Constructing on LG AI Analysis’s exceptional achievements in AI language fashions, particularly with the launch of EXAONE 3.0, the event of EXAONEPath represents one other important milestone. This new chapter in EXAONE’s journey focuses on reworking digital histopathology, a essential space in medical diagnostics, by addressing the complicated challenges of Complete Slide Pictures (WSIs) in histopathology and by enabling the environment friendly processing of histopathology pictures, EXAONEPath is well-utilized for varied medical duties together with prediction of genetic mutations and/or suggestion of essentially the most appropriate therapy strategies and medicines. This innovation reduces the time required for genetic testing, which historically took as much as two weeks, thereby saving money and time and enhancing affected person care. The introduction of EXAONEPath highlights LG AI Analysis’s dedication to advancing AI applied sciences in specialised and difficult domains, reinforcing its imaginative and prescient of democratizing entry to skilled AI.
Introduction to EXAONEPath: A New Frontier in Digital Histopathology
EXAONEPath is designed as a patch-level foundational mannequin that operates on WSIs, that are high-resolution pictures of tissue slides utilized in histopathology. Typically containing over the billions of pixels, these pictures are essential for most cancers subtyping, prognosis prediction, and tissue microenvironment evaluation. Nevertheless, the standard fashions educated on these pictures usually endure from a phenomenon generally known as WSI-specific function collapse, the place the options extracted by the mannequin are inclined to cluster based mostly on the person WSI quite than the pathological traits of the tissue. This clustering can considerably restrict the mannequin’s capability to generalize throughout totally different WSIs and, consequently, its effectiveness in real-world purposes.
Technical Improvements in EXAONEPath: Overcoming WSI-Particular Characteristic Collapse
On the core of EXAONEPath’s innovation is its method to overcoming the WSI-specific function collapse. This mannequin employs self-supervised studying and stain normalization methods, particularly Macenko normalization, to standardize the colour traits of WSIs earlier than function extraction. This course of reduces the variability launched by totally different staining protocols throughout laboratories, which is a main explanation for function collapse. By making use of this normalization, EXAONEPath ensures that the options it learns are extra targeted on the pathologically important facets of the tissue, corresponding to nuclear measurement and form, cell density, and structural modifications, quite than superficial coloration variations.
There are a number of distinctive challenges addressed by EXAONEPath as follows:
- Multi-Occasion Studying (MIL) Framework: A Cornerstone in Histopathology Picture Processing: One of many essential challenges in processing histopathology pictures, significantly WSIs, is their immense measurement and the intricate particulars they comprise. Conventional picture processing strategies usually need assistance to deal with these high-resolution pictures successfully. That is the place the MIL framework comes into play, changing into a cornerstone in histopathology picture evaluation. A WSI is split into smaller patches or tiles within the MIL framework. Every tile is then processed by means of a pre-trained picture encoder, changing it right into a latent vector. These vectors, which encapsulate the morphological traits of the cells inside every tile, are then built-in to kind a complete latent vector representing the complete slide. This method ensures that the intricate particulars of cell constructions and surrounding tissues are preserved, at the same time as the information is processed at a manageable scale. EXAONEPath leverages this MIL framework to excel in processing gigapixel-scale histopathology pictures. By using self-supervised studying strategies, corresponding to DINO, and mixing them with stain normalization methods, EXAONEPath can mitigate the challenges posed by WSI-specific function collapse. This functionality enhances the mannequin’s efficiency and makes it an important software in digital histopathology, the place correct and detailed picture evaluation is essential for prognosis and therapy planning.
- Advancing Self-Supervised Studying: Overcoming Shade-Primarily based Characteristic Collapse: Histopathology pictures are distinctive in composition, usually containing refined but important variations in cell construction and tissue group. Nevertheless, a standard challenge in coaching fashions on these pictures is the phenomenon of color-based function collapse, significantly when utilizing self-supervised studying strategies like DINO. This happens when the mannequin, as a substitute of studying the essential options associated to cell morphology and tissue construction, primarily focuses on coloration variations throughout totally different slides. EXAONEPath employs a complicated method generally known as stain normalization to deal with this. This course of includes deciding on a high-quality, well-stained picture as a reference and reworking different pictures to match its coloration profile. By doing so, the mannequin can deal with studying the important pathological options quite than getting biased by coloration discrepancies. The effectiveness of this method is clear within the mannequin’s efficiency, the place post-normalization, the patches are now not clustered based mostly on coloration however are as a substitute evenly distributed based mostly on their latent options. This development improves the standard of the mannequin’s outputs and units a brand new commonplace within the coaching of histopathology picture encoders.
Coaching EXAONEPath: A Rigorous and Moral Method
The event of EXAONEPath concerned a complete and ethically accountable coaching course of. The mannequin was educated on 285,153,903 patches extracted from 34,795 WSIs, making certain a various and consultant dataset. The coaching was performed utilizing DINO (self-Distillation with NO labels), a self-supervised studying methodology that enhances the mannequin’s capability to generalize from giant quantities of unlabeled knowledge. This method allowed the mannequin to be taught sturdy options essential for downstream duties corresponding to most cancers classification and survival evaluation.
A key facet of this coaching course of was the strict adherence to knowledge high quality and compliance requirements. LG AI Analysis fastidiously curated the coaching knowledge to incorporate pathological circumstances, making certain the mannequin would apply to numerous medical situations. Furthermore, by incorporating moral concerns all through the mannequin’s growth, LG AI Analysis ensured that EXAONEPath can be a dependable and reliable software for pathologists.
Efficiency Analysis: Benchmarking EXAONEPath In opposition to the State-of-the-Artwork
EXAONEPath’s efficiency was rigorously evaluated throughout six numerous patch-level duties, together with PCAM (Pathology Classification utilizing Consideration Fashions), MHIST (Micro-Histology Picture Segmentation Process), and CRC-100K (Colorectal Most cancers Patch Classification). The mannequin was benchmarked towards state-of-the-art fashions, and the outcomes had been spectacular.
The efficiency of the EXAONEPath mannequin stands out in a comparability throughout a number of benchmarks towards different state-of-the-art fashions. Particularly, EXAONEPath demonstrates aggressive outcomes with a mean rating of 0.861, surpassing many different fashions, corresponding to ViT-L/16 ImageNet and Phikon, and its accuracy is corresponding to competing fashions, corresponding to GigaPath. Notably, EXAONEPath excels within the MSI CRC and MSI STAD duties, attaining scores of 0.756 and 0.804, respectively, that are the best in these classes. Whereas it barely trails in some duties like PCAM and CRC-100K, the mannequin nonetheless performs robustly throughout the board, showcasing its effectivity and functionality in dealing with complicated histopathology picture evaluation. This efficiency highlights EXAONEPath’s sturdy potential as a flexible and efficient software in digital histopathology, particularly contemplating its comparatively smaller measurement and the effectivity of its coaching course of.
New Horizons: Potential Purposes and Future Instructions
The success of EXAONEPath opens up new potentialities for making use of AI in histopathology. By offering a dependable and environment friendly mannequin for WSI evaluation, EXAONEPath has the potential to revolutionize a number of facets of medical diagnostics, from most cancers detection to personalised drugs. The mannequin’s capability to deal with giant and complicated datasets makes it a helpful software for pathologists, who can enhance diagnostic accuracy and cut back the time required for evaluation. Going ahead, there are a number of thrilling instructions for future analysis. One space of focus could possibly be the event of extra superior stain normalization methods which might be computationally environment friendly and may be easily built-in into present workflows. Additionally, exploring new mannequin architectures that may additional cut back function collapse and improve the generalization capabilities of AI fashions in histopathology will probably be essential.
Moral Concerns: Guaranteeing Accountable Use of AI in Histopathology
As with all highly effective AI expertise, the deployment of EXAONEPath comes with important moral obligations. LG AI Analysis has taken proactive steps to deal with these considerations, implementing strict tips to make sure the mannequin is used ethically and responsibly. This contains measures to stop the misuse of the mannequin, corresponding to prohibiting its use for business functions with out express consent and making certain that it isn’t used to generate dangerous or deceptive info. The mannequin has been totally examined to align with moral requirements, significantly in bias mitigation and consumer privateness. By embedding these moral concerns into the event and deployment of EXAONEPath, LG AI Analysis is setting a regular for the accountable use of AI in medical purposes.
Discover the Innovation of EXAONEPath: A Breakthrough in Digital Histopathology
LG AI Analysis proudly presents EXAONEPath, their groundbreaking patch-level basis mannequin for histopathology picture evaluation. Designed to excel in processing gigapixel-scale pictures, EXAONEPath leverages superior self-supervised studying and stain normalization methods to ship unparalleled accuracy in medical diagnostics. This pioneering mannequin has been launched as open-source on the Hugging Face platform, making it accessible to researchers, healthcare professionals, and AI builders globally for analysis functions. EXAONEPath not solely units new requirements within the subject of digital histopathology but in addition unlocks transformative potentialities for AI-driven healthcare improvements. LG AI Analysis invitations the worldwide group to discover the highly effective capabilities of EXAONEPath and to remain engaged by means of their LinkedIn web page for the most recent analysis, updates, and collaborative alternatives. Additionally, customers, researchers, and professionals can observe the most recent updates on the LG AI Analysis Web site, as many new releases are within the line for the EXAONE sequence.
Conclusion: A New Period in Digital Histopathology
EXAONEPath is a exceptional feat in digital histopathology and one other welcome boost to EXAONE analysis pursued by the LG AI Analysis group. It builds on the foundational work of EXAONE 3.0 and pushes the boundaries of what AI can obtain in medical diagnostics. By addressing the challenges of WSI-specific function collapse and enhancing the generalization capabilities of AI fashions, EXAONEPath will grow to be a helpful software for pathologists worldwide. As this journey continues, the teachings realized from EXAONEPath will undoubtedly inform the following technology of AI fashions, paving the best way for extra correct, environment friendly, and moral diagnostic instruments. With this new addition, LG AI Analysis’s imaginative and prescient of democratizing entry to expert-level AI extends into the medical subject.
I hope you loved studying the 2nd article of this sequence from LG AI Analysis. When you have not learn the first article (EXAONE 3.0), You must proceed studying the first article (EXAONE 3.0) right here…
Sources
Due to the LG AI Analysis group for the thought management/ Assets for this text. LG AI Analysis group has supported us on this content material/article.
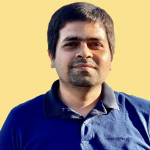
Asif Razzaq is the CEO of Marktechpost Media Inc.. As a visionary entrepreneur and engineer, Asif is dedicated to harnessing the potential of Synthetic Intelligence for social good. His most up-to-date endeavor is the launch of an Synthetic Intelligence Media Platform, Marktechpost, which stands out for its in-depth protection of machine studying and deep studying information that’s each technically sound and simply comprehensible by a large viewers. The platform boasts of over 2 million month-to-month views, illustrating its reputation amongst audiences.