Understanding social interactions in advanced real-world settings requires deep psychological reasoning to deduce the underlying psychological states driving these interactions, referred to as the Concept of Thoughts (ToM). Social interactions are sometimes multi-modal, involving actions, conversations, and previous behaviors. For AI to successfully interact in human environments, it should grasp these psychological states and their interrelations. Regardless of advances in machine ToM, present benchmarks primarily deal with particular person psychological states and lack multi-modal datasets for evaluating multi-agent ToM. This hole hinders the event of AI programs able to understanding nuanced social interactions, which is essential for secure human-AI interplay.
Researchers from Johns Hopkins College and the College of Virginia launched MuMA-ToM, the primary benchmark to evaluate multi-modal, multi-agent ToM reasoning in embodied interactions. MuMA-ToM presents movies and textual content describing real-life eventualities and poses questions on brokers’ objectives and beliefs about others’ objectives. They validated MuMA-ToM via human experiments and launched LIMP (Language model-based Inverse Multi-agent Planning), a novel ToM mannequin. LIMP outperformed current fashions, together with GPT-4o and BIP-ALM, by integrating two-level reasoning and eliminating the necessity for symbolic representations. The work highlights the hole between human and machine ToM.
ToM benchmarks historically deal with single-agent reasoning, whereas multi-agent benchmarks typically lack questions on inter-agent relationships. Current ToM benchmarks normally depend on textual content or video, with few exceptions like MMToM-QA, which addresses single-agent actions in a multi-modal format. MuMA-ToM, nevertheless, introduces a benchmark for multi-agent ToM reasoning utilizing textual content and video to depict lifelike interactions. In contrast to earlier strategies like BIP-ALM, which requires symbolic representations, the LIMP mannequin enhances multi-agent planning and employs basic, domain-invariant representations, bettering ToM reasoning in multi-modal, multi-agent contexts.
The MuMA-ToM Benchmark evaluates fashions for understanding multi-agent social interactions utilizing video and textual content. It options 225 interactions and 900 questions targeted on three ToM ideas: perception inference, social objective inference, and perception of objective inference. The interactions are procedurally generated with distinct multimodal inputs, difficult fashions to successfully fuse this data. Based mostly on the I-POMDP framework, the benchmark employs LIMP, which integrates vision-language and language fashions to deduce psychological states. Human accuracy is excessive, however even high fashions like Gemini 1.5 Professional and Llava 1.6 have to catch up.
In experiments, 18 contributors from Prolific answered 90 randomly chosen questions from the MuMA-ToM benchmark, reaching a excessive accuracy fee of 93.5%. State-of-the-art fashions, together with Gemini 1.5 Professional and Llava 1.6, carried out considerably worse, with the very best mannequin accuracy at 56.4%. The LIMP mannequin outperformed others with a 76.6% accuracy by successfully integrating multimodal inputs and utilizing pure language for motion inference. Nevertheless, LIMP’s limitations embrace susceptibility to visible hallucinations and lack of express multi-level reasoning. The benchmark is at present restricted to two-agent interactions in artificial family settings.
In conclusion, MuMA-ToM is the primary multimodal Concept of Thoughts benchmark for evaluating psychological reasoning in advanced multi-agent interactions. MuMA-ToM makes use of video and textual content inputs to evaluate understanding of objectives and beliefs in lifelike family settings. The examine systematically evaluated human efficiency and examined state-of-the-art fashions, proposing a mannequin LIMP (Language model-based Inverse Multi-agent Planning). LIMP outperformed current fashions, together with GPT-4o and Gemini-1.5 Professional. Future work will prolong the benchmark to extra advanced real-world eventualities, together with interactions involving a number of brokers and real-world movies.
Try the Paper. All credit score for this analysis goes to the researchers of this venture. Additionally, don’t neglect to observe us on Twitter and LinkedIn. Be a part of our Telegram Channel. For those who like our work, you’ll love our e-newsletter..
Don’t Overlook to hitch our 50k+ ML SubReddit
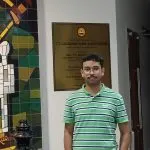
Sana Hassan, a consulting intern at Marktechpost and dual-degree scholar at IIT Madras, is captivated with making use of know-how and AI to handle real-world challenges. With a eager curiosity in fixing sensible issues, he brings a recent perspective to the intersection of AI and real-life options.