Mannequin merging, significantly throughout the realm of enormous language fashions (LLMs), presents an intriguing problem that addresses the rising demand for versatile AI programs. These fashions usually possess specialised capabilities similar to multilingual proficiency or domain-specific experience, making their integration essential for creating extra strong, multi-functional programs. Nonetheless, merging LLMs successfully will not be trivial; it usually requires deep experience and important computational sources to stability totally different coaching strategies and fine-tuning processes with out deteriorating general efficiency. To simplify this course of and scale back the complexity related to present mannequin merging strategies, researchers are striving to develop extra adaptive, much less resource-intensive merging strategies.
Researchers from Arcee AI and Liquid AI suggest a novel merging approach referred to as Differentiable Adaptive Merging (DAM). DAM goals to sort out the complexities of merging language fashions by providing an environment friendly, adaptive methodology that reduces the computational overhead usually related to present mannequin merging practices. Particularly, DAM offers a substitute for compute-heavy approaches like evolutionary merging by optimizing mannequin integration by way of scaling coefficients, enabling easier but efficient merging of a number of LLMs. The researchers additionally carried out a comparative evaluation of DAM in opposition to different merging approaches, similar to DARE-TIES, TIES-Merging, and easier strategies like Mannequin Soups, to focus on its strengths and limitations.
The core of DAM is its potential to merge a number of LLMs utilizing a data-informed strategy, which includes studying optimum scaling coefficients for every mannequin’s weight matrix. The tactic is relevant to varied parts of the fashions, together with linear layers, embedding layers, and layer normalization layers. DAM works by scaling every column of the burden matrices to stability the enter options from every mannequin, thus making certain that the merged mannequin retains the strengths of every contributing mannequin. The target operate of DAM combines a number of parts: minimizing Kullback-Leibler (KL) divergence between the merged mannequin and the person fashions, cosine similarity loss to encourage variety in scaling coefficients, and L1 and L2 regularization to make sure sparsity and stability throughout coaching. These components work in tandem to create a sturdy and well-integrated merged mannequin able to dealing with various duties successfully.

The researchers carried out in depth experiments to match DAM with different mannequin merging strategies. The analysis was carried out throughout totally different mannequin households, similar to Mistral and Llama 3, and concerned merging fashions with various capabilities, together with multilingual processing, coding proficiency, and mathematical reasoning. The outcomes confirmed that DAM not solely matches however, in some instances, outperforms extra computationally demanding strategies like Evolutionary Merging. For instance, in a case examine specializing in Japanese language processing and mathematical reasoning, DAM demonstrated superior adaptability, successfully balancing the specialised capabilities of various fashions with out the intensive computational necessities of different strategies. Efficiency was measured utilizing a number of metrics, with DAM usually scoring greater or on par with options throughout duties involving language comprehension, mathematical reasoning, and structured question processing.
The analysis concludes that DAM is a sensible answer for merging LLMs with diminished computational value and handbook intervention. This examine additionally emphasizes that extra complicated merging strategies, whereas highly effective, don’t all the time outperform easier options like linear averaging when fashions share related traits. DAM proves that specializing in effectivity and scalability with out sacrificing efficiency can present a major benefit in AI improvement. Shifting ahead, researchers intend to discover DAM’s scalability throughout totally different domains and languages, doubtlessly increasing its affect on the broader AI panorama.
Try the Paper. All credit score for this analysis goes to the researchers of this venture. Additionally, don’t neglect to observe us on Twitter and be a part of our Telegram Channel and LinkedIn Group. In case you like our work, you’ll love our e-newsletter.. Don’t Neglect to hitch our 50k+ ML SubReddit.
[Upcoming Live Webinar- Oct 29, 2024] The Greatest Platform for Serving High quality-Tuned Fashions: Predibase Inference Engine (Promoted)
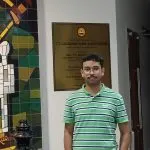
Sana Hassan, a consulting intern at Marktechpost and dual-degree pupil at IIT Madras, is obsessed with making use of know-how and AI to deal with real-world challenges. With a eager curiosity in fixing sensible issues, he brings a recent perspective to the intersection of AI and real-life options.