Multimodal massive language fashions (MLLMs) concentrate on creating synthetic intelligence (AI) methods that may interpret textual and visible knowledge seamlessly. These fashions purpose to bridge the hole between pure language understanding and visible comprehension, permitting machines to cohesively course of numerous types of enter, from textual content paperwork to pictures. Understanding and reasoning throughout a number of modalities is turning into essential, particularly as AI strikes in the direction of extra subtle purposes in areas like picture recognition, pure language processing, and pc imaginative and prescient. By bettering how AI integrates and processes various knowledge sources, MLLMs are set to revolutionize duties reminiscent of picture captioning, doc understanding, and interactive AI methods.
A big problem in growing MLLMs is guaranteeing they carry out equally nicely on text-based and vision-language duties. Typically, enhancements in a single space can result in a decline within the different. For example, enhancing a mannequin’s visible comprehension would possibly negatively have an effect on its language capabilities, which is problematic for purposes requiring each, reminiscent of optical character recognition (OCR) or complicated multimodal reasoning. The important thing difficulty is balancing processing visible knowledge, like high-resolution photographs, and sustaining strong textual content reasoning. As AI purposes turn out to be extra superior, this trade-off turns into a crucial bottleneck within the progress of multimodal AI fashions.
Present approaches to MLLMs, together with fashions reminiscent of GPT-4V and InternVL, have tried to deal with this drawback utilizing numerous architectural strategies. These fashions freeze the language mannequin throughout coaching or make use of cross-attention mechanisms to course of picture and textual content tokens concurrently. Nonetheless, these strategies usually are not with out flaws. Freezing the language mannequin throughout multimodal coaching typically leads to poorer efficiency on vision-language duties. In distinction, open-access fashions like LLaVA-OneVision and InternVL have proven marked degradation in text-only efficiency after multimodal coaching. This displays a persistent difficulty within the discipline, the place developments in a single modality come at the price of one other.
Researchers from NVIDIA have launched the NVLM 1.0 fashions, representing a major leap ahead in multimodal language modeling. The NVLM 1.0 household consists of three fundamental architectures: NVLM-D, NVLM-X, and NVLM-H. Every of those fashions addresses the shortcomings of prior approaches by integrating superior multimodal reasoning capabilities with environment friendly textual content processing. A noteworthy characteristic of NVLM 1.0 is the inclusion of high-quality text-only supervised fine-tuning (SFT) knowledge throughout coaching, which permits these fashions to take care of and even enhance their text-only efficiency whereas excelling in vision-language duties. The analysis group highlighted that their strategy is designed to surpass present proprietary fashions like GPT-4V and open-access options reminiscent of InternVL.
The NVLM 1.0 fashions make use of a hybrid structure to steadiness textual content and picture processing. NVLM-D, the decoder-only mannequin, handles each modalities in a unified method, making it notably adept at multimodal reasoning duties. NVLM-X, however, is constructed utilizing cross-attention mechanisms, which improve computational effectivity when processing high-resolution photographs. The hybrid mannequin, NVLM-H, combines the strengths of each approaches, permitting for extra detailed picture understanding whereas preserving the effectivity wanted for textual content reasoning. These fashions incorporate dynamic tiling for high-resolution pictures, considerably bettering efficiency on OCR-related duties with out sacrificing reasoning capabilities. Integrating a 1-D tile tagging system permits for correct picture token processing, which boosts efficiency in duties like doc understanding and scene textual content studying.
Relating to efficiency, the NVLM 1.0 fashions have achieved spectacular outcomes throughout a number of benchmarks. For example, on text-only duties like MATH and GSM8K, the NVLM-D1.0 72B mannequin noticed a 4.3-point enchancment over its text-only spine, due to integrating high-quality textual content datasets throughout coaching. The fashions additionally demonstrated robust vision-language efficiency, with accuracy scores of 93.6% on the VQAv2 dataset and 87.4% on AI2D for visible query answering and reasoning duties. In OCR-related duties, the NVLM fashions considerably outperformed present methods, scoring 87.4% on DocVQA and 81.7% on ChartQA, highlighting their potential to deal with complicated visible info. These outcomes have been achieved by the NVLM-X and NVLM-H fashions, which demonstrated superior dealing with of high-resolution photographs and multimodal knowledge.
One of many key findings of the analysis is that the NVLM fashions not solely excel in vision-language duties but additionally preserve or enhance their text-only efficiency, one thing that different multimodal fashions wrestle to attain. For instance, in text-based reasoning duties like MMLU, NVLM fashions maintained excessive accuracy ranges, even surpassing their text-only counterparts in some circumstances. That is notably necessary for purposes that require strong textual content comprehension alongside visible knowledge processing, reminiscent of doc evaluation and image-text reasoning. The NVLM-H mannequin, particularly, strikes a steadiness between picture processing effectivity and multimodal reasoning accuracy, making it some of the promising fashions on this discipline.
In conclusion, the NVLM 1.0 fashions developed by researchers at NVIDIA characterize a major breakthrough in multimodal massive language fashions. By integrating high-quality textual content datasets into multimodal coaching and using modern architectural designs like dynamic tiling and tile-tagging for high-resolution photographs, these fashions tackle the crucial problem of balancing textual content and picture processing with out sacrificing efficiency. The NVLM household of fashions not solely outperforms main proprietary methods in vision-language duties but additionally maintains superior text-only reasoning capabilities, marking a brand new frontier within the growth of multimodal AI methods.
Try the Paper. All credit score for this analysis goes to the researchers of this challenge. Additionally, don’t overlook to comply with us on Twitter and be part of our Telegram Channel and LinkedIn Group. When you like our work, you’ll love our e-newsletter..
Don’t Neglect to hitch our 50k+ ML SubReddit
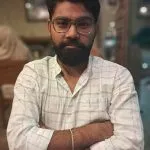
Nikhil is an intern advisor at Marktechpost. He’s pursuing an built-in twin diploma in Supplies on the Indian Institute of Expertise, Kharagpur. Nikhil is an AI/ML fanatic who’s at all times researching purposes in fields like biomaterials and biomedical science. With a robust background in Materials Science, he’s exploring new developments and creating alternatives to contribute.