The event of vision-language fashions (VLMs) has confronted challenges in dealing with advanced visible question-answering duties. Regardless of substantial advances in reasoning capabilities by giant language fashions like OpenAI’s GPT-o1, VLMs nonetheless wrestle with systematic and structured reasoning. Present fashions usually lack the flexibility to prepare data and interact in logical, sequential reasoning, limiting their effectiveness for duties that require deep cognitive processing, notably when coping with multimodal inputs equivalent to pictures mixed with textual content. Conventional VLMs are inclined to generate speedy responses and not using a step-by-step reasoning strategy, resulting in errors and inconsistencies.
Meet LLaVA-o1
A group of researchers from Peking College, Tsinghua College, Peng Cheng Laboratory, Alibaba DAMO Academy, and Lehigh College has launched LLaVA-o1: a visible language mannequin able to systematic reasoning, much like GPT-o1. LLaVA-o1 is an 11-billion-parameter mannequin designed for autonomous, multistage reasoning. It builds upon the Llama-3.2-Imaginative and prescient-Instruct mannequin and introduces a structured reasoning course of, addressing the constraints of earlier VLMs with a extra methodical strategy. The important thing innovation in LLaVA-o1 is the implementation of 4 distinct reasoning phases: abstract, caption, reasoning, and conclusion.
The mannequin is fine-tuned utilizing a dataset referred to as LLaVA-o1-100k, derived from visible query answering (VQA) sources and structured reasoning annotations generated by GPT-4o. This allows LLaVA-o1 to carry out multistage reasoning, extending capabilities much like GPT-o1 into vision-language duties, which have traditionally lagged behind text-based fashions.
Technical Particulars and Advantages
LLaVA-o1 employs a novel inference-time scaling method referred to as stage-level beam search. Not like earlier strategies, equivalent to best-of-N or sentence-level beam search, LLaVA-o1 generates a number of responses for every stage of its structured reasoning course of and selects the perfect candidate at every step, making certain higher-quality outcomes. This structured strategy maintains logical coherence all through the reasoning course of, resulting in extra correct conclusions.
Advantageous-tuned from the Llama-3.2-11B-Imaginative and prescient-Instruct mannequin, LLaVA-o1 exhibits an 8.9% enchancment on multimodal reasoning benchmarks in comparison with its base mannequin, even outperforming bigger or closed-source rivals like Gemini-1.5-pro, GPT-4o-mini, and Llama-3.2-90B-Imaginative and prescient-Instruct. It achieves this with solely 100,000 coaching samples, making LLaVA-o1 an environment friendly resolution by way of each efficiency and scalability. By using structured considering by way of distinct phases, LLaVA-o1 systematically addresses issues, minimizing reasoning errors frequent in different VLMs.
Significance and Outcomes
LLaVA-o1 addresses a big hole between textual and visible question-answering fashions by enabling systematic reasoning in vision-language duties. Experimental outcomes present that LLaVA-o1 improves efficiency throughout benchmarks like MMStar, MMBench, MMVet, MathVista, AI2D, and HallusionBench. It constantly surpasses its base mannequin by over 6.9% throughout multimodal benchmarks, notably in reasoning-intensive domains equivalent to mathematical and scientific visible questions.

Stage-level beam search enhances the mannequin’s reliability by producing and verifying a number of candidate responses for every stage, deciding on essentially the most applicable one. This permits LLaVA-o1 to excel in advanced visible duties, in comparison with conventional inference scaling strategies that may be inefficient. LLaVA-o1 demonstrates that structured responses are essential for reaching high-quality, constant reasoning, setting a brand new normal for equally sized fashions.
Conclusion
LLaVA-o1 is a visible language mannequin able to systematic reasoning, much like GPT-o1. Its four-stage reasoning construction, mixed with stage-level beam search, units a brand new benchmark for multimodal AI. By coaching on a comparatively small but strategically constructed dataset, LLaVA-o1 demonstrates that environment friendly and scalable multimodal reasoning is achievable with out the huge sources required by bigger closed-source fashions. LLaVA-o1 paves the way in which for future analysis on structured reasoning inside vision-language fashions, promising extra superior capabilities in AI-driven cognitive processing throughout visible and textual domains.
Try the Paper and GitHub Web page. All credit score for this analysis goes to the researchers of this mission. Additionally, don’t overlook to observe us on Twitter and be a part of our Telegram Channel and LinkedIn Group. In case you like our work, you’ll love our e-newsletter.. Don’t Neglect to affix our 55k+ ML SubReddit.
Why AI-Language Fashions Are Nonetheless Weak: Key Insights from Kili Expertise’s Report on Massive Language Mannequin Vulnerabilities [Read the full technical report here]
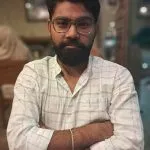
Nikhil is an intern marketing consultant at Marktechpost. He’s pursuing an built-in twin diploma in Supplies on the Indian Institute of Expertise, Kharagpur. Nikhil is an AI/ML fanatic who’s all the time researching functions in fields like biomaterials and biomedical science. With a robust background in Materials Science, he’s exploring new developments and creating alternatives to contribute.