Imaginative and prescient-language fashions (VLMs) are gaining prominence in synthetic intelligence for his or her capacity to combine visible and textual information. These fashions play an important function in fields like video understanding, human-computer interplay, and multimedia purposes, providing instruments to reply questions, generate captions, and improve decision-making primarily based on video inputs. The demand for environment friendly video-processing techniques is rising as video-based duties proliferate throughout industries, from autonomous techniques to leisure and medical purposes. Regardless of advances, dealing with the huge quantity of visible info in movies stays a core problem in creating scalable and environment friendly VLMs.
A vital problem in video understanding is that current fashions typically depend on processing every video body individually, producing hundreds of visible tokens. This course of consumes in depth computational assets and time, limiting the mannequin’s capacity to effectively deal with lengthy or complicated movies. The problem is lowering the computational load whereas capturing related visible and temporal particulars. And not using a resolution, duties requiring real-time or large-scale video processing turn into impractical, creating a necessity for progressive approaches that steadiness effectivity and accuracy.
Present options try to cut back the variety of visible tokens by means of strategies equivalent to pooling throughout frames. Fashions like Video-ChatGPT and Video-LLaVA deal with spatial and temporal pooling mechanisms to condense frame-level info into smaller tokens. Nonetheless, these strategies nonetheless generate many tokens, with fashions like MiniGPT4-Video and LLaVA-OneVision producing hundreds of tokens, resulting in inefficient dealing with of longer movies. These fashions typically need assistance to optimize token effectivity and video processing efficiency, necessitating simpler options to streamline token administration.
In response, researchers from Salesforce AI Analysis launched BLIP-3-Video, a complicated VLM particularly designed to handle the inefficiencies in video processing. The mannequin incorporates a “temporal encoder” that dramatically reduces the visible tokens required to characterize a video. By limiting the token rely to as few as 16 to 32 tokens, the mannequin considerably improves computational effectivity with out sacrificing efficiency. This breakthrough permits BLIP-3-Video to carry out video-based duties with a lot decrease computational prices, making it a groundbreaking step towards scalable video understanding options.
The temporal encoder in BLIP-3-Video is central to its capacity to course of movies extra effectively. It employs a learnable spatio-temporal attentional pooling mechanism that extracts solely essentially the most informative tokens throughout video frames. The system consolidates spatial and temporal information from every body, remodeling them right into a compact set of video-level tokens. The mannequin features a imaginative and prescient encoder, a frame-level tokenizer, and an autoregressive language mannequin that generates textual content or solutions primarily based on video enter. The temporal encoder makes use of sequential fashions and a spotlight mechanisms to retain the video’s core info whereas lowering redundant information, guaranteeing that BLIP-3-Video can deal with complicated video duties effectively.
Efficiency outcomes show BLIP-3-Video’s superior effectivity in comparison with bigger fashions. The mannequin achieves video question-answering (QA) accuracy much like state-of-the-art fashions, equivalent to Tarsier-34B, whereas utilizing a mere fraction of the visible tokens. For example, Tarsier-34B makes use of 4608 tokens for 8 video frames, whereas BLIP-3-Video reduces this quantity to simply 32 tokens. Regardless of this discount, BLIP-3-Video nonetheless maintains robust efficiency, reaching a rating of 77.7% on the MSVD-QA benchmark and 60.0% on the MSRVTT-QA benchmark, each of that are broadly used datasets for evaluating video-based question-answering duties. These outcomes underscore the mannequin’s capacity to retain excessive ranges of accuracy whereas working with fewer assets.
The mannequin carried out exceptionally properly on multiple-choice question-answering duties, such because the NExT-QA dataset, scoring 77.1%. That is notably noteworthy on condition that it used solely 32 tokens per video, considerably fewer than many competing fashions. Moreover, on the TGIF-QA dataset, which requires understanding dynamic actions and transitions in movies, the mannequin achieved a formidable 77.1% accuracy, additional highlighting its effectivity in dealing with complicated video queries. These outcomes set up BLIP-3-Video as one of the token-efficient fashions out there, offering comparable or superior accuracy to a lot bigger fashions whereas dramatically lowering computational overhead.
In conclusion, BLIP-3-Video addresses the problem of token inefficiency in video processing by introducing an progressive temporal encoder that reduces the variety of visible tokens whereas sustaining excessive efficiency. Developed by Salesforce AI Analysis, the mannequin demonstrates that processing complicated video information with far fewer tokens than beforehand thought essential is feasible, providing a extra scalable and environment friendly resolution for video understanding duties. This development represents a big step ahead in vision-language fashions, paving the best way for extra sensible purposes of AI in video-based techniques throughout varied industries.
Take a look at the Paper and Venture. All credit score for this analysis goes to the researchers of this venture. Additionally, don’t neglect to comply with us on Twitter and be part of our Telegram Channel and LinkedIn Group. When you like our work, you’ll love our publication.. Don’t Overlook to hitch our 55k+ ML SubReddit.
[Upcoming Live Webinar- Oct 29, 2024] The Greatest Platform for Serving High quality-Tuned Fashions: Predibase Inference Engine (Promoted)
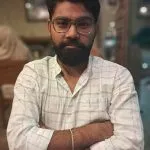
Nikhil is an intern advisor at Marktechpost. He’s pursuing an built-in twin diploma in Supplies on the Indian Institute of Expertise, Kharagpur. Nikhil is an AI/ML fanatic who’s all the time researching purposes in fields like biomaterials and biomedical science. With a robust background in Materials Science, he’s exploring new developments and creating alternatives to contribute.