Giant language fashions (LLMs) have demonstrated vital reasoning capabilities, but they face points like hallucinations and the lack to conduct trustworthy reasoning. These challenges stem from information gaps, resulting in factual errors throughout complicated duties. Whereas information graphs (KGs) are more and more used to bolster LLM reasoning, present KG-enhanced approaches—retrieval-based and agent-based—battle with both correct information retrieval or effectivity in reasoning on a big scale.
Researchers from Monash College, Nanjing College of Science and Know-how, and Griffith College suggest a novel framework known as Graph-Constrained Reasoning (GCR). This framework goals to bridge the hole between structured information in KGs and the unstructured reasoning of LLMs, guaranteeing trustworthy, KG-grounded reasoning. GCR introduces a trie-based index named KG-Trie to combine KG constructions straight into the LLM decoding course of. This integration allows LLMs to generate reasoning paths grounded in KGs, minimizing hallucinations. GCR additionally employs two fashions: a light-weight KG-specialized LLM for environment friendly reasoning on graphs and a strong basic LLM for inductive reasoning over a number of generated paths.
The GCR framework contains three predominant elements. Firstly, it constructs a Information Graph Trie (KG-Trie), which acts as a structured index to information LLM reasoning by encoding the paths inside the KG. Secondly, GCR makes use of a course of known as graph-constrained decoding, using a KG-specialized LLM to generate KG-grounded reasoning paths and hypothesized solutions. The KG-Trie constrains the LLM’s decoding course of, guaranteeing all reasoning paths are legitimate and rooted within the KG. Lastly, GCR leverages the inductive reasoning capabilities of a basic LLM, which processes a number of generated reasoning paths to derive last, correct solutions.
Intensive experiments show that GCR achieves state-of-the-art efficiency throughout a number of KGQA benchmarks, together with WebQSP and CWQ. Notably, GCR surpassed earlier strategies by 2.1% and 9.1% in accuracy (Hit) on these datasets, respectively. The framework efficiently eradicated hallucinations, reaching a 100% trustworthy reasoning ratio, and exhibited robust zero-shot generalizability to unseen KGs with out extra coaching. For instance, GCR confirmed a powerful 7.6% improve in accuracy when examined on a brand new commonsense information graph, in comparison with baseline LLMs.
In conclusion, GCR presents a sturdy answer to the challenges of trustworthy reasoning in LLMs by straight integrating structured KGs into the reasoning course of. The usage of a KG-Trie for decoding constraints and the dual-model strategy that leverages each KG-specialized and basic LLMs leads to trustworthy, environment friendly, and correct reasoning. This strategy ensures that LLMs generate dependable outputs with out hallucinations, showcasing its potential as a reliable methodology for large-scale reasoning duties involving structured and unstructured information.
Try the Paper and GitHub. All credit score for this analysis goes to the researchers of this challenge. Additionally, don’t neglect to observe us on Twitter and be a part of our Telegram Channel and LinkedIn Group. In the event you like our work, you’ll love our e-newsletter.. Don’t Neglect to hitch our 50k+ ML SubReddit.
[Upcoming Live Webinar- Oct 29, 2024] The Finest Platform for Serving Nice-Tuned Fashions: Predibase Inference Engine (Promoted)
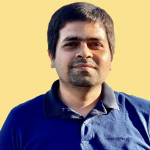
Asif Razzaq is the CEO of Marktechpost Media Inc.. As a visionary entrepreneur and engineer, Asif is dedicated to harnessing the potential of Synthetic Intelligence for social good. His most up-to-date endeavor is the launch of an Synthetic Intelligence Media Platform, Marktechpost, which stands out for its in-depth protection of machine studying and deep studying information that’s each technically sound and simply comprehensible by a large viewers. The platform boasts of over 2 million month-to-month views, illustrating its recognition amongst audiences.