Automated scientific discovery has the potential to boost progress throughout numerous scientific fields considerably. Nevertheless, assessing an AI agent’s skill to make use of complete scientific reasoning is difficult as a result of excessive prices and impracticalities of conducting real-world experiments. Whereas current neural methods have led to profitable discovery methods for particular issues like protein folding, arithmetic, and supplies science, these methods usually circumvent the whole discovery course of, focusing as a substitute on systematic searches inside a predefined speculation area. This raises the query of how way more will be achieved if AI is utilized all through the scientific course of, together with creativity, speculation era, and experimental design.
Latest developments in real-world discovery methods have proven promise in fields like genetics, chemistry, and proteomics, although these methods are sometimes expensive and tailor-made to particular duties. Numerous digital environments have been developed for robotics, exploration, and scientific discovery, together with AI2-Thor and NetHack. Nevertheless, many of those environments prioritize leisure over rigorous scientific exploration. Whereas some, like ScienceWorld, sort out fundamental science challenges, they usually want extra complete processes for thorough scientific discovery. General, current methods differ in complexity and utility, typically emphasizing slim process efficiency fairly than fostering broad scientific analysis abilities.
Researchers from the Allen Institute, Microsoft Analysis, and the College of Arizona have developed DISCOVERYWORLD, a pioneering digital surroundings designed for brokers to conduct full cycles of scientific discovery. This text-based platform options 120 challenges throughout eight various matters, resembling rocket science and proteomics, emphasizing creating basic discovery abilities fairly than task-specific options. The surroundings permits brokers to hypothesize, experiment, analyze, and draw conclusions. Moreover, a sturdy analysis framework measures agent efficiency by way of process completion and related actions, revealing that current brokers face vital challenges on this new context, highlighting the surroundings’s potential to advance AI discovery capabilities.
The DISCOVERYWORLD simulator makes use of a customized engine to create dynamic discovery simulations with various object properties and behaviors. It consists of roughly 20,000 strains of Python code utilizing the Pygame framework, that includes an API for agent growth and a graphical interface for human interplay. The surroundings is organized as a 32 × 32 tile grid, the place brokers obtain observations in textual content and visible codecs. The simulation contains 14 doable actions and parametrically generated duties throughout eight discovery themes with three issue ranges. Analysis metrics assess process completion, course of adherence, and the accuracy of found information, enabling a complete efficiency analysis of brokers.
The research analyzes the efficiency of robust baseline brokers and human scientists on DISCOVERYWORLD duties, specializing in zero-shot generalization for iterative scientific discovery. The research evaluates three baseline fashions in a zero-shot setting throughout 120 duties, every assessed independently. Efficiency metrics embody process completion and explanatory information discovery. Outcomes point out that whereas baseline brokers present various ranges of success, there’s a notable efficiency hole in comparison with human scientists. Eleven human contributors with related scientific backgrounds have been recruited to supply insights into human efficiency.
The efficiency of human contributors in discovery duties various broadly, with completion charges starting from duties solved by all to these tackled by just one participant. On common, people achieved a 66% completion charge, whereas their information efficiency was barely decrease at 55%, generally resorting to brute-force options with out offering explanatory insights. In distinction, baseline agent efficiency was subpar, with one of the best agent, REACT, finishing solely 38% of simple duties and 18% of problem duties. DISCOVERYWORLD is a pioneering digital platform for assessing brokers’ capabilities in scientific discovery, highlighting the necessity for improved basic AI discovery brokers.
Try the Paper. All credit score for this analysis goes to the researchers of this challenge. Additionally, don’t overlook to observe us on Twitter and be part of our Telegram Channel and LinkedIn Group. Should you like our work, you’ll love our e-newsletter.. Don’t Neglect to affix our 50k+ ML SubReddit
[Upcoming Event- Oct 17 202] RetrieveX – The GenAI Information Retrieval Convention (Promoted)
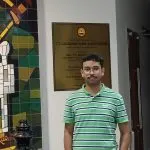
Sana Hassan, a consulting intern at Marktechpost and dual-degree pupil at IIT Madras, is keen about making use of know-how and AI to handle real-world challenges. With a eager curiosity in fixing sensible issues, he brings a recent perspective to the intersection of AI and real-life options.