One of many core challenges in semilocal density useful idea (DFT) is the constant underestimation of band gaps, primarily on account of self-interaction and delocalization errors. This subject complicates the prediction of digital properties and cost switch mechanisms. Hybrid DFT, incorporating a fraction of tangible trade power, gives improved band hole predictions however typically requires system-specific tuning. Machine studying approaches have emerged to reinforce DFT accuracy, notably for molecular response energies and strongly correlated techniques. Explicitly becoming power gaps, as demonstrated by the DM21 useful, may enhance DFT predictions by addressing self-interaction errors.
Researchers from Harvard SEAS have developed a machine studying methodology utilizing Gaussian processes to reinforce the precision of density functionals for predicting power gaps and response energies. Their mannequin integrates nonlocal options of the density matrix to forecast molecular power gaps precisely and estimate polaron formation energies in solids, regardless that it was educated solely on molecular information. This development builds upon the CIDER framework, which is thought for its effectivity and scalability in dealing with massive techniques. Though the mannequin presently targets trade power, it holds promise for broader functions, together with predicting digital properties resembling band gaps.
The research introduces key ideas and strategies for becoming exchange-correlation (XC) functionals in DFT, specializing in band hole prediction and single-particle energies. It covers the appliance of Gaussian course of regression to mannequin XC functionals, incorporating coaching options that improve accuracy. The theoretical basis relies on Janak’s theorem and the idea of by-product discontinuity, that are essential for predicting properties like ionization potentials, electron affinities, and band gaps inside generalized Kohn-Sham DFT. The method goals to enhance useful coaching by addressing the challenges of orbital occupation and by-product discontinuities.
The CIDER24X trade power mannequin was developed utilizing a Gaussian course of, enhancing flexibility. Key options have been chosen for minimal covariance, adjusted to a particular vary, and employed to coach a dense neural community approximating the Gaussian course of. The coaching information comprised uniform electron fuel trade power, molecular power variations, and power ranges sourced from databases resembling W4-11, G21IP, and 3d-SSIP30. Two variants of the CIDER24X mannequin have been created: one incorporating power stage information (CIDER24X-e) and one other excluding it (CIDER24X-ne) to judge the impact of together with power ranges within the becoming course of.
The research introduces the CIDER24X mannequin with SDMX options, exhibiting enhanced predictive accuracy for molecular energies and HOMO-LUMO gaps in comparison with earlier fashions and semilocal functionals. CIDER24X-ne, educated with out power ranges, aligns intently with PBE0, whereas CIDER24X-e, which incorporates power stage information, gives a greater steadiness between power and band hole predictions. Although trade-offs exist, notably with eigenvalue coaching, CIDER24X-e outperforms semilocal functionals and approaches hybrid DFT accuracy, making it a promising different that reduces computational prices.
In conclusion, The research presents a framework for becoming density functionals to each whole power and single-particle power ranges utilizing machine studying, leveraging Janak’s theorem. A brand new set of options, SDMX, is launched to be taught the trade perform with out requiring the total trade operator. The mannequin, CIDER24X-e, maintains accuracy in molecular energies whereas considerably bettering power hole predictions, matching hybrid DFT outcomes. The framework is extendable to full XC functionals and different machine studying fashions, providing the potential for environment friendly and correct digital property predictions throughout various techniques.
Take a look at the Paper. All credit score for this analysis goes to the researchers of this venture. Additionally, don’t neglect to observe us on Twitter and be part of our Telegram Channel and LinkedIn Group. For those who like our work, you’ll love our publication..
Don’t Overlook to hitch our 50k+ ML SubReddit
Here’s a extremely beneficial webinar from our sponsor: ‘Constructing Performant AI Functions with NVIDIA NIMs and Haystack’
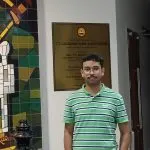
Sana Hassan, a consulting intern at Marktechpost and dual-degree scholar at IIT Madras, is captivated with making use of expertise and AI to handle real-world challenges. With a eager curiosity in fixing sensible issues, he brings a recent perspective to the intersection of AI and real-life options.