Current developments in SSL have led to the event of basis fashions (FMs) that analyze intensive biomedical knowledge, enhancing well being outcomes. Steady Glucose Monitoring (CGM) provides wealthy, temporal glucose knowledge however should be utilized for broader well being predictions. SSL allows FMs to research unlabelled knowledge effectively, bettering detection charges in varied medical fields, from retinal photos to sleep issues and pathology. With diabetes affecting over 500 million folks globally and growing healthcare prices, CGM has confirmed superior to conventional glucose monitoring by bettering glycemic management and high quality of life. The FDA’s approval of an over-the-counter CGM system highlights its rising accessibility and potential advantages for diabetic and non-diabetic people.
Researchers from establishments together with Weizmann Institute of Science and NVIDIA introduce GluFormer, a generative basis mannequin utilizing a transformer structure, educated on over 10 million CGM measurements from 10,812 non-diabetic people. GluFormer, educated with a self-supervised strategy, generalizes effectively throughout 15 exterior datasets and varied populations, exhibiting superior efficiency in predicting medical parameters corresponding to HbA1c, liver metrics, and future well being outcomes, together with as much as 4 years forward. The mannequin additionally integrates dietary knowledge to simulate dietary interventions and personalize meals responses. GluFormer represents a big development in managing continual illnesses and bettering precision well being methods.
GluFormer is a transformer-based mannequin designed to research CGM knowledge from 10,812 individuals; every tracked for 2 weeks. The mannequin processes glucose readings, that are recorded each quarter-hour and initially cleansed of calibration noise. Information was tokenized into 460 glucose worth intervals and segmented into 1200-measurement samples, with a particular token used for shorter samples. The mannequin structure contains 16 transformer layers, 16 consideration heads, and an embedding dimension of 1024, dealing with sequences of as much as 25,000 tokens. Pretraining concerned predicting subsequent tokens utilizing causal masking and cross-entropy loss. Optimization was carried out with AdamW and a studying charge scheduler, and the ultimate analysis was based mostly on efficiency metrics from a validation set.
GluFormer outputs have been pooled for medical utility utilizing strategies corresponding to Max Pooling, which proved best for predicting medical outcomes like HbA1c. Medical metrics derived from CGM knowledge utilizing the R bundle iglu have been used with ridge regression fashions to foretell varied outcomes. The mannequin’s generalizability was assessed on exterior datasets, and its means to foretell outcomes of randomized medical trials was evaluated. Comparisons have been made with different fashions, together with a plain transformer and CNNs. On the identical time, strategies for incorporating temporal data and dietary knowledge have been additionally explored, demonstrating that realized temporal embeddings and food regimen tokens improved predictive efficiency.
The GluFormer mannequin demonstrated sturdy efficiency in producing CGM knowledge and predicting medical outcomes. Skilled on a big dataset, GluFormer excels in producing correct CGM indicators and embedding them for varied functions. It confirmed sturdy efficiency in predicting medical parameters like HbA1c, visceral adipose tissue, and fasting glucose. The mannequin successfully generalizes throughout cohorts and integrates dietary knowledge, enhancing glucose response predictions. Enhancements, together with temporal encoding and multimodal integration, refine its predictive accuracy. These outcomes underscore GluFormer’s utility in personalised healthcare and its adaptability to numerous datasets and timeframes.
In conclusion, GluFormer, educated on CGM knowledge from over 10,000 non-diabetic people, excels in producing correct glucose indicators and predicting a spread of medical outcomes. It outperforms conventional metrics in forecasting parameters like HbA1c and liver perform and exhibits broad applicability throughout totally different populations and well being circumstances. The mannequin’s latent house successfully captures nuanced facets of glucose metabolism, enhancing its means to foretell diabetes development and medical trial outcomes. Integration of dietary knowledge additional refines its predictions. Regardless of its potential, challenges stay, together with dataset limitations, dietary knowledge accuracy, and mannequin interpretability. GluFormer represents a big development in personalised metabolic well being administration and analysis.
Try the Paper and Challenge. All credit score for this analysis goes to the researchers of this venture. Additionally, don’t overlook to observe us on Twitter and be part of our Telegram Channel and LinkedIn Group. For those who like our work, you’ll love our e-newsletter..
Don’t Overlook to hitch our 50k+ ML SubReddit
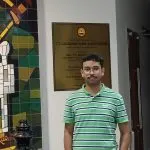
Sana Hassan, a consulting intern at Marktechpost and dual-degree pupil at IIT Madras, is obsessed with making use of know-how and AI to deal with real-world challenges. With a eager curiosity in fixing sensible issues, he brings a contemporary perspective to the intersection of AI and real-life options.