Pure language processing (NLP) has skilled fast developments, with giant language fashions (LLMs) getting used to deal with numerous difficult issues. Among the many numerous purposes of LLMs, mathematical problem-solving has emerged as a benchmark to evaluate their reasoning skills. These fashions have demonstrated exceptional efficiency on math-specific benchmarks resembling GSM8K, which measures their capabilities to resolve grade-school math issues. Nevertheless, there’s an ongoing debate concerning whether or not these fashions really comprehend mathematical ideas or exploit patterns inside coaching knowledge to supply right solutions. This has led to a necessity for a deeper analysis to know the extent of their reasoning capabilities in dealing with advanced, interconnected drawback sorts.
Regardless of their success on current math benchmarks, researchers recognized a vital drawback: most LLMs have to exhibit constant reasoning when confronted with extra advanced, compositional questions. Whereas commonplace benchmarks contain fixing particular person issues independently, real-world situations usually require understanding relationships between a number of issues, the place the reply to at least one query have to be used to resolve one other. Conventional evaluations don’t adequately symbolize such situations, which focus solely on remoted problem-solving. This creates a discrepancy between the excessive benchmark scores and LLMs’ sensible usability for advanced duties requiring step-by-step reasoning and deeper understanding.
Researchers from Mila, Google DeepMind, and Microsoft Analysis have launched a brand new analysis technique known as “Compositional Grade-Faculty Math (GSM).” This technique includes chaining two separate math issues such that the answer to the primary drawback turns into a variable within the second drawback. Utilizing this method, researchers can analyze the LLMs’ skills to deal with dependencies between questions, an idea that must be adequately captured by current benchmarks. The Compositional GSM technique affords a extra complete evaluation of LLMs’ reasoning capabilities by introducing linked issues that require the mannequin to hold info from one drawback to a different, making it vital to resolve each accurately for a profitable consequence.
The analysis was carried out utilizing a wide range of LLMs, together with open-weight fashions like LLAMA3 and closed-weight fashions like GPT and Gemini households. The examine included three check units: the unique GSM8K check break up, a modified model of GSM8K the place some variables had been substituted, and the brand new Compositional GSM check set, every containing 1,200 examples. Fashions had been examined utilizing an 8-shot prompting technique, the place they got a number of examples earlier than being requested to resolve the compositional issues. This technique enabled the researchers to benchmark the fashions’ efficiency comprehensively, contemplating their capacity to resolve issues individually and in a compositional context.
The outcomes confirmed a substantial hole in reasoning skills. As an illustration, cost-efficient fashions resembling GPT-4o mini exhibited a 2 to 12 instances worse reasoning hole on compositional GSM in comparison with their efficiency on the usual GSM8K. Additional, math-specialized fashions like Qwen2.5-MATH-72B, which achieved above 80% accuracy on high-school competition-level questions, may solely clear up lower than 60% of the compositional grade-school math issues. This substantial drop means that greater than specialised coaching in arithmetic is required to organize fashions for multi-step reasoning duties adequately. Moreover, it was noticed that fashions like LLAMA3-8B and Mistral-7B, regardless of attaining excessive scores on remoted issues, confirmed a pointy decline when required to hyperlink solutions between associated issues.
The researchers additionally explored the impression of instruction tuning and code era on mannequin efficiency. Instruction-tuning improved outcomes for smaller fashions on commonplace GSM8K issues however led to solely minor enhancements on compositional GSM. In the meantime, producing code options as a substitute of utilizing pure language resulted in a 71% to 149% enchancment for some smaller fashions on compositional GSM. This discovering signifies that whereas code era helps scale back the reasoning hole, it doesn’t remove it, and systematic variations in reasoning capabilities persist amongst numerous fashions.
Evaluation of the reasoning gaps revealed that the efficiency drop was not on account of test-set leakage however somewhat to distractions brought on by extra context and poor second-hop reasoning. For instance, when fashions like LLAMA3-70B-IT and Gemini 1.5 Professional had been required to resolve a second query utilizing the reply of the primary, they incessantly wanted to use the answer precisely, leading to incorrect last solutions. This phenomenon, known as the second-hop reasoning hole, was extra pronounced in smaller fashions, which tended to miss essential particulars when fixing advanced issues.
The examine highlights that present LLMs, no matter their efficiency on commonplace benchmarks, nonetheless wrestle with compositional reasoning duties. The Compositional GSM benchmark launched within the analysis gives a useful device for evaluating the reasoning skills of LLMs past remoted problem-solving. These outcomes counsel that extra sturdy coaching methods and benchmark designs are wanted to boost the compositional capabilities of those fashions, enabling them to carry out higher in advanced problem-solving situations. This analysis underscores the significance of reassessing current analysis strategies and prioritizing the event of fashions able to multi-step reasoning.
Try the Paper. All credit score for this analysis goes to the researchers of this venture. Additionally, don’t neglect to comply with us on Twitter and be a part of our Telegram Channel and LinkedIn Group. Should you like our work, you’ll love our e-newsletter.. Don’t Neglect to hitch our 50k+ ML SubReddit
Occupied with selling your organization, product, service, or occasion to over 1 Million AI builders and researchers? Let’s collaborate!
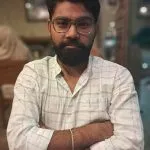
Nikhil is an intern marketing consultant at Marktechpost. He’s pursuing an built-in twin diploma in Supplies on the Indian Institute of Expertise, Kharagpur. Nikhil is an AI/ML fanatic who’s at all times researching purposes in fields like biomaterials and biomedical science. With a powerful background in Materials Science, he’s exploring new developments and creating alternatives to contribute.