Basis fashions present spectacular capabilities throughout duties and modalities, outperforming conventional AI approaches usually task-specific and restricted by modality. In drugs, nonetheless, growing such fashions faces challenges on account of restricted entry to numerous information and strict privateness legal guidelines. Whereas succesful in particular areas, current medical basis fashions have to be improved by their give attention to specific duties and modalities. The restrictions embody difficulties in centralized coaching on account of privateness legal guidelines like HIPAA and GDPR and restricted adaptability throughout features. Federated studying presents an answer, enabling decentralized mannequin improvement with out sharing delicate information immediately whereas incorporating broader medical information, which stays an ongoing problem.
Basis fashions, with huge parameters and datasets, have grow to be outstanding in healthcare, providing options for duties like illness detection and precision oncology. Regardless of these advances, medical basis fashions are restricted by the complexities of healthcare information. Federated studying (FL) permits fine-tuning basis fashions with regionally saved information, supporting full or parameter-efficient fine-tuning (PEFT) strategies like Low-Rank Adaptation (LoRA), which reduces computational calls for by factorizing parameters. Whereas Combination of Specialists (MOE) approaches additional refine PEFT for advanced duties, current strategies don’t absolutely deal with the various, multimodal wants distinctive to healthcare settings.
Researchers from Pennsylvania State College and Georgia State College have developed FEDKIM, an modern information injection methodology to develop medical basis fashions inside a federated studying framework. FEDKIM makes use of light-weight native fashions to collect healthcare insights from personal information, that are included right into a centralized basis mannequin. That is achieved by means of the Multitask Multimodal Combination of Specialists (M3OE) module, which adapts to completely different medical duties and modalities whereas safeguarding information privateness. Experiments on twelve duties throughout seven modalities verify FEDKIM’s functionality to scale medical basis fashions successfully, even with out direct entry to delicate information.
The FEDKIM framework contains two most important elements: native shopper information extractors and a central server-side information injector. Every shopper, representing a hospital or medical institute, trains a multimodal, multi-task mannequin on personal information, which is then shared with the server. These shopper parameters are aggregated and injected right into a central medical basis mannequin on the server, enhanced with a Multitask M3OE module. This module dynamically selects skilled techniques for every task-modality pair, permitting FEDKIM to deal with advanced medical eventualities. This iterative course of updates native and server fashions, enabling environment friendly information integration and privateness preservation.
The research assesses FEDKIM’s efficiency by means of zero-shot and fine-tuning evaluations. In zero-shot assessments, the place coaching and analysis duties differ, FEDKIM outperformed baselines like FedPlug and FedPlugL, notably in dealing with unseen duties, on account of its M3OE module that selects specialists adaptively. FEDKIM additionally confirmed robust efficiency with each FedAvg and FedProx backbones, although FedProx usually enhanced outcomes. Nice-tuning analysis on identified duties confirmed FEDKIM’s superior efficiency, particularly over FedPlug variants, as information injected by means of federated studying proved beneficial. Ablation research underscored the need of FEDKIM’s modules, validating their significance in dealing with advanced healthcare duties and modalities.
In conclusion, the research introduces FEDKIM, an strategy for enhancing medical basis fashions by means of information injection. FEDKIM makes use of federated studying to extract information from safely distributed personal healthcare information. It integrates it right into a central mannequin utilizing the M3OE module, which adapts to deal with numerous duties and modalities. This method addresses challenges in medical AI, similar to privateness constraints and restricted information entry, whereas enhancing mannequin efficiency throughout advanced duties. Experimental outcomes throughout 12 duties and 7 modalities verify FEDKIM’s effectiveness, highlighting its potential for constructing complete, privacy-preserving healthcare fashions with out direct entry to delicate information.
Try the Paper. All credit score for this analysis goes to the researchers of this challenge. Additionally, don’t neglect to comply with us on Twitter and be a part of our Telegram Channel and LinkedIn Group. For those who like our work, you’ll love our e-newsletter.. Don’t Neglect to affix our 55k+ ML SubReddit.
[Sponsorship Opportunity with us] Promote Your Analysis/Product/Webinar with 1Million+ Month-to-month Readers and 500k+ Group Members
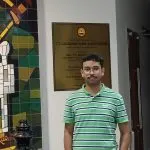
Sana Hassan, a consulting intern at Marktechpost and dual-degree scholar at IIT Madras, is enthusiastic about making use of expertise and AI to handle real-world challenges. With a eager curiosity in fixing sensible issues, he brings a recent perspective to the intersection of AI and real-life options.