Wenn wir über Risikofaktoren für die Alzheimer-Krankheit nachdenken, konzentrieren sich viele sofort auf Berufe mit hohen kognitiven Anforderungen. Oft werden Hochdruckberufe wie Ärzte oder Forscher mit einem geringeren Alzheimer-Risiko in Verbindung gebracht. Diese Berufe erfordern ständiges Denken und Problemlösen. Doch eine überraschende neue Studie der Harvard Medical School stellt diese Annahme in Frage. Die Studie zeigt, dass Taxi- und Krankenwagenfahrer die niedrigsten Alzheimer-Sterberaten unter über 400 untersuchten Berufen aufweisen.
Die Harvard-Studie: Neue Erkenntnisse zum kognitiven Abbau
Im Dezember 2024 veröffentlichten Forscher der Harvard Medical School im British Medical Journal eine Studie mit Daten von fast 9 Millionen Menschen aus 443 Berufen. Die überraschenden Ergebnisse: Taxi- und Krankenwagenfahrer wiesen mit 1,03% bzw. 0,91% die niedrigsten Alzheimer-Sterberaten auf. Diese Entdeckung stellt bisherige Annahmen über den Zusammenhang zwischen kognitiver Aktivität und Demenzrisiko in Frage.
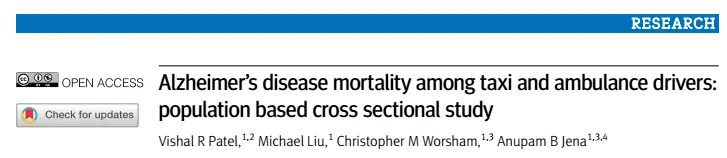
Forschungs-Screenshots
Die Rolle der räumlichen Navigation
Der Schlüssel zum Verständnis dieser Ergebnisse könnte in der räumlichen Orientierung liegen. Sowohl Taxi- als auch Rettungsfahrer müssen ständig komplexe Navigationsentscheidungen in Echtzeit treffen. Diese ständige Beanspruchung des Hippocampus – der für Gedächtnis und räumliche Orientierung zuständigen Hirnregion – könnte eine schützende Wirkung entfalten. Studien an Londoner Taxifahrern zeigen, dass sich deren Hippocampi tatsächlich strukturell verändern.
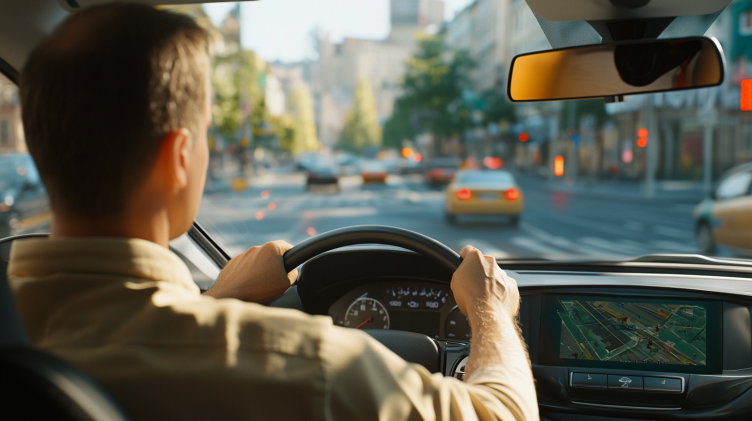
Vergleich verschiedener Fahrberufe
Interessanterweise zeigen andere Fahrberufe wie Busfahrer oder Piloten (4,57% Alzheimer-Sterblichkeit) dieses Phänomen nicht. Der entscheidende Unterschied liegt in der Notwendigkeit zu spontaner Navigation. Während Busfahrer festen Routen folgen und Piloten standardisierten Flugplänen folgen, erfordert die dynamische Routenplanung von Taxi- und Rettungsfahrern ständige Anpassungsfähigkeit.
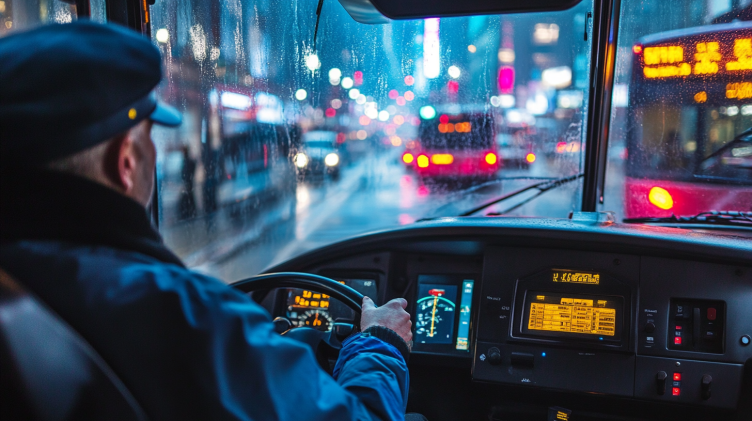
Kognitive Reserve und Alzheimer-Prävention
Diese Erkenntnisse stützen die “kognitive Reserve”-Theorie. Studien an Londoner Taxifahrern zeigen, dass deren Hippocampi durch die berufliche Beanspruchung strukturelle Veränderungen entwickeln. Diese Anpassungsfähigkeit des Gehirns könnte den kognitiven Abbau verlangsamen. Eine Studie des University College London zeigte, dass Taxifahrer mit mehr Berufsjahren eine höhere graue Substanz im Hippocampus aufweisen.
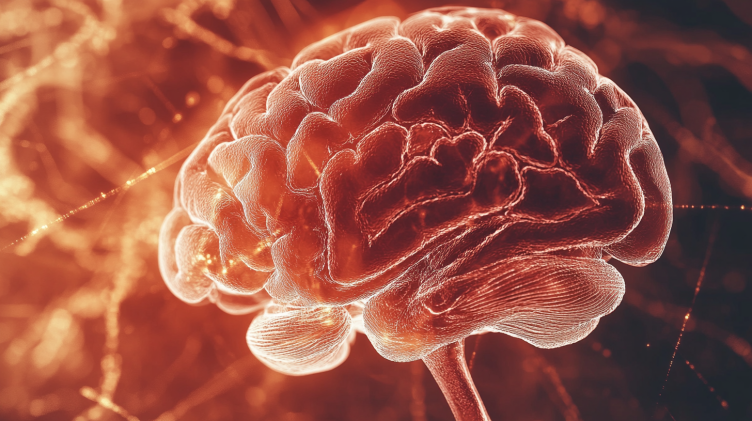
Alzheimer-Prävention ab 40
Die Alzheimer-Erkrankung beginnt schleichend. Erste Symptome wie Kurzzeitgedächtnisstörungen können bereits Jahrzehnte vor der Diagnose auftreten. Bildgebende Verfahren zeigen, dass erste Veränderungen im medialen Temporallappen bereits 10-15 Jahre vor der Diagnose nachweisbar sind. Diese Erkenntnis unterstreicht die Bedeutung frühzeitiger Prävention.
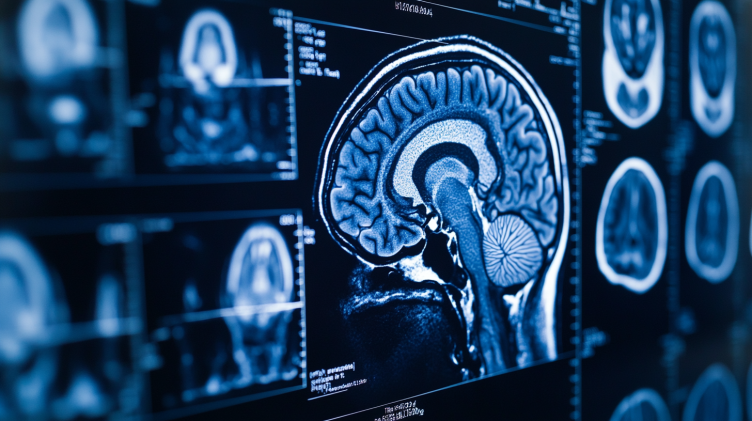
7 Wege zur Alzheimer-Prävention
Die 2024 veröffentlichten chinesischen Präventionsleitlinien betonen sieben Schlüsselstrategien:
- Soziale Aktivität und psychische Gesundheit
Regelmßiger sozialer Austausch reduziert das Demenzrisiko um bis zu 60%. Eine Studie der Johns Hopkins University zeigt, dass einsame Menschen ein 2,8-fach erhöhtes Alzheimer-Risiko haben.
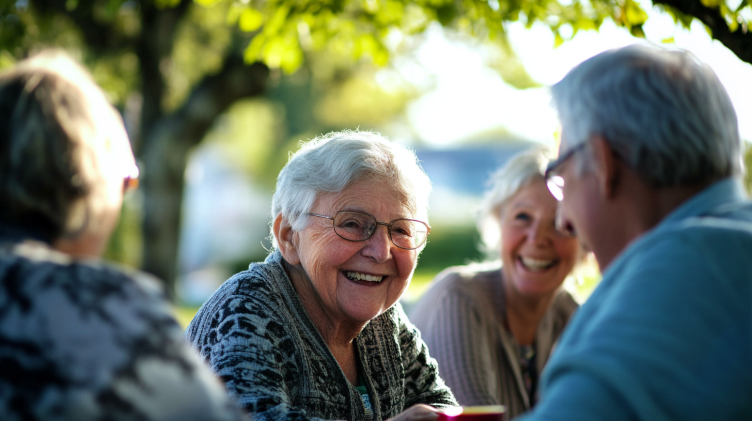
- Lebenslanges Lernen
Eine Studie der Universität Cambridge zeigt, dass das Erlernen neuer Sprachen das Demenzrisiko um 40% senken kann. Das Erlernen eines Musikinstruments kann die kognitive Reserve um bis zu 35% erhöhen. - Regelmßige Bewegung
Eine Studie des Karolinska-Instituts zeigt, dass bereits 150 Minuten moderate Bewegung pro Woche das Alzheimer-Risiko um 45% reduziert. Besonders effektiv ist Ausdauersport wie Schwimmen oder Radfahren.
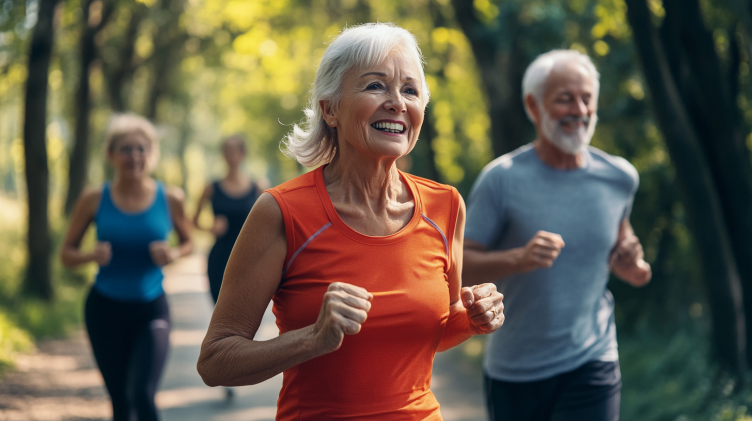
- Verzicht auf Rauchen und Alkohol
Eine Studie der Universität Oxford zeigt, dass Raucher ein 79% höheres Alzheimer-Risiko haben. Mäßiger Alkoholkonsum (max. 1 Glas/Tag für Frauen) kann jedoch das Risiko um 23% senken. - Schlafhygiene
Eine Studie der National Institutes of Health zeigt, dass bereits eine Nacht mit weniger als 6 Stunden Schlaf die Beta-Amyloid-Ablagerungen um 25% erhöht. Die optimale Schlafdauer liegt bei 7-8 Stunden. - Mittelmeer-Diät
Eine Studie im “Journal of Alzheimer’s Disease” zeigt, dass die Mittelmeer-Diät das Alzheimer-Risiko um 53% senken kann. Besonders Olivenöl und Nüsse zeigen starke Schutzeffekte.
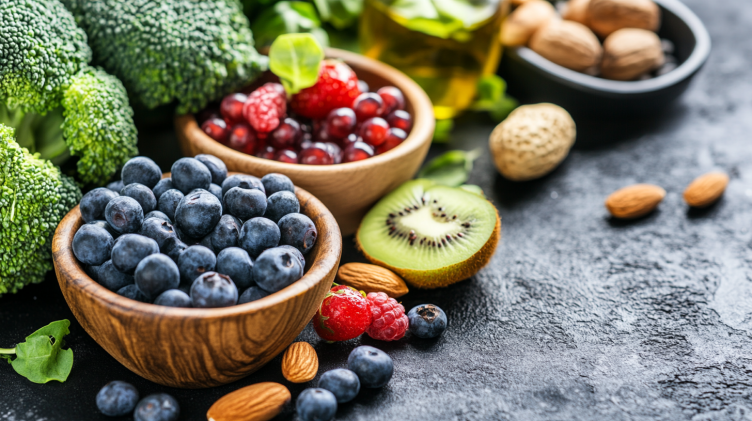
- Gewichtskontrolle und Blutdruckmanagement
Eine Studie der Universität Cambridge zeigt, dass ein BMI über 30 das Alzheimer-Risiko um 74% erhöht. Jeder verlorene BMI-Punkt reduziert das Risiko um 13%.
Fazit
Die Verbindung zwischen Navigationsfähigkeit und Alzheimer-Risiko unterstreicht die Bedeutung der kognitiven Flexibilität. Während kognitive Stimulation wichtig ist, zeigt die Studie, dass spezifische Formen der mentalen Beanspruchung – wie die dynamische Raumorientierung – besonders schützend wirken. Diese Erkenntnisse eröffnen neue Wege in der Alzheimer-Prävention.