Edge AI has lengthy confronted the problem of balancing effectivity and effectiveness. Deploying Imaginative and prescient Language Fashions (VLMs) on edge units is tough because of their massive measurement, excessive computational calls for, and latency points. Fashions designed for cloud environments typically wrestle with the restricted assets of edge units, leading to extreme battery utilization, slower response instances, and inconsistent connectivity. The demand for light-weight but environment friendly fashions has been rising, pushed by purposes akin to augmented actuality, good dwelling assistants, and industrial IoT, which require speedy processing of visible and textual inputs. These challenges are additional sophisticated by elevated hallucination charges and unreliable ends in duties like visible query answering or picture captioning, the place high quality and accuracy are important.
Nexa AI Releases OmniVision-968M: World’s Smallest Imaginative and prescient Language Mannequin with 9x Tokens Discount for Edge Units. OmniVision-968M has been engineered with improved structure over LLaVA (Massive Language and Imaginative and prescient Assistant), reaching a brand new stage of compactness and effectivity, ultimate for working on the sting. With a design targeted on the discount of picture tokens by an element of 9—from 729 to only 81—the latency and computational burden sometimes related to such fashions have been drastically minimized.
OmniVision’s structure is constructed round three principal elements:
- Base Language Mannequin: Qwen2.5-0.5B-Instruct serves because the core mannequin for processing textual content inputs.
- Imaginative and prescient Encoder: SigLIP-400M, with a 384 decision and 14×14 patch measurement, generates picture embeddings.
- Projection Layer: A Multi-Layer Perceptron (MLP) aligns the imaginative and prescient encoder’s embeddings with the token house of the language mannequin. In contrast to the usual Llava structure, our projector reduces the variety of picture tokens by 9 instances.
OmniVision-968M integrates a number of key technical developments that make it an ideal match for edge deployment. The mannequin’s structure has been enhanced based mostly on LLaVA, permitting it to course of each visible and textual content inputs with excessive effectivity. The picture token discount from 729 to 81 represents a major leap in optimization, making it nearly 9 instances extra environment friendly in token processing in comparison with present fashions. This has a profound affect on decreasing latency and computational prices, that are essential components for edge units. Moreover, OmniVision-968M leverages Direct Desire Optimization (DPO) coaching with reliable knowledge sources, which helps mitigate the issue of hallucination—a standard problem in multimodal AI methods. By specializing in visible query answering and picture captioning, the mannequin goals to supply a seamless, correct consumer expertise, making certain reliability and robustness in edge purposes the place real-time response and energy effectivity are essential.


The discharge of OmniVision-968M represents a notable development for a number of causes. Primarily, the discount in token rely considerably decreases the computational assets required for inference. For builders and enterprises trying to implement VLMs in constrained environments—akin to wearables, cell units, and IoT {hardware}—the compact measurement and effectivity of OmniVision-968M make it a super resolution. Moreover, the DPO coaching technique helps reduce hallucination, a standard subject the place fashions generate incorrect or deceptive info, making certain that OmniVision-968M is each environment friendly and dependable. Preliminary benchmarks point out that OmniVision-968M achieves a 35% discount in inference time in comparison with earlier fashions whereas sustaining and even enhancing accuracy in duties like visible query answering and picture captioning. These developments are anticipated to encourage adoption throughout industries that require high-speed, low-power AI interactions, akin to healthcare, good cities, and the automotive sector.
In conclusion, Nexa AI’s OmniVision-968M addresses a long-standing hole within the AI trade: the necessity for extremely environment friendly imaginative and prescient language fashions that may run seamlessly on edge units. By decreasing picture tokens, optimizing LLaVA’s structure, and incorporating DPO coaching to make sure reliable outputs, OmniVision-968M represents a brand new frontier in edge AI. This mannequin brings us nearer to the imaginative and prescient of ubiquitous AI—the place good, related units can carry out refined multimodal duties regionally with out the necessity for fixed cloud assist.
Try the Mannequin on Hugging Face and Different Particulars. All credit score for this analysis goes to the researchers of this venture. Additionally, don’t overlook to observe us on Twitter and be a part of our Telegram Channel and LinkedIn Group. For those who like our work, you’ll love our publication.. Don’t Overlook to affix our 55k+ ML SubReddit.
[FREE AI WEBINAR] Implementing Clever Doc Processing with GenAI in Monetary Companies and Actual Property Transactions
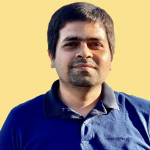
Asif Razzaq is the CEO of Marktechpost Media Inc.. As a visionary entrepreneur and engineer, Asif is dedicated to harnessing the potential of Synthetic Intelligence for social good. His most up-to-date endeavor is the launch of an Synthetic Intelligence Media Platform, Marktechpost, which stands out for its in-depth protection of machine studying and deep studying information that’s each technically sound and simply comprehensible by a large viewers. The platform boasts of over 2 million month-to-month views, illustrating its recognition amongst audiences.