those who like our work, you’ll love our e-newsletter.. Don’t Overlook to hitch our 50k+ ML SubReddit.
[Upcoming Live Webinar- Oct 29, 2024] The Finest Platform for Serving Wonderful-Tuned Fashions: Predibase Inference Engine (Promoted)
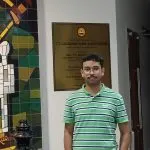
Sana Hassan, a consulting intern at Marktechpost and dual-degree scholar at IIT Madras, is keen about making use of know-how and AI to deal with real-world challenges. With a eager curiosity in fixing sensible issues, he brings a contemporary perspective to the intersection of AI and real-life options.