Basis fashions (FMs) and huge language fashions (LLMs) are revolutionizing AI purposes by enabling duties akin to textual content summarization, real-time translation, and software program improvement. These applied sciences have powered the event of autonomous brokers that may carry out advanced decision-making and iterative processes with minimal human intervention. Nonetheless, as these methods sort out more and more multifaceted duties, they require sturdy observability, traceability, and compliance mechanisms. Making certain their reliability has turn out to be important, particularly because the demand for FM-based autonomous brokers grows throughout academia and trade.
A significant hurdle in FM-based autonomous brokers is their want for constant traceability and observability throughout operational workflows. These brokers depend on intricate processes, integrating numerous instruments, reminiscence modules, and decision-making capabilities to carry out their duties. This complexity typically results in suboptimal outputs which can be troublesome to debug and proper. Regulatory necessities, such because the EU AI Act, add one other layer of complexity by demanding transparency and traceability in high-risk AI methods. Compliance with such frameworks is significant for gaining belief and guaranteeing the moral deployment of AI methods.
Present instruments and frameworks present partial options however must ship end-to-end observability. As an illustration, LangSmith and Arize supply options for monitoring agent prices and bettering latency however fail to handle the broader life-cycle traceability required for debugging and compliance. Equally, frameworks akin to SuperAGI and CrewAI allow multi-agent collaboration and agent customization however lack sturdy mechanisms for monitoring decision-making pathways or tracing errors to their supply. These limitations urgently require instruments that may present complete oversight all through the agent manufacturing life-cycle.
Researchers at CSIRO’s Data61, Australia, performed a fast evaluate of instruments and methodologies within the AgentOps ecosystem to handle these gaps. Their research examined present AgentOps instruments and recognized key options for reaching observability and traceability in FM-based brokers. Based mostly on their findings, the researchers proposed a complete overview of observability knowledge and traceable artifacts that span all the agent life cycle. Their evaluate underscores the significance of those instruments in guaranteeing system reliability, debugging, and compliance with regulatory frameworks such because the EU AI Act.
The methodology employed within the research concerned an in depth evaluation of instruments supporting the AgentOps ecosystem. The researchers recognized observability and traceability as core parts for enhancing the reliability of FM-based brokers. AgentOps instruments permit builders to watch workflows, document LLM interactions, and hint exterior instrument utilization. Reminiscence modules had been highlighted as essential for sustaining each short-term and long-term context, enabling brokers to supply coherent outputs in multi-step duties. One other vital function is the combination of guardrails, which implement moral and operational constraints to information brokers towards reaching their predefined goals. Observability options like artifact tracing and session-level analytics had been important for real-time monitoring and debugging.
The research revealed outcomes that emphasize the effectiveness of AgentOps instruments in addressing the challenges of FM-based brokers. These instruments guarantee compliance with the EU AI Act’s Articles 12, 26, and 79 by implementing complete logging and monitoring capabilities. Builders can hint each choice made by the agent, from preliminary consumer inputs to intermediate steps and last outputs. This degree of traceability not solely simplifies debugging but in addition enhances transparency in agent operations. Observability instruments inside the AgentOps ecosystem additionally allow efficiency optimization by way of session-level analytics and actionable insights, serving to builders refine workflows and enhance effectivity. Though particular numerical enhancements weren’t supplied within the paper, the power of those instruments to streamline processes and improve system reliability was persistently emphasised.
The findings by CSIRO’s Data61 researchers present a scientific overview of the AgentOps panorama and its potential to remodel FM-based agent improvement. Their evaluate presents beneficial insights for builders and stakeholders trying to deploy dependable and compliant AI methods by specializing in observability and traceability. The research underscores the significance of integrating these capabilities into AgentOps platforms, which function a basis for constructing scalable, clear, and reliable autonomous brokers. Because the demand for FM-based brokers continues to develop, the methodologies and instruments outlined on this analysis set a benchmark for future developments.
Take a look at the Paper. All credit score for this analysis goes to the researchers of this mission. Additionally, don’t overlook to observe us on Twitter and be a part of our Telegram Channel and LinkedIn Group. For those who like our work, you’ll love our e-newsletter.. Don’t Neglect to hitch our 55k+ ML SubReddit.
[FREE AI WEBINAR] Implementing Clever Doc Processing with GenAI in Monetary Providers and Actual Property Transactions– From Framework to Manufacturing
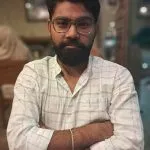
Nikhil is an intern guide at Marktechpost. He’s pursuing an built-in twin diploma in Supplies on the Indian Institute of Know-how, Kharagpur. Nikhil is an AI/ML fanatic who’s all the time researching purposes in fields like biomaterials and biomedical science. With a robust background in Materials Science, he’s exploring new developments and creating alternatives to contribute.