In right this moment’s quickly evolving panorama, enterprise chatbots have gotten important instruments to boost worker productiveness by offering fast entry to organizational data. Nevertheless, the journey to construct efficient, scalable, and safe Retrieval-Augmented Technology (RAG) techniques is fraught with challenges. NVIDIA’s latest analysis gives a complete answer with the FACTS framework, addressing points comparable to content material freshness, structure, price effectivity, testing, and safety.
Challenges in Growing Enterprise Chatbots
Growing conversational AI techniques for enterprises presents distinctive challenges. Current chatbots typically wrestle to supply correct and contextually related responses, significantly when coping with dynamic and proprietary enterprise data. Retrieval-Augmented Technology (RAG) techniques mix the generative energy of Giant Language Fashions (LLMs), comparable to GPT-4, with retrieval mechanisms that guarantee responses are each informative and up-to-date. Nevertheless, implementing these techniques requires cautious engineering and optimization throughout a number of dimensions.
The FACTS Framework
To sort out the complexities of constructing profitable enterprise-grade chatbots, NVIDIA introduces the FACTS framework, specializing in Freshness, Structure, Price, Testing, and Safety. This complete method goals to information builders by designing, implementing, and optimizing RAG pipelines for enterprise environments.
- Freshness: Making certain that chatbot responses mirror probably the most present enterprise knowledge is important. By integrating vector databases that assist real-time content material retrieval, chatbots can keep up-to-date data for customers, addressing a typical weak point in static fashions.
- Structure: Constructing versatile and modular chatbot platforms is important to accommodate various enterprise wants. The framework helps the combination of a number of LLMs, vector databases, and different parts, permitting for personalisation and scalability.
- Price: Deploying generative AI options might be expensive, significantly when utilizing giant fashions. The FACTS framework emphasizes balancing the usage of each giant and small LLMs to optimize financial feasibility with out compromising on efficiency.
- Testing: Rigorous testing, together with each automated evaluations and human-in-the-loop validation, is important to make sure the accuracy, reliability, and security of chatbot responses.
- Safety: Defending delicate enterprise knowledge is a prime precedence. The framework addresses compliance with entry management insurance policies and implements guardrails to forestall unauthorized knowledge publicity.
Case Research: Chatbots at NVIDIA
NVIDIA’s analysis consists of case research of three enterprise chatbots developed utilizing the FACTS framework: NVInfo Bot, NVHelp Bot, and Scout Bot. Every chatbot serves a definite area, comparable to enterprise content material, IT assist, HR advantages, or monetary earnings, demonstrating the flexibility of the framework.
- NVInfo Bot manages roughly 500 million paperwork, guaranteeing that enterprise content material is accessible whereas implementing doc entry controls.
- NVHelp Bot focuses on IT assist and HR advantages, utilizing multi-modal knowledge sources to reply worker queries successfully.
- Scout Bot offers insights into firm financials by managing structured and unstructured knowledge from public sources.
The outcomes present that adhering to the FACTS rules considerably improves the reliability and consumer expertise of RAG-based chatbots. For instance, integrating a hybrid search mechanism—combining vector and lexical search—enhanced retrieval relevance, whereas using multi-agent architectures enabled the dealing with of extra complicated queries.
Conclusion
NVIDIA’s FACTS framework offers a holistic method to constructing efficient, safe, and enterprise-grade chatbots. By specializing in freshness, structure, price, testing, and safety, this analysis presents sensible options to the inherent challenges of deploying conversational AI in enterprise settings. The framework finally enhances chatbot efficiency, reliability, and consumer satisfaction, making it a precious useful resource for organizations seeking to leverage RAG-based techniques for his or her inner operations.
Take a look at the Paper. All credit score for this analysis goes to the researchers of this mission. Additionally, don’t overlook to comply with us on Twitter and be part of our Telegram Channel and LinkedIn Group. In case you like our work, you’ll love our e-newsletter.. Don’t Overlook to hitch our 50k+ ML SubReddit
Excited about selling your organization, product, service, or occasion to over 1 Million AI builders and researchers? Let’s collaborate!
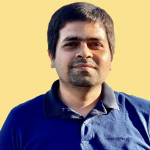
Asif Razzaq is the CEO of Marktechpost Media Inc.. As a visionary entrepreneur and engineer, Asif is dedicated to harnessing the potential of Synthetic Intelligence for social good. His most up-to-date endeavor is the launch of an Synthetic Intelligence Media Platform, Marktechpost, which stands out for its in-depth protection of machine studying and deep studying information that’s each technically sound and simply comprehensible by a large viewers. The platform boasts of over 2 million month-to-month views, illustrating its reputation amongst audiences.