Latest developments in medical multimodal massive language fashions (MLLMs) have proven vital progress in medical decision-making. Nonetheless, many fashions, akin to Med-Flamingo and LLaVA-Med, are designed for particular duties and require massive datasets and excessive computational assets, limiting their practicality in medical settings. Whereas the Combination-of-Professional (MoE) technique gives an answer utilizing smaller, task-specific modules to cut back computational price, its software within the medical area stays underexplored. Light-weight but efficient fashions that deal with various duties and supply higher scalability are important for broader medical utility in resource-constrained environments.
Researchers from Zhejiang College, the Nationwide College of Singapore, and Peking College launched Med-MoE, a light-weight framework for multimodal medical duties like Med-VQA and picture classification. Med-MoE integrates domain-specific specialists with a world meta-expert, emulating hospital workflows. The mannequin aligns medical photos and textual content, makes use of instruction tuning for multimodal duties, and employs a router to activate related specialists. Med-MoE outperforms or matches state-of-the-art fashions like LLaVA-Med with solely 30%-50% of activated parameters. Examined on datasets like VQA-RAD and Path-VQA, it reveals robust potential for enhancing medical decision-making in resource-constrained settings.
Developments in MLLMs like Med-Flamingo, Med-PaLM M, and LLaVA-Med have considerably improved medical diagnostics by constructing on basic AI fashions akin to ChatGPT and GPT-4. These fashions improve capabilities in few-shot studying and medical query answering however are sometimes expensive and underutilized in resource-limited settings. The MoE method in MLLMs improves activity dealing with and effectivity, both activating completely different specialists for particular duties or changing commonplace layers with MoE constructions. Nonetheless, these strategies typically battle with modal biases and lack efficient specialization for various medical information.
The Med-MoE framework trains in three phases. First, within the Multimodal Medical Alignment section, the mannequin aligns medical photos with textual descriptions utilizing a imaginative and prescient encoder to supply picture tokens and integrates them with textual content tokens to coach a language mannequin. Second, throughout Instruction Tuning and Routing, the mannequin learns to deal with medical duties and generates responses whereas a router is educated to determine enter modalities. Lastly, in Area-Particular MoE Tuning, the framework replaces the mannequin’s feed-forward community with an MoE construction, the place a meta-expert captures world info and domain-specific specialists deal with particular duties, optimizing the mannequin for exact medical decision-making.
The research evaluates Med-MoE fashions utilizing varied datasets and metrics, together with accuracy and recall, with base fashions StableLM (1.7B) and Phi2 (2.7B). Med-MoE (Phi2) demonstrates superior efficiency over LLaVA-Med in VQA duties and medical picture classification, reaching 91.4% accuracy on PneumoniaMNIST. MoE-Tuning constantly outperforms conventional SFT, and integration with LoRA advantages GPU reminiscence utilization and inference pace. Less complicated router architectures and specialised specialists improve mannequin effectivity, with 2-4 activated specialists successfully balancing efficiency and computation.
In conclusion, Med-MoE is a streamlined framework designed for multimodal medical duties, optimizing efficiency in resource-limited settings by aligning medical photos with language mannequin tokens, task-specific tuning, and domain-specific fine-tuning. It achieves state-of-the-art outcomes whereas lowering activated parameters. Regardless of its effectivity, Med-MoE encounters challenges akin to restricted medical coaching information as a consequence of privateness issues and excessive prices of handbook annotations. The mannequin additionally struggles with advanced, open-ended questions and should guarantee reliable, explainable outputs in important healthcare purposes. Med-MoE gives a sensible resolution for superior medical AI in constrained environments however wants enhancements in information scalability and mannequin reliability.
Try the Paper. All credit score for this analysis goes to the researchers of this undertaking. Additionally, don’t overlook to observe us on Twitter and LinkedIn. Be part of our Telegram Channel.
Should you like our work, you’ll love our publication..
Don’t Overlook to hitch our 50k+ ML SubReddit
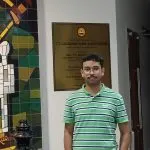
Sana Hassan, a consulting intern at Marktechpost and dual-degree pupil at IIT Madras, is obsessed with making use of expertise and AI to deal with real-world challenges. With a eager curiosity in fixing sensible issues, he brings a contemporary perspective to the intersection of AI and real-life options.