Microscopic imaging is essential in trendy drugs as an indispensable software for researchers and clinicians. This imaging expertise permits detailed examination of organic buildings on the mobile and molecular ranges, enabling the research of tissue samples in illness prognosis and pathology. By capturing these microscopic photographs, medical professionals can higher perceive illness mechanisms and development, usually revealing delicate modifications not detectable by way of different strategies. Nonetheless, regardless of the significance of those photographs, their classification and interpretation often demand specialised experience and substantial time funding, resulting in inefficiencies in prognosis. As the quantity of medical knowledge grows, the demand for automated, environment friendly, and correct instruments for microscopic picture classification has change into extra urgent.
A key situation in medical picture classification is the problem of successfully decoding and classifying these complicated photographs. Guide classification is gradual and vulnerable to inconsistencies as a result of subjective nature of human judgment. Furthermore, the dimensions of the info generated by way of microscopic imaging makes handbook evaluation impractical in lots of situations. Conventional machine studying strategies, equivalent to convolutional neural networks (CNNs), have been employed for this activity, however they arrive with limitations. Whereas CNNs are highly effective in extracting native options, their means to seize long-range dependencies throughout the picture is restricted. This restriction prevents them from absolutely using the semantic info embedded in medical photographs, which is essential for correct classification and prognosis. However, imaginative and prescient transformers (ViTs), identified for his or her effectivity in modeling international dependencies, undergo from excessive computational complexity, notably in long-sequence modeling, which renders them much less appropriate for real-time medical functions the place computational sources could also be restricted.
Present strategies to handle these limitations have included hybrid approaches combining CNNs and transformers. These strategies try to stability between native and international characteristic extraction however usually come at the price of both accuracy or computational effectivity. Some research have proposed reduced-complexity ViTs to make them extra possible for sensible use. Nonetheless, these fashions usually sacrifice precision in medical imaging, the place each pixel’s info may very well be essential for correct prognosis. Thus, there’s a clear want for extra environment friendly fashions that may successfully deal with each native and international info with out a vital computational burden.
A analysis staff from Nanjing Agricultural College, Nationwide College of Protection Expertise, Xiangtan College, Nanjing College of Posts and Telecommunications, and Soochow College launched a novel structure referred to as Microscopic-Mamba to handle these challenges. This hybrid mannequin was particularly designed to enhance microscopic picture classification by combining the strengths of CNNs in native characteristic extraction with the effectivity of State House Fashions (SSMs) in capturing long-range dependencies. The staff’s mannequin integrates the Partially Chosen Feed-Ahead Community (PSFFN) to interchange the ultimate linear layer within the Imaginative and prescient State House Module (VSSM), considerably enhancing the power to understand native options whereas sustaining a compact and environment friendly structure. By incorporating international and native info processing capabilities, the Microscopic-Mamba mannequin seeks to set a brand new benchmark in medical picture classification.
The core methodology behind Microscopic-Mamba lies in its dual-branch construction, consisting of a convolutional department for native characteristic extraction and an SSM department for international characteristic modeling. The mannequin additionally introduces the Modulation Interplay Characteristic Aggregation (MIFA) module, designed to successfully fuse international and native options. On this structure, the CNN department makes use of depth-wise separable convolution (DWConv) and point-wise convolution (PWConv) for localized characteristic extraction. In distinction, the SSM department focuses on international characteristic modeling by way of the parallel Imaginative and prescient State House Module (VSSM). Integrating these two modules permits Microscopic-Mamba to course of detailed native info and broad international patterns, which is important for correct medical picture evaluation. The ultimate layer within the VSSM is changed with the PSFFN, which refines the mannequin’s means to seize native info, optimizing the stability between element and generalization.
The Microscopic-Mamba mannequin demonstrated superior efficiency on 5 public medical picture datasets in in depth testing. These datasets included the Retinal Pigment Epithelium (RPE) Cell dataset, the SARS dataset for malaria cell classification, the MHIST dataset for colorectal polyp classification, the MedFM Colon dataset for tumor tissue classification, and the TissueMNIST dataset, which comprises over 236,386 photographs of human kidney cells. The mannequin achieved a outstanding stability of excessive accuracy and low computational calls for, making it superb for real-world medical functions. On the RPE dataset, for instance, Microscopic-Mamba achieved an general accuracy (OA) of 87.60% and an space below the curve (AUC) of 98.28%, outperforming present strategies. The mannequin’s light-weight design, with solely 4.49 GMACs and 1.10 million parameters on some duties, ensures that it may be deployed in environments with restricted computational sources whereas sustaining excessive accuracy.
Ablation research confirmed that introducing the MIFA module and the PSFFN was essential to the mannequin’s success. Combining these two components led to notable enhancements in efficiency throughout all datasets. On the MHIST dataset, the mannequin achieved an AUC of 99.56% with solely 4.86 million parameters, underscoring its effectivity and effectiveness in medical picture classification.

In conclusion, the Microscopic-Mamba mannequin considerably advances medical picture classification. By combining the strengths of CNNs and SSMs, this hybrid structure efficiently addresses the restrictions of earlier strategies, providing an answer that’s each computationally environment friendly and extremely correct. The mannequin’s means to course of and combine native and international options makes it well-suited for microscopic picture evaluation. With its spectacular efficiency throughout a number of datasets, Microscopic-Mamba has the potential to change into a typical software in automated medical diagnostics, streamlining the method and enhancing the accuracy of illness identification.
Try the Paper and GitHub Web page. All credit score for this analysis goes to the researchers of this venture. Additionally, don’t overlook to comply with us on Twitter and be part of our Telegram Channel and LinkedIn Group. In case you like our work, you’ll love our e-newsletter..
Don’t Overlook to affix our 50k+ ML SubReddit
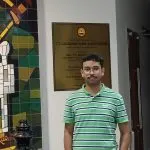
Sana Hassan, a consulting intern at Marktechpost and dual-degree pupil at IIT Madras, is obsessed with making use of expertise and AI to handle real-world challenges. With a eager curiosity in fixing sensible issues, he brings a recent perspective to the intersection of AI and real-life options.