Machine Studying in Membrane Science:
ML considerably transforms pure sciences, significantly cheminformatics and supplies science, together with membrane know-how. This evaluation focuses on present ML purposes in membrane science, providing insights from each ML and membrane views. It begins by explaining foundational ML algorithms and design rules, then an in depth examination of conventional and deep studying approaches within the membrane area. The evaluation highlights the function of information and featurization in molecular and membrane programs and explores how ML has been utilized in areas like reverse osmosis, fuel separation, and nanofiltration. The excellence between predictive duties and generative membrane design can also be mentioned, together with really useful finest practices for guaranteeing reproducibility in ML research on membranes. That is the primary evaluation that systematically covers the intersection of ML and membrane science.
Introducing data-driven approaches, similar to ML, has led to important developments in numerous scientific disciplines. Challenges in membrane science typically contain complicated, multidimensional issues that ML can successfully handle. Membrane processes similar to fuel separation and filtration profit from the flexibility of ML algorithms to research huge datasets, predict materials properties, and help in membrane design. Furthermore, current research emphasize the rising curiosity in ML purposes inside this subject, as evidenced by the rising variety of publications on the subject. The evaluation additionally explores superior methods like graph neural networks (GNNs) and generative membrane design, that are promising for future developments in nonlinear materials innovation.
Machine Studying Approaches in Membrane Science:
Conventional scientific analysis typically follows a hypothesis-driven framework, the place new theories come up from established observations and are validated by way of experiments. This mannequin formulation course of entails refining a bodily mannequin based mostly on empirical proof. Nevertheless, the emergence of information science has shifted this paradigm, enabling researchers to make use of ML methods that may mannequin bodily phenomena with no predefined theoretical foundation. By leveraging huge quantities of information, ML fashions can adapt and acknowledge patterns with out important a priori conceptualization, relying closely on the standard and quantity of coaching information. The efficiency of those fashions is crucially assessed by way of validation and testing phases to keep away from underfitting and overfitting—situations that impede the mannequin’s predictive accuracy.
Efficient featurization is significant for profitable ML implementation within the context of membrane purposes. Membrane separation processes include a matrix, membrane, and numerous course of parameters, which should be precisely represented. Completely different featurization methods—similar to fingerprints and graph-based representations—rework molecular constructions into codecs that ML algorithms can course of. This strategy permits for higher prediction of properties based mostly on the underlying chemical relationships and traits. By using area information to pick related parameters, researchers can optimize their fashions and enhance the accuracy of predictions, addressing challenges like information sparsity and overfitting whereas facilitating developments in membrane science.
Developments in Membrane Expertise by way of Machine Studying Improvements:
Latest research have targeted on enhancing membrane efficiency by way of ML methods, addressing excessive prices and labor-intensive materials growth challenges. Conventional approaches, typically reliant on trial and error, need assistance with the multi-dimensional complexities of membrane design. By using computational fashions, researchers have analyzed efficiency metrics similar to permeability and selectivity, optimizing present processes and informing the event of recent supplies. Predictive fashions are instrumental in figuring out structure-property relationships throughout numerous membrane sorts and purposes, together with ultrafiltration and electrolytic conductivity, enhancing general efficiency and effectivity in membrane know-how.
Fouling is a major subject in membrane purposes, which negatively impacts efficiency and will increase operational prices. Knowledge-driven strategies have emerged to observe and predict fouling, resulting in value financial savings by optimizing cleansing schedules and lowering pointless membrane replacements. Numerous ML methods, together with synthetic neural networks (ANNs) and genetic algorithms, have been utilized to deal with fouling by analyzing enter parameters similar to biomass traits and working situations. Moreover, ML is being more and more built-in into wastewater remedy and fuel separation processes, optimizing operational parameters and enhancing the design of membranes, significantly in complicated purposes like natural solvent nanofiltration. These developments spotlight the potential of hybrid ML approaches in bettering membrane know-how on an industrial scale. Nevertheless, there stays a necessity for broader analysis encompassing various membrane supplies and real-time monitoring capabilities.
Tips for Machine Studying in Membrane Science:
Adopting finest practices in ML is essential to enhancing reproducibility in membrane-related purposes. This consists of guaranteeing dependable information sources, cleansing datasets, and deciding on acceptable algorithms. Mannequin coaching ought to contain correct validation and hyperparameter tuning. Analysis metrics should be well-defined, with methods to stop overfitting and guarantee mannequin explainability. Moral issues ought to information the usage of ML in analysis. Complete documentation and clear reporting of methodologies and outcomes are important for fostering belief inside the membrane analysis neighborhood and facilitating efficient information sharing.
Try the Paper. All credit score for this analysis goes to the researchers of this mission. Additionally, don’t overlook to observe us on Twitter and be a part of our Telegram Channel and LinkedIn Group. For those who like our work, you’ll love our e-newsletter..
Don’t Neglect to affix our 50k+ ML SubReddit
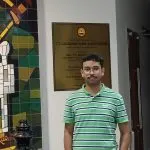
Sana Hassan, a consulting intern at Marktechpost and dual-degree pupil at IIT Madras, is captivated with making use of know-how and AI to handle real-world challenges. With a eager curiosity in fixing sensible issues, he brings a recent perspective to the intersection of AI and real-life options.