Whereas multimodal fashions (LMMs) have superior considerably for textual content and picture duties, video-based fashions stay underdeveloped. Movies are inherently complicated, combining spatial and temporal dimensions that demand extra from computational sources. Current strategies usually adapt image-based approaches immediately or depend on uniform body sampling, which poorly captures movement and temporal patterns. Furthermore, coaching large-scale video fashions is computationally costly, making it troublesome to discover design selections effectively.
To deal with these points, researchers from Meta AI and Stanford developed Apollo, a household of video-focused LMMs designed to push the boundaries of video understanding. Apollo addresses these challenges by considerate design choices, bettering effectivity, and setting a brand new benchmark for duties like temporal reasoning and video-based query answering.
Meta AI Introduces Apollo: A Household of Scalable Video-LMMs
Meta AI’s Apollo fashions are designed to course of movies as much as an hour lengthy whereas reaching robust efficiency throughout key video-language duties. Apollo is available in three sizes – 1.5B, 3B, and 7B parameters – providing flexibility to accommodate numerous computational constraints and real-world wants.
Key improvements embrace:
- Scaling Consistency: Design selections made on smaller fashions are proven to switch successfully to bigger ones, lowering the necessity for large-scale experiments.
- Body-Per-Second (fps) Sampling: A extra environment friendly video sampling approach in comparison with uniform body sampling, guaranteeing higher temporal consistency.
- Twin Imaginative and prescient Encoders: Combining SigLIP for spatial understanding with InternVideo2 for temporal reasoning permits a balanced illustration of video knowledge.
- ApolloBench: A curated benchmark suite that reduces redundancy in analysis whereas offering detailed insights into mannequin efficiency.

Technical Highlights and Benefits
The Apollo fashions are constructed on a collection of well-researched design selections aimed toward overcoming the challenges of video-based LMMs:
- Body-Per-Second Sampling: In contrast to uniform body sampling, fps sampling maintains a constant temporal circulation, permitting Apollo to raised perceive movement, velocity, and sequence of occasions in movies.
- Scaling Consistency: Experiments present that mannequin design selections made on reasonably sized fashions (2B-4B parameters) generalize properly to bigger fashions. This method reduces computational prices whereas sustaining efficiency beneficial properties.
- Twin Imaginative and prescient Encoders: Apollo makes use of two complementary encoders: SigLIP, which excels at spatial understanding, and InternVideo2, which boosts temporal reasoning. Their mixed strengths produce extra correct video representations.
- Token Resampling: By utilizing a Perceiver Resampler, Apollo effectively reduces video tokens with out dropping info. This permits the fashions to course of lengthy movies with out extreme computational overhead.
- Optimized Coaching: Apollo employs a three-stage coaching course of the place video encoders are initially fine-tuned on video knowledge earlier than integrating with textual content and picture datasets. This staged method ensures steady and efficient studying.
- Multi-Flip Conversations: Apollo fashions can help interactive, multi-turn conversations grounded in video content material, making them supreme for functions like video-based chat techniques or content material evaluation.
Efficiency Insights
Apollo’s capabilities are validated by robust outcomes on a number of benchmarks, usually outperforming bigger fashions:
- Apollo-1.5B:
- Surpasses fashions like Phi-3.5-Imaginative and prescient (4.2B) and LongVA-7B.
- Scores: 60.8 on Video-MME, 63.3 on MLVU, 57.0 on ApolloBench.
- Apollo-3B:
- Competes with and outperforms many 7B fashions.
- Scores: 58.4 on Video-MME, 68.7 on MLVU, 62.7 on ApolloBench.
- Achieves 55.1 on LongVideoBench.
- Apollo-7B:
- Matches and even surpasses fashions with over 30B parameters, reminiscent of Oryx-34B and VILA1.5-40B.
- Scores: 61.2 on Video-MME, 70.9 on MLVU, 66.3 on ApolloBench.
Benchmark Abstract:

Conclusion
Apollo marks a major step ahead in video-LMM improvement. By addressing key challenges reminiscent of environment friendly video sampling and mannequin scalability, Apollo offers a sensible and highly effective resolution for understanding video content material. Its potential to outperform bigger fashions highlights the significance of well-researched design and coaching methods.
The Apollo household gives sensible options for real-world functions, from video-based query answering to content material evaluation and interactive techniques. Importantly, Meta AI’s introduction of ApolloBench offers a extra streamlined and efficient benchmark for evaluating video-LMMs, paving the best way for future analysis.
Try the Paper, Web site, Demo, Code, and Fashions. All credit score for this analysis goes to the researchers of this challenge. Additionally, don’t neglect to observe us on Twitter and be a part of our Telegram Channel and LinkedIn Group. Don’t Overlook to hitch our 60k+ ML SubReddit.
🚨 Trending: LG AI Analysis Releases EXAONE 3.5: Three Open-Supply Bilingual Frontier AI-level Fashions Delivering Unmatched Instruction Following and Lengthy Context Understanding for World Management in Generative AI Excellence….
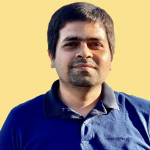
Asif Razzaq is the CEO of Marktechpost Media Inc.. As a visionary entrepreneur and engineer, Asif is dedicated to harnessing the potential of Synthetic Intelligence for social good. His most up-to-date endeavor is the launch of an Synthetic Intelligence Media Platform, Marktechpost, which stands out for its in-depth protection of machine studying and deep studying information that’s each technically sound and simply comprehensible by a large viewers. The platform boasts of over 2 million month-to-month views, illustrating its reputation amongst audiences.