Supporting the well being and well-being of various international populations necessitates a nuanced understanding of the complicated relationships between human conduct and native environments. This requires figuring out weak populations and optimizing useful resource allocation for optimum affect. Conventional strategies usually depend on manually curated options and task-specific fashions, making them inflexible and difficult to adapt to new or associated duties. Inhabitants dynamics fashions, in contrast, present a versatile framework for analyzing how environmental, social, and financial components affect public well being outcomes. The analysis underscores that native ecological components can higher predict long-term well being outcomes than genetics, highlighting the important function of geospatial modeling in tackling public well being challenges, together with illness administration and climate-related well being impacts.
Machine studying has considerably enhanced geospatial modeling by leveraging various knowledge sources to extend spatial and temporal decision. Research have utilized cell phone knowledge, net search developments, satellite tv for pc imagery, and climate info to foretell inhabitants motion, illness outbreaks, and financial developments. Regardless of providing actionable insights, these strategies usually rely upon labor-intensive, hand-crafted options and customized fashions, limiting scalability and interoperability. To deal with this, latest developments resembling GPS2Vec, SatCLIP, and GeoCLIP deal with creating versatile geographic encoders through the use of geotagged knowledge, satellite tv for pc imagery, and image-to-GPS alignment. Constructing on these improvements, newer fashions intention to combine human conduct alerts with environmental knowledge to provide general-purpose frameworks for improved geospatial inference.
Researchers from Google Analysis and the College of Nevada, Reno, launched the Inhabitants Dynamics Basis Mannequin (PDFM), a flexible framework for geospatial modeling. By establishing a geo-indexed dataset incorporating human conduct (e.g., aggregated search developments) and environmental alerts (e.g., climate, air high quality), PDFM makes use of graph neural networks to create embeddings for various duties. Benchmarked throughout 27 well being, socioeconomic, and environmental duties, PDFM achieves state-of-the-art geospatial interpolation, extrapolation, and super-resolution efficiency. It enhances forecasting fashions like TimesFM, surpassing supervised strategies with out fine-tuning. With publicly out there embeddings and code, PDFM provides scalable geospatial options for analysis, social good, well being, and enterprise purposes.
The examine curated 5 datasets on the postal code degree throughout the contiguous US (CONUS) for coaching and analysis, specializing in aggregated search developments, maps, busyness, climate, and satellite tv for pc imagery. Search developments concerned the highest 1,000 queries from July 2022, scaled and anonymized for privateness. Maps and busyness knowledge supplied insights into services and exercise ranges by class. Climate and air high quality metrics included local weather and pollutant knowledge for July 2022. Satellite tv for pc embeddings utilized SatCLIP’s Sentinel-2 imagery from 2021–2023. Whereas temporal alignment various, these datasets coated 28,000 postal codes, representing over 95% of the US inhabitants, with exclusions for sparsely populated areas.
To develop PDFM, 5 datasets protecting maps, busyness, search developments, climate, and air high quality had been collected at postal code and county ranges. Utilizing GNNs, PDFM was skilled to generate versatile embeddings for fixing 27 downstream well being, socioeconomic, and environmental duties. Interpolation and extrapolation experiments simulated lacking knowledge situations at postal code ranges, with PDFM outperforming benchmarks like SatCLIP and GeoCLIP throughout most duties. Ablation research revealed search developments and maps as key contributors. In super-resolution duties, PDFM confirmed superior efficiency, reaching excessive correlation in postal code-level predictions, highlighting its effectiveness in geospatial forecasting and downstream purposes.
In conclusion, The PDFM framework addresses various geospatial challenges throughout the U.S., outperforming current fashions like SatCLIP and GeoCLIP on varied duties and enhancing forecasting fashions resembling TimesFM. It integrates various datasets, demonstrating adaptability to new duties, restricted knowledge situations, and ranging resolutions. Future instructions embrace addressing temporal alignment points, incorporating dynamic embeddings, exploring extra datasets, and leveraging non-spatial graph edges. Limitations embrace reliance on aggregated knowledge and regional knowledge disparities. The PDFM’s privacy-preserving design ensures broad applicability, with potential international extensions requiring revolutionary options for low-data areas and reliability estimates to boost predictions in underrepresented areas.
Try the Paper and GitHub Repo. All credit score for this analysis goes to the researchers of this venture. Additionally, don’t neglect to comply with us on Twitter and be a part of our Telegram Channel and LinkedIn Group. When you like our work, you’ll love our publication.. Don’t Neglect to hitch our 60k+ ML SubReddit.
🚨 [Must Attend Webinar]: ‘Rework proofs-of-concept into production-ready AI purposes and brokers’ (Promoted)
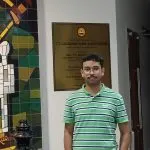
Sana Hassan, a consulting intern at Marktechpost and dual-degree pupil at IIT Madras, is obsessed with making use of know-how and AI to handle real-world challenges. With a eager curiosity in fixing sensible issues, he brings a recent perspective to the intersection of AI and real-life options.