Multi-label textual content classification (MLTC) assigns a number of related labels to a textual content. Whereas deep studying fashions have achieved state-of-the-art outcomes on this space, they require giant quantities of labeled information, which is dear and time-consuming. Lively studying helps optimize this course of by choosing essentially the most informative unlabeled samples for annotation, lowering the labeling effort. Nonetheless, most present energetic studying strategies are designed for conventional single-label fashions and don’t instantly apply to deep multi-label fashions. Given the complexity of multi-label duties and the excessive value of annotations, there’s a want for energetic studying strategies tailor-made to deep multi-label classification.
Lively studying allows a mannequin to request labels for essentially the most informative unlabeled samples, lowering annotation prices. Widespread energetic studying approaches embody membership question synthesis, stream-based selective sampling, and pool-based sampling, specializing in the latter on this work. Uncertainty-based sampling is usually utilized in multi-label classification, however challenges nonetheless should be solved in making use of energetic studying to deep multi-label fashions. Whereas Bayesian deep studying strategies have proven promise for uncertainty estimation, most analysis has centered on single-label duties.
Researchers from the Institute of Automation, Chinese language Academy of Sciences, and different establishments suggest BEAL, a deep energetic studying technique for MLTC. BEAL makes use of Bayesian deep studying with dropout to deduce the mannequin’s posterior predictive distribution and introduces a brand new anticipated confidence-based acquisition operate to pick unsure samples. Experiments with a BERT-based MLTC mannequin on benchmark datasets like AAPD and StackOverflow present that BEAL improves coaching effectivity, reaching convergence with fewer labeled samples. This technique could be prolonged to different multi-label classification duties and considerably reduces labeled information necessities in comparison with present strategies.
The methodology introduces a batch-mode energetic studying framework for deep multi-label textual content classification. Beginning with a small labeled dataset, the framework iteratively selects unlabeled samples for annotation primarily based on an acquisition operate. This operate chooses samples with the bottom anticipated confidence, measured by the mannequin’s predictive uncertainty. Bayesian deep studying calculates the posterior predictive distribution utilizing Monte Carlo dropout, approximating the mannequin’s confidence. The acquisition operate selects a batch of samples with the bottom anticipated confidence for labeling, bettering the mannequin’s effectivity by lowering the necessity for labeled information. The method continues till the mannequin’s efficiency converges.
On this examine, the authors consider the BEAL technique for deep multi-label textual content classification utilizing two benchmark datasets: AAPD and StackOverflow. The method is in contrast with a number of energetic studying methods, together with random sampling, BADGE, BALD, Core-Set, and the full-data strategy. BEAL outperforms these strategies by choosing essentially the most informative samples primarily based on posterior predictive distribution, lowering the necessity for labeled information. Outcomes present that BEAL achieves the best efficiency with fewer labeled samples than others, requiring solely 64% of labeled samples on AAPD and 40% on StackOverflow. An ablation examine highlights the benefit of utilizing Bayesian deep studying in BEAL.
In conclusion, the examine introduces BEAL, an energetic studying technique for deep MLTC fashions. BEAL makes use of Bayesian deep studying to deduce the posterior predictive distribution and defines an anticipated confidence-based acquisition operate to pick unsure samples for coaching. Experimental outcomes present that BEAL outperforms different energetic studying strategies, enabling extra environment friendly mannequin coaching with fewer labeled samples. That is priceless in real-world purposes the place acquiring large-scale labeled information is tough. Future work will discover integrating diversity-based strategies to cut back additional the labeled information required for efficient coaching of MLTC fashions.
Take a look at the Paper. All credit score for this analysis goes to the researchers of this challenge. Additionally, don’t neglect to observe us on Twitter and be a part of our Telegram Channel and LinkedIn Group. In case you like our work, you’ll love our e-newsletter.. Don’t Neglect to hitch our 55k+ ML SubReddit.
[FREE AI WEBINAR] Implementing Clever Doc Processing with GenAI in Monetary Companies and Actual Property Transactions– From Framework to Manufacturing
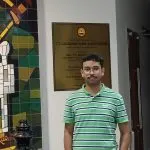
Sana Hassan, a consulting intern at Marktechpost and dual-degree pupil at IIT Madras, is keen about making use of expertise and AI to deal with real-world challenges. With a eager curiosity in fixing sensible issues, he brings a contemporary perspective to the intersection of AI and real-life options.