Multimodal language fashions (LMMs) are a transformative know-how that blends pure language processing with visible information interpretation. Their purposes lengthen to multilingual digital assistants, cross-cultural data retrieval, and content material understanding. By combining linguistic comprehension and picture evaluation, LMMs promise enhanced accessibility to digital instruments, particularly in linguistically various and visually wealthy contexts. Nevertheless, their effectiveness hinges on their skill to adapt to cultural and linguistic nuances, a difficult activity given the range of world languages and traditions.
One of many crucial challenges on this subject is the necessity for extra efficiency of LMMs in low-resource languages and culturally particular contexts. Whereas many fashions excel in high-resource languages like English and Mandarin, they falter with languages equivalent to Amharic or Sinhala, which have restricted coaching information. Moreover, cultural information is usually underrepresented, with present fashions needing assist decoding traditions, rituals, or domain-specific data. These limitations scale back the inclusivity and utility of LMMs for international populations.
Benchmarks for evaluating LMMs have traditionally wanted to be improved. CulturalVQA and Henna benchmarks, for example, cowl a restricted variety of languages and cultural domains. CulturalVQA focuses totally on English and culturally particular content material, whereas Henna addresses cultural points in Arabic throughout 11 nations however wants extra breadth in area and language variety. Present datasets are sometimes skewed in the direction of high-resource languages and single-question codecs, incompletely evaluating a mannequin’s cultural and linguistic skills.
Researchers from the College of Central Florida, Mohamed bin Zayed College of AI, Amazon, Aalto College, Australian Nationwide College, and Linköping College launched the All Languages Matter Benchmark (ALM-bench) to deal with these shortcomings. This intensive framework evaluates LMMs throughout 100 languages from 73 nations, together with high- and low-resource languages. The benchmark encompasses 24 scripts and 19 cultural and generic domains, guaranteeing complete linguistic and cultural illustration.
The methodology behind ALM-bench is rigorous and data-driven. It contains over 22,763 manually verified question-answer pairs, categorized into 6,000 normal VQA pairs and 16,763 culturally particular ones. Query codecs vary from multiple-choice to true/false and visible query answering (VQA), guaranteeing a radical analysis of multimodal reasoning. The information have been collected utilizing GPT-4o translations, later refined by native language consultants, with greater than 800 hours devoted to annotation. Care was taken to incorporate pictures and cultural artifacts representing 13 distinct domains, equivalent to structure, music, festivals, and notable key figures, reflecting cultural depth and variety.
Analysis outcomes revealed vital insights into the efficiency of 16 state-of-the-art LMMs. Proprietary fashions like GPT-4o and Gemini-1.5-Professional outperformed open-source fashions, reaching 78.8% and 74.3% accuracy, respectively. Whereas closed-source fashions excelled in high-resource languages, they confirmed a steep efficiency drop for low-resource ones. For instance, GPT-4o’s accuracy fell from 88.4% for English to 50.8% for Amharic. Open-source fashions like GLM-4V-9B carried out higher than others of their class however remained much less efficient, with an general accuracy of 51.9%. The benchmark additionally highlighted disparities throughout cultural domains, with the perfect ends in training (83.7%) and heritage (83.5%) and weaker efficiency in decoding customs and notable key figures.
This analysis offers a number of crucial takeaways that underscore the importance of ALM-bench in advancing LMM know-how:
- Cultural Inclusivity: ALM-bench units a brand new customary by together with 100 languages and 73 nations, making it probably the most complete benchmark for LMM analysis.
- Sturdy Analysis: The benchmark checks fashions’ skill to motive about complicated linguistic and cultural contexts utilizing various query codecs and domains.
- Efficiency Gaps: The examine recognized a stark distinction between high-resource and low-resource languages, urging extra inclusive mannequin coaching.
- Proprietary vs. Open Supply: Closed-source fashions persistently outperformed open-source counterparts, showcasing the significance of proprietary improvements.
- Mannequin Limitations: Even the perfect fashions struggled with nuanced cultural reasoning, emphasizing the necessity for improved datasets and coaching methodologies.

In conclusion, the ALM-bench analysis sheds mild on the constraints of multimodal language fashions whereas providing a groundbreaking framework for enchancment. By encompassing 22,763 various questions throughout 19 domains and 100 languages, the benchmark fills a crucial hole in evaluating linguistic and cultural inclusivity. It highlights the necessity for innovation to deal with disparities in efficiency between high- and low-resource languages, guaranteeing these applied sciences are extra inclusive and efficient for a world viewers. This work paves the best way for future developments in AI to embrace and mirror the wealthy tapestry of world languages and cultures.
Take a look at the Paper and Venture. All credit score for this analysis goes to the researchers of this undertaking. Additionally, don’t neglect to comply with us on Twitter and be a part of our Telegram Channel and LinkedIn Group. If you happen to like our work, you’ll love our e-newsletter.. Don’t Overlook to affix our 55k+ ML SubReddit.
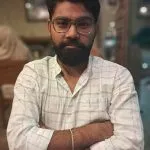
Nikhil is an intern advisor at Marktechpost. He’s pursuing an built-in twin diploma in Supplies on the Indian Institute of Know-how, Kharagpur. Nikhil is an AI/ML fanatic who’s at all times researching purposes in fields like biomaterials and biomedical science. With a powerful background in Materials Science, he’s exploring new developments and creating alternatives to contribute.