Synthetic intelligence has considerably enhanced complicated reasoning duties, notably in specialised domains akin to arithmetic. Giant Language Fashions (LLMs) have gained consideration for his or her capability to course of giant datasets and clear up intricate issues. The mathematical reasoning capabilities of those fashions have vastly improved over time. This progress has been pushed by developments in coaching strategies, akin to Chain-of-Thought (CoT) prompting, and various datasets, permitting these fashions to resolve numerous mathematical issues, from easy arithmetic to complicated high-school competition-level duties. The rising sophistication of LLMs has made them indispensable instruments in fields the place superior reasoning is required. Nonetheless, the standard and scale of obtainable pre-training datasets have restricted their full potential, particularly for open-source initiatives.
A key concern that hinders the event of mathematical reasoning in LLMs is the dearth of complete multimodal datasets that combine textual content and visible knowledge, akin to diagrams, equations, and geometric figures. Most mathematical information is expressed by means of textual explanations and visible components. Whereas proprietary fashions like GPT-4 and Claude 3.5 Sonnet have leveraged intensive personal datasets for pre-training, the open-source neighborhood has struggled to maintain up as a result of shortage of high-quality, publicly out there datasets. With out these sources, it’s troublesome for open-source fashions to advance in dealing with the complicated reasoning duties that proprietary fashions deal with. This hole in multimodal datasets has made it difficult for researchers to coach fashions that may deal with text-based and visible reasoning duties.
A number of approaches have been used to coach LLMs for mathematical reasoning, however most deal with text-only datasets. For example, proprietary datasets like WebMath and MathMix have offered billions of textual content tokens for coaching fashions like GPT-4, however they don’t deal with the visible components of arithmetic. Open-source datasets like OpenWebMath and DeepSeekMath have additionally been launched, however they’re primarily targeted on mathematical textual content quite than integrating visible and textual knowledge. Whereas these datasets have superior LLMs in particular areas of math, akin to arithmetic and algebra, they fall brief in the case of complicated, multimodal reasoning duties that require integrating visible components with textual content. This limitation has led to creating fashions that carry out properly on text-based duties however battle with multimodal issues that mix written explanations with diagrams or equations.
Researchers from ByteDance and the Chinese language Academy of Sciences launched InfiMM-WebMath-40B, a complete dataset that gives a large-scale multimodal useful resource particularly designed for mathematical reasoning. This dataset contains 24 million net pages, 85 million related picture URLs, and roughly 40 billion textual content tokens extracted and filtered from the CommonCrawl repository. The analysis crew meticulously filtered the information to make sure the inclusion of high-quality, related content material, making it the primary of its variety within the open-source neighborhood. By combining textual and visible mathematical knowledge, InfiMM-WebMath-40B affords an unprecedented useful resource for coaching Multimodal Giant Language Fashions (MLLMs), enabling them to course of and purpose with extra complicated mathematical ideas than ever.
The dataset was constructed utilizing a rigorous knowledge processing pipeline. Researchers started with 122 billion net pages, filtered to 24 million net paperwork, guaranteeing the content material targeted on arithmetic and science. FastText, a language identification instrument, filtered out non-English and non-Chinese language content material. The dataset’s multimodal nature required particular consideration to picture extraction and the alignment of pictures with their corresponding textual content. In whole, 85 million picture URLs have been extracted, filtered, and paired with related mathematical content material, making a dataset that integrates visible and textual components to reinforce the mathematical reasoning capabilities of LLMs.
The efficiency of fashions skilled on InfiMM-WebMath-40B has considerably improved in comparison with earlier open-source datasets. In evaluations performed on benchmarks akin to MathVerse and We-Math, fashions skilled utilizing this dataset outperformed others of their capability to course of each textual content and visible data. For example, regardless of using solely 40 billion tokens, the researchers’ mannequin, InfiMM-Math, carried out comparably to proprietary fashions that used 120 billion tokens. On the MathVerse benchmark, InfiMM-Math demonstrated superior efficiency in text-dominant, text-lite, and vision-intensive classes, outperforming many open-source fashions with a lot bigger datasets. Equally, on the We-Math benchmark, the mannequin achieved exceptional outcomes, demonstrating its functionality to deal with multimodal duties and setting a brand new customary for open-source LLMs.

In conclusion, InfiMM-WebMath-40B, providing a large-scale, multimodal dataset, should deal with extra knowledge for coaching open-source fashions to deal with complicated reasoning duties involving textual content and visible knowledge. The dataset’s meticulous development and mixture of 40 billion textual content tokens with 85 million picture URLs present a strong basis for the subsequent technology of Multimodal Giant Language Fashions. The efficiency of fashions skilled on InfiMM-WebMath-40B highlights the significance of integrating visible components with textual knowledge to enhance mathematical reasoning capabilities. This dataset bridges the hole between proprietary and open-source fashions and paves the best way for future analysis to reinforce AI’s capability to resolve complicated mathematical issues.
Try the Paper and Dataset. All credit score for this analysis goes to the researchers of this venture. Additionally, don’t neglect to observe us on Twitter and be a part of our Telegram Channel and LinkedIn Group. Should you like our work, you’ll love our e-newsletter..
Don’t Neglect to hitch our 50k+ ML SubReddit
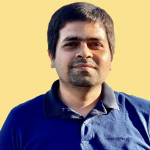
Asif Razzaq is the CEO of Marktechpost Media Inc.. As a visionary entrepreneur and engineer, Asif is dedicated to harnessing the potential of Synthetic Intelligence for social good. His most up-to-date endeavor is the launch of an Synthetic Intelligence Media Platform, Marktechpost, which stands out for its in-depth protection of machine studying and deep studying information that’s each technically sound and simply comprehensible by a large viewers. The platform boasts of over 2 million month-to-month views, illustrating its recognition amongst audiences.