In recent times, there was important improvement within the area of huge pre-trained fashions for studying robotic insurance policies. The time period “coverage illustration” right here refers back to the alternative ways of interfacing with the decision-making mechanisms of robots, which may doubtlessly facilitate generalization to new duties and environments. Imaginative and prescient-language-action (VLA) fashions are pre-trained with large-scale robotic knowledge to combine visible notion, language understanding, and action-based decision-making to information robots in varied duties. On prime of vision-language fashions (VLMs), they give you the promise of generalization to new objects, scenes, and duties. Nonetheless, VLAs nonetheless should be extra dependable to be deployed outdoors the slender lab settings they’re skilled in. Whereas these drawbacks will be mitigated by increasing the scope and variety of robotic datasets, that is extremely resource-intensive and difficult to scale. In easy phrases, these coverage representations both want to offer extra context or over-specified context that yields much less sturdy insurance policies.
Current coverage representations resembling language, aim photos, and trajectory sketches are broadly used and are useful. One of the crucial frequent coverage representations is conditioning on language. A lot of the robotic datasets are labeled with underspecified descriptions of the duty, and language-based steering doesn’t present sufficient steering on the way to carry out the duty. Objective image-conditioned insurance policies present detailed spatial details about the ultimate aim configuration of the scene. Nonetheless, aim photos are high-dimensional, which presents studying challenges because of over-specification points. Intermediate illustration resembling Trajectory sketches, or key factors makes an attempt to offer spatial plans for guiding the robotic’s actions. Whereas these spatial plans present steering, they nonetheless lack ample info for the coverage on the way to carry out particular actions.
A workforce of researchers from Google DeepMind performed detailed analysis on coverage illustration for robots and proposed RT-Affordance which is a hierarchical mannequin that first creates an affordance plan given the duty language, after which makes use of the coverage on this affordance plan to information the robotic’s actions for manipulation. In robotics, affordance refers back to the potential interactions that an object permits for a robotic, based mostly on its form, measurement and so forth. The RT-Affordance mannequin can simply join heterogeneous sources of supervision together with giant internet datasets and robotic trajectories.
First, the affordance plan is predicted for the given job language and the preliminary picture of the duty. This affordance plan is then mixed with language directions to situation the coverage for job execution. It’s then projected onto the picture, and following this, the coverage is conditioned on photos overlaid with the affordance plan. The mannequin is co-trained on internet datasets (the most important knowledge supply), robotic trajectories, and a modest variety of cheap-to-collect photos labeled with affordances. This method advantages from leveraging each robotic trajectory knowledge and in depth internet datasets, permitting the mannequin to generalize properly throughout new objects, scenes, and duties.
The analysis workforce performed varied experiments that primarily targeted on how affordances assist to enhance robotic greedy, particularly for actions of home items with complicated shapes (like kettles, dustpans, and pots). An in depth analysis confirmed that RT-A stays sturdy throughout varied out-of-distribution (OOD) eventualities, resembling novel objects, digital camera angles, and backgrounds. The RT-A mannequin carried out higher than RT-2 and its goal-conditioned variant, reaching success charges of 68%-76% in comparison with RT-2’s 24%-28%. In duties past greedy, like putting objects into containers, RT-A confirmed a big efficiency with a 70% success charge. Nonetheless, the efficiency of RT-A barely dropped when it confronted completely new objects.
In conclusion, affordance-based insurance policies are well-guided and in addition carry out in a greater manner. The RT- Affordance technique considerably improves the robustness and generalization of robotic insurance policies, which makes it a worthwhile device for numerous manipulation duties. Though it cannot adapt to thoroughly new moments or expertise, RT-Affordance surpasses conventional strategies by way of efficiency. This affordance method opens the gate for varied future analysis alternatives in robotics and may function a baseline for future research!
Try the Paper. All credit score for this analysis goes to the researchers of this challenge. Additionally, don’t neglect to observe us on Twitter and be a part of our Telegram Channel and LinkedIn Group. In the event you like our work, you’ll love our e-newsletter.. Don’t Neglect to affix our 55k+ ML SubReddit.
[Sponsorship Opportunity with us] Promote Your Analysis/Product/Webinar with 1Million+ Month-to-month Readers and 500k+ Neighborhood Members
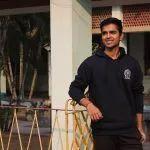
Divyesh is a consulting intern at Marktechpost. He’s pursuing a BTech in Agricultural and Meals Engineering from the Indian Institute of Know-how, Kharagpur. He’s a Information Science and Machine studying fanatic who needs to combine these main applied sciences into the agricultural area and resolve challenges.