Internet navigation brokers revolve round creating autonomous methods able to performing duties like looking out, procuring, and retrieving info from the web. These brokers make the most of superior language fashions to interpret directions and navigate by means of digital environments, making choices to execute duties that sometimes require human intervention. Regardless of vital developments on this space, brokers nonetheless wrestle with advanced, long-horizon duties that contain a sequence of interdependent actions. These duties demand a degree of adaptability and studying that present methods have but to have the ability to obtain successfully.
One main problem in growing these brokers is their incapability to study from earlier duties. Whereas they might carry out effectively with examples they’ve been particularly educated on, they’re typically inefficient when going through unfamiliar duties. Brokers function in isolation, fixing every job individually with out reusing previous experiences to tell future choices. This limitation reduces their effectivity and adaptableness, notably in environments that require them to deal with a number of duties throughout numerous domains.
Historically, the instruments and strategies to sort out these issues have relied on mounted coaching examples or in-context studying. These strategies allow brokers to carry out effectively on predefined motion sequences however fall quick when dealing with novel conditions or duties that differ from their coaching information. For instance, brokers educated on particular procuring duties could fail when requested to navigate a brand new web site or full a distinct job, corresponding to reserving a flight or retrieving social media info. The rigidity of those approaches limits the generalization functionality of brokers throughout diverse duties and environments.
A analysis group from the Carnegie Mellon College & the Massachusetts Institute of Expertise (MIT) has launched a brand new technique referred to as Agent Workflow Reminiscence (AWM) to handle these challenges. AWM helps brokers study reusable job workflows from their previous experiences, which they will apply to future duties. This technique allows brokers to generate and retailer workflows—frequent sequences of actions—from beforehand solved duties, making it potential to reuse them in several contexts. AWM may be utilized in offline and on-line settings, the place workflows are pre-trained or induced in real-time from take a look at queries, providing a flexible resolution for internet navigation duties.
Intimately, AWM works by analyzing the agent’s previous experiences and extracting workflows from profitable job completions. These workflows encompass goal-oriented routines saved within the agent’s reminiscence for future use. For instance, an agent would possibly study a primary workflow for locating a spot by its identify on a map. It may possibly then construct on this by studying extra advanced workflows, corresponding to retrieving the ZIP code for the situation. This memory-based strategy permits the agent to adapt to more and more advanced duties by leveraging beforehand discovered workflows to tell future actions.
Relating to efficiency, AWM was examined on two main benchmarks—Mind2Web and WebArena—which encompass over 1,000 duties spanning greater than 200 domains, together with journey, procuring, and social media. AWM considerably improved the baseline efficiency. On the Mind2Web benchmark, the success charge of duties elevated by 24.6%, whereas on WebArena, the relative success charge improved by 51.1%. Additional, AWM decreased the variety of steps required to finish duties on WebArena, attaining as much as a 22.5-point enchancment over conventional strategies after processing solely tens of examples. These outcomes display AWM’s potential to boost the effectivity and adaptableness of brokers in numerous digital duties.
The researchers additionally discovered that AWM improved generalization throughout duties, web sites, and domains. In cross-task and cross-domain evaluations, AWM surpassed different baseline strategies by 8.9 to 14.0 absolute share factors. This generalization potential is especially noteworthy, because it reveals that AWM can adapt to duties that differ considerably from these the agent was initially educated on. For instance, an agent educated on duties involving procuring web sites might successfully generalize to different domains, corresponding to social media or journey, without having extra domain-specific coaching information.
In conclusion, the introduction of Agent Workflow Reminiscence provides a promising resolution to the constraints of current internet navigation brokers. By enabling brokers to study and reuse workflows from previous experiences, AWM improves job effectivity and adaptableness, making these methods extra versatile in dealing with advanced, long-horizon duties. The outcomes from testing on Mind2Web and WebArena clearly present the strategy’s potential to revolutionize internet navigation, permitting brokers to deal with a broader vary of duties with improved efficiency and fewer steps. This strategy marks a big development in growing extra clever and versatile digital brokers able to generalizing throughout numerous duties and domains.
Try the Paper. All credit score for this analysis goes to the researchers of this mission. Additionally, don’t neglect to comply with us on Twitter and be part of our Telegram Channel and LinkedIn Group. In the event you like our work, you’ll love our publication..
Don’t Overlook to affix our 50k+ ML SubReddit
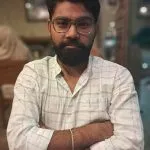
Nikhil is an intern guide at Marktechpost. He’s pursuing an built-in twin diploma in Supplies on the Indian Institute of Expertise, Kharagpur. Nikhil is an AI/ML fanatic who’s all the time researching functions in fields like biomaterials and biomedical science. With a powerful background in Materials Science, he’s exploring new developments and creating alternatives to contribute.