Predicting RNA 3D constructions is important for understanding its organic features, advancing RNA-targeted drug discovery, and designing artificial biology purposes. Nevertheless, RNA’s structural flexibility and the restricted availability of experimentally resolved information pose challenges. Regardless of RNA’s significance in gene regulation, RNA-only constructions symbolize lower than 1% of the Knowledge Financial institution, and conventional strategies like X-ray crystallography and cryo-EM are gradual and resource-intensive. Computational strategies, together with template-based strategies like ModeRNA and de novo approaches like FARFAR2, have superior RNA modeling however typically want extra pace and information availability. Deep studying fashions have emerged as transformative instruments by leveraging RNA sequence information.
Current deep learning-based strategies combine a number of sequence alignments (MSAs) and secondary construction constraints to reinforce RNA 3D construction prediction. Approaches like DeepFoldRNA and trRosettaRNA use MSAs to derive geometric options for energy-based modeling, whereas end-to-end frameworks like AlphaFold3 and RoseTTAFoldNA instantly predict 3D constructions from sequences. Though MSA-based strategies provide excessive accuracy, they’re computationally costly attributable to intensive database searches. Alternate options like DRFold rely solely on single sequences, offering quicker outcomes with barely decrease precision. Future developments purpose to mix the pace of single-sequence fashions with the accuracy of MSA-based strategies for extra environment friendly predictions.
RhoFold+ is a complicated deep studying framework developed by researchers from establishments together with The Chinese language College of Hong Kong, Shanghai Zelixir Biotech Firm Ltd, Shenzhen Institute of Superior Expertise, Fudan College, Shanghai Synthetic Intelligence Laboratory, Harvard College, MIT, Broad Institute of MIT and Harvard, Arizona State College, and Built-in Biosciences. Designed for correct de novo RNA 3D construction prediction, RhoFold+ leverages an RNA language mannequin pretrained on over 23.7 million sequences and incorporates a number of sequence alignments (MSAs) to deal with information limitations. Validated by way of benchmarks like RNA-Puzzles and CASP15, it predicts secondary constructions and interhelical angles, providing broad applicability in RNA biology and purposeful research.
The RhoFold+ platform combines a number of strategies for RNA construction prediction. It incorporates MSA options utilizing instruments like Infernal and rMSA, which seize co-evolutionary data from RNA sequences. The RNA-FM language mannequin, constructed on a transformer structure much like BERT, is skilled on a big dataset of noncoding RNA sequences from RNAcentral. The mannequin makes use of self-supervised studying, predicting masked nucleotides in sequences. RhoFold+ integrates a construction prediction module that employs a geometry-aware consideration mechanism (IPA) for 3D construction refinement. The mannequin is skilled with numerous loss features, together with MLM, distance loss, and secondary construction loss, for correct RNA construction predictions.
RhoFold+ is a computational software for RNA 3D construction prediction, constructed utilizing RNA-specific insights and information. It leverages a big RNA language mannequin (RNA-FM) for sequence embeddings and MSAs for construction modeling. The mannequin’s efficiency was rigorously benchmarked, displaying superior accuracy in comparison with current strategies in RNA-Puzzles and CASP15 challenges, with a median RMSD of 4.02 Å. RhoFold+ excels at construction prediction, even for unseen sequences, and demonstrates quicker prediction occasions than different strategies. It was examined on numerous RNA constructions, persistently attaining excessive accuracy throughout a number of validation situations.
In conclusion, RhoFold+ is a deep learning-based RNA 3D construction prediction software that integrates an RNA language mannequin pretrained on 23.7 million sequences. It provides a totally automated, differentiable method to RNA construction prediction with out requiring skilled information or computationally intensive processes. RhoFold+ outperforms current strategies in accuracy, significantly for single-strand RNAs, and is efficient in predicting each RNA 3D and secondary constructions. It may generalize throughout completely different datasets and predict unseen RNA constructions. Regardless of its strengths, challenges nonetheless have to be addressed, together with restricted structural variety information, difficulties with giant RNA sequences, and interactions with ligands or proteins. Future enhancements might tackle these limitations.
Take a look at the Paper. All credit score for this analysis goes to the researchers of this challenge. Additionally, don’t neglect to observe us on Twitter and be part of our Telegram Channel and LinkedIn Group. When you like our work, you’ll love our e-newsletter.. Don’t Overlook to hitch our 55k+ ML SubReddit.
[FREE AI VIRTUAL CONFERENCE] SmallCon: Free Digital GenAI Convention ft. Meta, Mistral, Salesforce, Harvey AI & extra. Be a part of us on Dec eleventh for this free digital occasion to study what it takes to construct large with small fashions from AI trailblazers like Meta, Mistral AI, Salesforce, Harvey AI, Upstage, Nubank, Nvidia, Hugging Face, and extra.
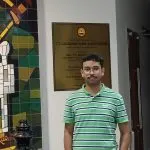
Sana Hassan, a consulting intern at Marktechpost and dual-degree scholar at IIT Madras, is obsessed with making use of expertise and AI to deal with real-world challenges. With a eager curiosity in fixing sensible issues, he brings a recent perspective to the intersection of AI and real-life options.