Predibase publicizes the Predibase Inference Engine, their new infrastructure providing designed to be the most effective platform for serving fine-tuned small language fashions (SLMs). The Predibase Inference Engine dramatically improves SLM deployments by making them quicker, simply scalable, and less expensive for enterprises grappling with the complexities of productionizing AI. Constructed on Predibase’s improvements–Turbo LoRA and LoRA eXchange (LoRAX)–the Predibase Inference Engine is designed from the bottom as much as provide a best-in-class expertise for serving fine-tuned SLMs.
The necessity for such an innovation is obvious. As AI turns into extra entrenched within the material of enterprise operations, the challenges related to deploying and scaling SLMs have grown more and more daunting. Homegrown infrastructure is usually ill-equipped to deal with the dynamic calls for of high-volume AI workloads, resulting in inflated prices, diminished efficiency, and operational bottlenecks. The Predibase Inference Engine addresses these challenges head-on, providing a tailored resolution for enterprise AI deployments.
Be a part of Predibase webinar on October twenty ninth to be taught extra concerning the Predibase Inference Engine!
The Key Challenges in Deploying LLMs at Scale
As companies proceed to combine AI into their core operations and have to show ROI, the demand for environment friendly, scalable options has skyrocketed. The deployment of LLMs, and fine-tuned SLMs particularly, has turn into a important part of profitable AI initiatives however presents vital challenges at scale:
- Efficiency Bottlenecks: Most cloud suppliers’ entry-level GPUs wrestle with manufacturing use circumstances, particularly these with spiky or variable workloads, leading to sluggish response occasions and a diminished buyer expertise. Moreover, scaling LLM deployments to fulfill peak demand with out incurring prohibitive prices or efficiency degradation is a major problem as a result of lack of GPU autoscaling capabilities in lots of cloud environments.
- Engineering Complexity: Adopting open-source fashions for manufacturing use requires enterprises to handle the whole serving infrastructure themselves—a high-stakes, resource-intensive proposition. This provides vital engineering complexity, demanding specialised experience and forcing groups to commit substantial assets to make sure dependable efficiency and scalability in manufacturing environments.
- Excessive Infrastructure Prices: Excessive-performing GPUs just like the NVIDIA H100 and A100 are in excessive demand and sometimes have restricted availability from cloud suppliers, resulting in potential shortages. These GPUs are usually provided in “always-on” deployment fashions, which guarantee availability however may be pricey because of steady billing, no matter precise utilization.
These challenges underscore the necessity for an answer just like the Predibase Inference Engine, which is designed to streamline the deployment course of and supply a scalable, cost-effective infrastructure for managing SLMs.
Technical Breakthroughs within the Predibase Inference Engine
On the coronary heart of the Predibase Inference Engine are a set of revolutionary options that collectively improve the deployment of SLMs:
- LoRAX: LoRA eXchange (LoRAX) permits for the serving of tons of of fine-tuned SLMs from a single GPU. This functionality considerably reduces infrastructure prices by minimizing the variety of GPUs wanted for deployment. It’s significantly helpful for companies that have to deploy varied specialised fashions with out the overhead of dedicating a GPU to every mannequin. Be taught extra.
- Turbo LoRA: Turbo LoRA is our parameter-efficient fine-tuning technique that accelerates throughput by 2-3 occasions whereas rivaling or exceeding GPT-4 by way of response high quality. These throughput enhancements significantly scale back inference prices and latency, even for high-volume use circumstances.
- FP8 Quantization: Implementing FP8 quantization can scale back the reminiscence footprint of deploying a fine-tuned SLM by 50%, main to almost 2x additional enhancements in throughput. This optimization not solely improves efficiency but additionally enhances the cost-efficiency of deployments, permitting for as much as 2x extra simultaneous requests on the identical variety of GPUs.
- GPU Autoscaling: Predibase SaaS deployments can dynamically regulate GPU assets based mostly on real-time demand. This flexibility ensures that assets are effectively utilized, decreasing waste and value during times of fluctuating demand.
These technical improvements are essential for enterprises seeking to deploy AI options which can be each highly effective and economical. By addressing the core challenges related to conventional mannequin serving, the Predibase Inference Engine units a brand new customary for effectivity and scalability in AI deployments.
LoRA eXchange: Scale 100+ Nice-Tuned LLMs Effectively on a Single GPU
LoRAX is a cutting-edge serving infrastructure designed to deal with the challenges of deploying a number of fine-tuned SLMs effectively. Not like conventional strategies that require every fine-tuned mannequin to run on devoted GPU assets, LoRAX permits organizations to serve tons of of fine-tuned SLMs on a single GPU, drastically decreasing prices. By using dynamic adapter loading, tiered weight caching, and multi-adapter batching, LoRAX optimizes GPU reminiscence utilization and maintains excessive throughput for concurrent requests. This revolutionary infrastructure permits cost-effective deployment of fine-tuned SLMs, making it simpler for enterprises to scale AI fashions specialised to their distinctive duties.
Get extra out of your GPU: 4x velocity enhancements for SLMs with Turbo LoRA and FP8
Optimizing SLM inference is essential for scaling AI deployments, and two key strategies are driving main throughput efficiency positive aspects. Turbo LoRA boosts throughput by 2-3x by means of speculative decoding, making it attainable to foretell a number of tokens in a single step with out sacrificing output high quality. Moreover, FP8 quantization additional will increase GPU throughput, enabling a lot more economical deployments when utilizing fashionable {hardware} like NVIDIA L40S GPUs.
Turbo LoRA Will increase Throughput by 2-3x
Turbo LoRA combines Low Rank Adaptation (LoRA) and speculative decoding to boost the efficiency of SLM inference. LoRA improves response high quality by including new parameters tailor-made to particular duties, however it usually slows down token technology as a result of further computational steps. Turbo LoRA addresses this by enabling the mannequin to foretell a number of tokens in a single step, considerably growing throughput by 2-3 occasions in comparison with base fashions with out compromising output high quality.
Turbo LoRA is especially efficient as a result of it adapts to all varieties of GPUs, together with high-performing fashions like H100s and entry degree fashions just like the A10g. This common compatibility ensures that organizations can deploy Turbo LoRA throughout completely different {hardware} setups (whether or not in Predibase’s cloud or their VPC setting) while not having particular changes for every GPU kind. This makes Turbo LoRA an economical resolution for enhancing the efficiency of SLMs throughout a variety of computing environments.
As well as, Turbo LoRA achieves these advantages all by means of a single mannequin whereas the vast majority of speculative decoding implementations use a draft mannequin along with their fundamental mannequin. This additional reduces the GPU necessities and community overhead.
Additional Improve Throughput with FP8
FP8 quantization is a way that reduces the precision of a mannequin’s information format from a normal floating-point illustration, akin to FP16, to an 8-bit floating-point format. This compression reduces the mannequin’s reminiscence footprint by as much as 50%, permitting it to course of information extra effectively and growing throughput on GPUs. The smaller measurement signifies that much less reminiscence is required to retailer weights and carry out matrix multiplications, which consequently can practically double the throughput of a given GPU.
Past simply efficiency enhancements, FP8 quantization additionally impacts the cost-efficiency of deploying SLMs. By growing the variety of concurrent requests a GPU can deal with, organizations can meet their efficiency SLAs with fewer compute assets. Whereas solely the newest technology of NVIDIA GPUs help FP8, making use of FP8 to L40S GPUs–now extra available in Amazon EC2–will increase throughput to outperform an A100 GPU whereas costing roughly 33% much less.
Optimized GPU Scaling for Efficiency and Value Effectivity
GPU autoscaling is a important function for managing AI workloads, guaranteeing that assets are dynamically adjusted based mostly on real-time demand. Our Inference Engine’s capacity to scale GPU assets as wanted helps enterprises optimize utilization, decreasing prices by solely scaling up when demand will increase and cutting down throughout quieter intervals. This flexibility permits organizations to take care of high-performance AI operations with out over-provisioning assets.
For purposes that require constant efficiency, our platform provides the choice to order GPU capability, guaranteeing availability throughout peak hundreds. That is significantly precious to be used circumstances the place response occasions are essential, guaranteeing that even throughout visitors spikes, AI fashions carry out with out interruptions or delays. Reserved capability ensures enterprises meet their efficiency SLAs with out pointless over-allocation of assets.
Moreover, the Inference Engine minimizes chilly begin occasions by quickly scaling assets, decreasing delays in startup and guaranteeing fast changes to sudden will increase in visitors. This function enhances the responsiveness of the system, permitting organizations to deal with unpredictable visitors surges effectively and with out compromising on efficiency.
Along with optimizing efficiency, GPU autoscaling considerably reduces deployment prices. Not like conventional “always-on” GPU deployments, which incur steady bills no matter precise utilization, autoscaling ensures assets are allotted solely when wanted. Within the instance above, a normal always-on deployment for an enterprise workload would price over $213,000 per yr, whereas an autoscaling deployment reduces that to lower than $155,000 yearly—providing a financial savings of practically 30%. (It’s necessary to notice that each deployment configurations price lower than half as a lot as utilizing fine-tuned GPT-4o-mini.) By dynamically adjusting GPU assets based mostly on real-time demand, enterprises can obtain excessive efficiency with out the burden of overpaying for idle infrastructure, making AI deployments far less expensive.
Enterprise readiness
Designing AI infrastructure for enterprise purposes is complicated, with many important particulars to handle in case you’re constructing your individual. From safety compliance to making sure excessive availability throughout areas, enterprise-scale deployments require cautious planning. Groups should steadiness efficiency, scalability, and cost-efficiency whereas integrating with current IT techniques.
Predibase’s Inference Engine simplifies this by providing enterprise-ready options that handle these challenges, together with VPC integration, multi-region excessive availability, and real-time deployment insights. These options assist enterprises like Convirza deploy and handle AI workloads at scale with out the operational burden of constructing and sustaining infrastructure themselves.
“At Convirza, our workload may be extraordinarily variable, with spikes that require scaling as much as double-digit A100 GPUs to take care of efficiency. The Predibase Inference Engine and LoRAX permit us to effectively serve 60 adapters whereas constantly attaining a mean response time of below two seconds,” mentioned Giuseppe Romagnuolo, VP of AI at Convirza. “Predibase offers the reliability we’d like for these high-volume workloads. The considered constructing and sustaining this infrastructure on our personal is daunting—fortunately, with Predibase, we don’t should.”
Our cloud or yours: Digital Personal Clouds
The Predibase Inference Engine is offered in our cloud or yours. Enterprises can select between deploying inside their very own personal cloud infrastructure or using Predibase’s absolutely managed SaaS platform. This flexibility ensures seamless integration with current enterprise IT insurance policies, safety protocols, and compliance necessities. Whether or not corporations favor to maintain their information and fashions totally inside their Digital Personal Cloud (VPC) for enhanced safety and to benefit from cloud supplier spend commitments or leverage Predibase’s SaaS for added flexibility, the platform adapts to fulfill numerous enterprise wants.
Multi-Area Excessive Availability
The Inference Engine’s multi-region deployment function ensures that enterprises can preserve uninterrupted service, even within the occasion of regional outages or disruptions. Within the occasion of a disruption, the platform routinely reroutes visitors to a functioning area and spins up further GPUs to deal with the elevated demand. This fast scaling of assets minimizes downtime and ensures that enterprises can preserve their service-level agreements (SLAs) with out compromising efficiency or reliability.
By dynamically provisioning further GPUs within the failover area, the Inference Engine offers speedy capability to help important AI workloads, permitting companies to proceed working easily even within the face of surprising failures. This mix of multi-region redundancy and autoscaling ensures that enterprises can ship constant, high-performance providers to their customers, regardless of the circumstances.
Maximizing Effectivity with Actual-Time Deployment Insights
Along with the Inference Engine’s highly effective autoscaling and multi-region capabilities, Predibase’s Deployment Well being Analytics present important real-time insights for monitoring and optimizing your deployments. This instrument tracks important metrics like request quantity, throughput, GPU utilization, and queue length, supplying you with a complete view of how nicely your infrastructure is performing. Through the use of these insights, enterprises can simply steadiness efficiency with price effectivity, scaling GPU assets up or down as wanted to fulfill fluctuating demand whereas avoiding over-provisioning.
With customizable autoscaling thresholds, Deployment Well being Analytics lets you fine-tune your technique based mostly on particular operational wants. Whether or not it’s guaranteeing that GPUs are effectively utilized throughout visitors spikes or cutting down assets to attenuate prices, these analytics empower companies to take care of high-performance deployments that run easily always. For extra particulars on optimizing your deployment technique, try the full weblog publish.
Why Select Predibase?
Predibase is the main platform for enterprises serving fine-tuned LLMs, providing unmatched infrastructure designed to fulfill the precise wants of contemporary AI workloads. Our Inference Engine is constructed for max efficiency, scalability, and safety, guaranteeing enterprises can deploy fine-tuned fashions with confidence. With built-in compliance and a concentrate on cost-effective, dependable mannequin serving, Predibase is the best choice for corporations seeking to serve fine-tuned LLMs at scale whereas sustaining enterprise-grade safety and effectivity.
Should you’re able to take your LLM deployments to the following degree, go to Predibase.com to be taught extra concerning the Predibase Inference Engine, or attempt it without spending a dime to see firsthand how our options can remodel your AI operations.
Because of the Predibase staff for the thought management/ Sources for this text. The Predibase AI staff has supported us on this content material/article.
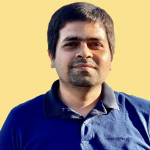
Asif Razzaq is the CEO of Marktechpost Media Inc.. As a visionary entrepreneur and engineer, Asif is dedicated to harnessing the potential of Synthetic Intelligence for social good. His most up-to-date endeavor is the launch of an Synthetic Intelligence Media Platform, Marktechpost, which stands out for its in-depth protection of machine studying and deep studying information that’s each technically sound and simply comprehensible by a large viewers. The platform boasts of over 2 million month-to-month views, illustrating its recognition amongst audiences.