Instruction-tuned LMs have proven outstanding zero-shot generalization however usually fail on duties exterior their coaching information. These LMs, constructed on giant datasets and billions of parameters, excel in In-Context Studying (ICL), producing responses primarily based on a number of examples with out re-training. Nevertheless, the coaching dataset’s scope limits its effectiveness on unfamiliar duties. Methods like immediate engineering and output diversification assist enhance efficiency however require vital effort. Latest analysis explores making use of the cognitive anchoring impact to LMs, suggesting that emphasizing preliminary prompts can improve task-specific responses and enhance constancy to directions.
Researchers from KAIST AI launched Instructive Decoding (ID), a way that enhances instruction-tuned LMs with out parameter updates. ID makes use of “noisy directions,” altered variations of the unique directions, to create a contrastive method for predicting the following token. By steering the mannequin’s output in numerous instructions, particularly utilizing “reverse” directions, ID improves mannequin efficiency throughout duties. Experiments present vital positive factors in accuracy, with smaller fashions enhanced by ID outperforming bigger ones. This methodology improves adherence to directions and enhances total response high quality, demonstrating its effectiveness throughout numerous fashions and duties.
Instruction-tuning fine-tunes pre-trained LMs to observe pure language directions higher, enhancing generalization to unseen duties, particularly in zero-shot situations. Increasing the range and complexity of coaching duties enhances this functionality, though the fashions usually rely closely on pre-trained data. Prior analysis highlights that LMs are delicate to acquainted directions, even dealing with deceptive ones, and this sensitivity might be leveraged by way of contrastive methods. Distinction in textual content era, like Contrastive Decoding, compares outputs from totally different fashions or inputs to enhance efficiency. This examine extends these concepts by utilizing noisy directions to spice up generalization in instruction-tuned LMs.
Instructive Decoding improves response era in instruction-tuned fashions by contrasting outputs generated from noisy directions. It builds on the anchoring impact, the place preliminary data influences subsequent judgments and leverages variations between responses generated from unique and altered directions. The tactic makes use of noisy instruction variants like truncated, shuffled, or random phrases to mislead the mannequin whereas making certain activity constancy. By evaluating logits from unique and noisy directions throughout decoding, Instructive Decoding helps fashions appropriate biases and produce responses extra aligned with the supposed directions, refining their efficiency on unseen duties.
The experimental setup makes use of the SUPNATINST and UNNATINST datasets, evaluating fashions like Tk-Instruct, Alpaca, and T0 throughout duties like Grammar Error Correction and Textual Entailment. Rouge-L, Precise Match (EM), Label Adherence (LA), and Label Coherence (LC) metrics assess efficiency. ID constantly improves outcomes, particularly for bigger fashions like Tk-XXL, enhancing LA and LC. Apparently, noisy directions improve output high quality with ID regardless of baseline efficiency degradation. Although task-specific efficiency varies, the ‘reverse’ instruction variant proves strong throughout duties. Total, ID reveals vital positive factors throughout mannequin sizes and activity varieties.
The examine investigates the challenges of unseen activity generalization in instruction-tuned language fashions. The proposed methodology, ID, leverages the anchoring impact utilizing “noisy” directions to counteract inherent mannequin biases. By contrasting predictions with these generated from altered directions, ID enhances mannequin efficiency, notably with the “reverse” noisy variant, which deviates most from the unique enter. Empirical outcomes present ID’s effectiveness throughout a number of duties, with notable enhancements in prediction range. The method requires no further parameter updates, making it a sensible software for enhancing instruction-following in language fashions.
Try the Paper. All credit score for this analysis goes to the researchers of this challenge. Additionally, don’t overlook to observe us on Twitter and be a part of our Telegram Channel and LinkedIn Group. For those who like our work, you’ll love our e-newsletter..
Don’t Overlook to hitch our 50k+ ML SubReddit
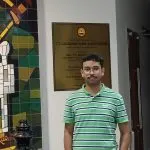
Sana Hassan, a consulting intern at Marktechpost and dual-degree scholar at IIT Madras, is obsessed with making use of expertise and AI to deal with real-world challenges. With a eager curiosity in fixing sensible issues, he brings a contemporary perspective to the intersection of AI and real-life options.