Present datasets used to coach and consider AI-based mathematical assistants, notably LLMs, are restricted in scope and design. They typically concentrate on undergraduate-level arithmetic and depend on binary score protocols, making them unsuitable for evaluating complicated proof-based reasoning comprehensively. These datasets lack illustration of crucial points of mathematical workflows, similar to intermediate steps and problem-solving methods important in mathematical analysis. To beat these limitations, there’s a urgent want to revamp datasets to incorporate components like “motivated proofs,” which emphasize reasoning processes over outcomes, and workflows that seize the nuanced duties concerned in mathematical discovery.
Latest developments in AI for arithmetic, similar to AlphaGeometry and Numina, have efficiently solved Olympiad-level issues and transformed mathematical queries into executable code. Nevertheless, the proliferation of benchmarks, similar to GSM8K and MATH, has led to over-reliance on a couple of datasets whereas neglecting superior arithmetic and sensible workflows. Whereas extremely specialised fashions excel in slim domains requiring formal language enter, general-purpose fashions like LLMs intention to help mathematicians broadly by way of pure language interplay and gear integration. Regardless of their progress, these programs face challenges similar to dataset contamination and lack of alignment with real-world mathematical practices, highlighting the necessity for extra complete analysis strategies and coaching knowledge.
Researchers from establishments like Oxford, Cambridge, Caltech, and Meta emphasize bettering LLMs to function efficient “mathematical copilots.” Present datasets, similar to GSM8K and MATH, fall in need of capturing the nuanced workflows and motivations central to mathematical analysis. The authors advocate for a shift in direction of datasets reflecting sensible mathematical duties impressed by ideas like Pólya’s “motivated proof.” They suggest integrating symbolic instruments and specialised LLM modules to reinforce reasoning alongside growing common fashions for theorem discovery. The research underscores the significance of datasets tailor-made to mathematicians’ must information the event of extra succesful AI programs.
Whereas not particularly designed for arithmetic, present general-purpose LLMs have demonstrated robust capabilities in fixing complicated issues and producing mathematical textual content. GPT-4, for instance, performs properly on undergraduate-level math issues, and Google’s Math-Specialised Gemini 1.5 Professional has achieved over 90% accuracy on the MATH dataset. Regardless of these developments, considerations exist concerning the reproducibility of outcomes, as datasets could also be contaminated or not correctly examined, doubtlessly affecting generalization to various drawback sorts. Specialised fashions like MathPrompter and MathVista carry out properly in arithmetic and geometry however are restricted by the slim focus of accessible datasets, typically omitting superior reasoning duties.
The research highlights how present datasets fail to assist AI fashions in addressing the total spectrum of mathematical analysis, notably in duties like conjecture era and proof methods. Present datasets primarily concentrate on question-answering or theorem proving with out evaluating the intermediate reasoning course of or workflows mathematicians observe. Many formal datasets lack drawback complexity, undergo from device misalignment, or face knowledge duplication points. To beat these challenges, the paper advocates for growing new datasets encompassing a variety of mathematical analysis actions, similar to literature search and proof formulation, together with a complete taxonomy of workflows to information future mannequin growth.
In conclusion, The research discusses AI’s challenges in turning into a real mathematical companion, much like GitHub Copilot for programmers. It highlights the complementary nature of pure and formal language datasets, noting that what is straightforward in a single illustration could also be tough within the different. The authors emphasize the necessity for higher datasets that seize mathematical workflows, intermediate steps, and the flexibility to evaluate proof strategies. They argue for growing datasets past proofs and outcomes to incorporate reasoning, heuristics, and summarization, which can help AI in accelerating mathematical discovery and supporting different scientific disciplines.
Try the Paper. All credit score for this analysis goes to the researchers of this undertaking. Additionally, don’t overlook to observe us on Twitter and be a part of our Telegram Channel and LinkedIn Group. Don’t Neglect to affix our 60k+ ML SubReddit.
🚨 Trending: LG AI Analysis Releases EXAONE 3.5: Three Open-Supply Bilingual Frontier AI-level Fashions Delivering Unmatched Instruction Following and Lengthy Context Understanding for International Management in Generative AI Excellence….
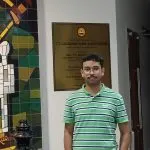
Sana Hassan, a consulting intern at Marktechpost and dual-degree pupil at IIT Madras, is keen about making use of expertise and AI to handle real-world challenges. With a eager curiosity in fixing sensible issues, he brings a recent perspective to the intersection of AI and real-life options.