Delays or errors in diagnosing pneumoperitoneum, with air outdoors the intestines throughout the peritoneal cavity, can severely influence affected person survival and well being outcomes. In adults, most instances outcome from a perforated viscus, with as much as 90% needing surgical intervention. Whereas CT scans are the popular diagnostic instrument for his or her excessive accuracy, interpretation delays are frequent in busy emergency departments. Variability in diagnostic confidence and interpretation between residents and attending radiologists can contribute to delays and misdiagnoses. AI has proven potential in bettering pace and accuracy in medical imaging evaluation, although its effectiveness for pneumoperitoneum detection is dependent upon cautious dataset choice and ongoing refinement.
Researchers from Cedars-Sinai Medical Middle and Far Japanese Memorial Hospital developed a deep-learning mannequin to detect pneumoperitoneum in CT pictures. Skilled on information from Far Japanese Memorial Hospital and validated on latest scans, the mannequin achieved excessive sensitivity (0.81–0.83) and specificity (0.97–0.99) throughout retrospective, potential, and exterior datasets, with sensitivity bettering to 0.92–0.98 when excluding instances with minimal free air. Moreover, they launched PACT-3D, a 3D UNet-based mannequin optimized for pneumoperitoneum segmentation. PACT-3D captures spatial particulars throughout a number of planes, providing correct, patient-level predictions with pixel-level segmentation, demonstrating sturdy efficiency in simulated and real-world situations.
The research adhered to the STARD pointers and was authorised by Institutional Overview Boards at Far Japanese Memorial Hospital (IRB 111086-F) and Cedars-Sinai Medical Middle (IRB STUDY00003494). The mannequin operated with out altering commonplace affected person care throughout the potential analysis part. Information collected throughout this era, together with mannequin predictions, have been later extracted for evaluation. The dataset included contrast-enhanced belly CT scans from Far Japanese Memorial Hospital, gathered between 2012 and 2021. Every scan was reviewed to substantiate the presence or absence of pneumoperitoneum, with two radiologists verifying optimistic instances. Actual-world mannequin efficiency was assessed from December 2022 to Could 2023 utilizing potential information from the identical facility.
Information acquisition concerned CT scans containing distinction, scanned within the axial aircraft with a 5 mm slice thickness and belly protection. NLP strategies have been employed to determine related stories, and take a look at units with a 5:1:1 ratio, avoiding information duplication throughout units. Handbook annotations of pneumoperitoneum have been accomplished by skilled radiologists, adopted by a secondary evaluation. A 3D U-Internet mannequin was developed for segmentation, integrating a contracting path for contextual understanding and an increasing path for detailed localization. Information augmentation and a mixed Cube and Focal loss perform have been used to deal with class imbalance, with coaching performed on an Nvidia RTX A6000 GPU. An adaptive second estimation (Adam) optimizer and a cosine annealing studying fee scheduler enhanced mannequin coaching stability and convergence.
The research analyzed 139,781 belly CT scans, with 973 exhibiting pneumoperitoneum, divided into coaching, validation, and take a look at units at a 5:1:1 ratio. The mannequin’s efficiency was validated with a potential set of 6,351 scans from December 2022 to Could 2023. The 3D U-Internet mannequin confirmed an F1-score of 0.54 within the simulated set and 0.58 within the potential set, with excessive sensitivity and specificity. In exterior validation at Cedars-Sinai Medical Middle, it achieved an F1-score of 0.80. Sensitivity elevated with larger free air volumes, and optimistic predictions correlated with the next fee of pressing surgical procedures.
The research presents PACT-3D, a 3D U-Internet-based deep studying mannequin designed to detect pneumoperitoneum in belly CT scans. Regardless of various scanner fashions and geographical variations, PACT-3D confirmed sturdy efficiency throughout numerous take a look at units, sustaining excessive sensitivity and specificity. The mannequin’s 3D structure permits improved differentiation of free air from bowel fuel, supporting dependable detection, particularly in vital instances needing instant intervention. Though efficient, the mannequin requires refinement to reinforce sensitivity for smaller volumes of free air. PACT-3D demonstrates sturdy potential to enhance diagnostic effectivity in emergency care, doubtlessly enhancing medical outcomes.
Take a look at the Paper. All credit score for this analysis goes to the researchers of this mission. Additionally, don’t neglect to observe us on Twitter and be a part of our Telegram Channel and LinkedIn Group. When you like our work, you’ll love our e-newsletter.. Don’t Neglect to affix our 55k+ ML SubReddit.
[AI Magazine/Report] Learn Our Newest Report on ‘SMALL LANGUAGE MODELS‘
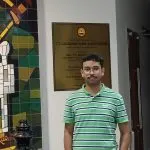
Sana Hassan, a consulting intern at Marktechpost and dual-degree pupil at IIT Madras, is obsessed with making use of expertise and AI to deal with real-world challenges. With a eager curiosity in fixing sensible issues, he brings a contemporary perspective to the intersection of AI and real-life options.