Drug-induced toxicity is a serious problem in drug improvement, contributing considerably to the failure of medical trials. Whereas efficacy points account for many failures, security issues are the second main trigger, at 24%. Toxicities can have an effect on numerous organ methods, together with the center, liver, kidneys, and lungs, and even accepted medication might face withdrawal on account of unexpected poisonous results in post-market surveillance. Present toxicity datasets, usually derived from labor-intensive professional analyses of FDA drug labels, are sometimes small and restricted to particular organ methods. These paperwork, which element a drug’s indications, dangers, and medical trial outcomes, are essential however time-consuming to curate, usually exceeding 100 pages per drug. Consequently, there’s a urgent want for predictive fashions to determine safer drug candidates early in improvement.
Efforts to construct complete toxicity datasets have confronted a number of limitations. Present databases, akin to SIDER, LiverTox, and PNEUMOTOX, are sometimes organ-specific or depend on in vitro assays, which can not precisely predict in vivo results. Annotation efforts are time-intensive, and methodologies for toxicity analysis differ broadly, resulting in inconsistencies throughout datasets. As an example, the FDA’s renal toxicity database, DIRIL, integrates conflicting sources with over 30% disagreement on sure medication. Giant language fashions (LLMs) like askFDALabel supply promise by streamlining information extraction from FDA labels, reaching as much as 78% settlement with human evaluations for cardiotoxicity. Nonetheless, regardless of developments, challenges in dataset scalability, annotation consistency, and complete protection persist, limiting the effectiveness of ML fashions skilled on these datasets.
Researchers from Stanford College and Genmab launched UniTox, a complete dataset of two,418 FDA-approved medication, summarizing and ranking drug-induced toxicities utilizing GPT-4o to course of FDA drug labels. Overlaying eight toxicity varieties, together with cardiotoxicity, liver toxicity, and infertility, UniTox is the most important systematic in vivo database and the primary to embody almost all non-combination FDA-approved medication for these toxicities. Clinicians validated a subset of GPT-4o annotations, with concordance charges of 85–96%. Benchmarks of machine studying fashions skilled on UniTox demonstrated its utility for predicting molecular toxicity, reaching as much as 93% accuracy on present datasets and surpassing askFDALabel’s efficiency.
To develop UniTox, researchers curated a dataset of two,418 FDA-approved medication by filtering and deduplicating drug labels from the FDALabel database, together with biologics. Utilizing GPT-4o and a two-step chain-of-thought prompting system, the group generated toxicity summaries and rankings for eight toxicity varieties. The mannequin categorized toxicity utilizing ternary (No, Much less, Most) and binary (Sure, No) scales. The validation included comparisons with present FDA datasets (DICTrank, DILIrank, DIRIL) and clinician opinions, reaching robust concordance. Clinicians evaluated a subset for toxicity varieties missing prior information, scoring the mannequin’s outputs primarily based on factual accuracy and alignment with professional information.
The UniTox dataset, encompassing 2,418 medication and eight toxicity varieties, provides a complete useful resource for toxicity evaluation. It consists of GPT-4o-generated toxicity summaries, ternary and binary classifications, and Structured Product Labeling (SPL) IDs. Summaries condense prolonged drug labels into 297 phrases on common, aiding fast comprehension and enabling their use as floor fact for coaching toxicity predictors. The dataset reveals toxicity correlations, with liver and hematological toxicity displaying the very best relationship (0.45). UniTox additionally offers insights into toxicity patterns throughout drug lessons primarily based on WHO-ATC classifications, highlighting variations linked to FDA danger tolerance for various therapeutic classes.
In conclusion, the examine highlights using GPT-4o for effectively summarizing complicated drug labels, producing correct toxicity rankings throughout eight varieties, together with liver, renal, and cardiotoxicity. These rankings confirmed robust settlement with datasets like DILIrank and medical reviewers, enabling coaching molecular classifiers with predictive worth. The UniTox dataset, comprising 2,418 FDA-approved medication, is the most important and bridges gaps in toxicity analysis throughout a number of organ methods. Regardless of challenges like nuanced toxicity translation and restricted applicability to failed medication, UniTox demonstrates the worth of LLMs in creating detailed datasets, advancing drug toxicity prediction, and supporting future analysis efforts.
Take a look at the Paper and Dataset. All credit score for this analysis goes to the researchers of this venture. Additionally, don’t overlook to observe us on Twitter and be part of our Telegram Channel and LinkedIn Group. Don’t Overlook to hitch our 60k+ ML SubReddit.
🚨 Trending: LG AI Analysis Releases EXAONE 3.5: Three Open-Supply Bilingual Frontier AI-level Fashions Delivering Unmatched Instruction Following and Lengthy Context Understanding for World Management in Generative AI Excellence….
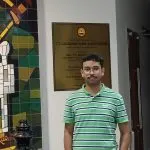
Sana Hassan, a consulting intern at Marktechpost and dual-degree pupil at IIT Madras, is obsessed with making use of expertise and AI to deal with real-world challenges. With a eager curiosity in fixing sensible issues, he brings a contemporary perspective to the intersection of AI and real-life options.