Growing therapeutics is dear and time-consuming, usually taking 10-15 years and as much as $2 billion, with most drug candidates failing throughout medical trials. A profitable therapeutic should meet numerous standards, similar to goal interplay, non-toxicity, and appropriate pharmacokinetics. Present AI fashions give attention to specialised duties inside this pipeline, however their restricted scope can hinder efficiency. The Therapeutics Information Commons (TDC) gives datasets to assist AI fashions predict drug properties, but these fashions work independently. LLMs, which excel at multi-tasking, present the potential to enhance therapeutic improvement by studying throughout various duties utilizing a unified method.
LLMs, significantly transformer-based fashions, have superior pure language processing, excelling in duties by way of self-supervised studying on massive datasets. Current research present LLMs can deal with various duties, together with regression, utilizing textual representations of parameters. In therapeutics, specialised fashions like graph neural networks (GNNs) symbolize molecules as graphs for capabilities similar to drug discovery. Protein and nucleic acid sequences are additionally encoded to foretell properties like binding and construction. LLMs are more and more utilized in biology and chemistry, with fashions like LlaSMol and protein-specific fashions reaching promising ends in drug synthesis and protein engineering duties.
Researchers from Google Analysis and Google DeepMind launched Tx-LLM, a generalist massive language mannequin fine-tuned from PaLM-2, designed to deal with various therapeutic duties. Educated on 709 datasets protecting 66 capabilities throughout the drug discovery pipeline, Tx-LLM makes use of a single set of weights to course of numerous chemical and organic entities, similar to small molecules, proteins, and nucleic acids. It achieves aggressive efficiency on 43 duties and surpasses state-of-the-art on 22. Tx-LLM excels in duties combining molecular representations with textual content and exhibits optimistic switch between totally different drug sorts. This mannequin is a precious software for end-to-end drug improvement.
The researchers compiled a dataset assortment referred to as TxT, containing 709 drug discovery datasets from the TDC repository, specializing in 66 duties. Every dataset was formatted for instruction tuning, that includes 4 elements: directions, context, query, and reply. These duties included binary classification, regression, and technology duties, with representations like SMILES strings for molecules and amino acid sequences for proteins. Tx-LLM was fine-tuned from PaLM-2 utilizing this knowledge. They evaluated the mannequin’s efficiency utilizing metrics similar to AUROC and Spearman correlation and set accuracy. Statistical checks and knowledge contamination analyses had been carried out to make sure sturdy outcomes.
The Tx-LLM mannequin demonstrated robust efficiency on TDC datasets, surpassing or matching state-of-the-art (SOTA) outcomes on 43 out of 66 duties. It outperformed SOTA on 22 datasets and achieved near-SOTA efficiency on 21 others. Notably, Tx-LLM excelled in datasets combining SMILES molecular strings with textual content options like illness or cell line descriptions, probably as a consequence of its pretrained information of the textual content. Nonetheless, it struggled on datasets that relied solely on SMILES strings, the place graph-based fashions had been simpler. General, the outcomes spotlight the strengths of fine-tuned language fashions for duties involving medicine and text-based options.
Tx-LLM is the primary LLM skilled on various TDC datasets, together with molecules, proteins, cells, and illnesses. Apparently, coaching with non-small molecule datasets, similar to proteins, improved efficiency on small molecule duties. Whereas common LLMs have struggled with specialised chemistry duties, Tx-LLM excelled in regression, outperforming state-of-the-art fashions in a number of circumstances. This mannequin exhibits potential for end-to-end drug improvement, from gene identification to medical trials. Nonetheless, Tx-LLM remains to be within the analysis stage, with limitations in pure language instruction and prediction accuracy, requiring additional enchancment and validation for broader purposes.
Take a look at the Paper and Particulars. All credit score for this analysis goes to the researchers of this mission. Additionally, don’t neglect to comply with us on Twitter and be a part of our Telegram Channel and LinkedIn Group. In the event you like our work, you’ll love our e-newsletter.. Don’t Overlook to hitch our 50k+ ML SubReddit
[Upcoming Event- Oct 17 202] RetrieveX – The GenAI Information Retrieval Convention (Promoted)
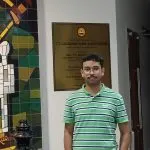
Sana Hassan, a consulting intern at Marktechpost and dual-degree scholar at IIT Madras, is enthusiastic about making use of expertise and AI to handle real-world challenges. With a eager curiosity in fixing sensible issues, he brings a contemporary perspective to the intersection of AI and real-life options.