Multimodal Retrieval Augmented Technology (RAG) expertise has opened new potentialities for synthetic intelligence (AI) functions in manufacturing, engineering, and upkeep industries. These fields rely closely on paperwork that mix advanced textual content and pictures, together with manuals, technical diagrams, and schematics. AI techniques able to deciphering each textual content and visuals have the potential to help intricate, industry-specific duties, however such duties current distinctive challenges. Efficient multimodal information integration can enhance activity accuracy and effectivity in contexts the place visuals are important to understanding advanced directions or configurations.
The AI system’s capacity to offer correct, related solutions utilizing textual content and image-based info from paperwork is a singular problem in industrial settings. Conventional giant language fashions (LLMs) usually want extra domain-specific data and face limitations in dealing with multimodal inputs, resulting in an inclination for ‘hallucinations’ or inaccuracies within the responses generated. As an illustration, in question-answering duties requiring each textual content and pictures, a text-only RAG mannequin might fail to interpret key visible parts like gadget schematics or operational layouts, that are frequent in technical fields. This underscores the necessity for an answer that not solely retrieves textual content information but in addition successfully integrates picture information to enhance the relevance and accuracy of AI-driven insights.
Present retrieval and technology strategies usually deal with both textual content or pictures independently, leading to gaps when dealing with paperwork that require each kinds of enter. Some text-only fashions try to enhance relevance by accessing giant datasets, whereas image-only approaches depend on strategies like optical character recognition or direct embeddings to interpret visuals. Nonetheless, these strategies are restricted in supporting industrial use circumstances the place the mixing of each textual content and picture is essential. Multimodal techniques that may retrieve and course of a number of enter sorts have emerged as an essential development to bridge these gaps. Nonetheless, optimizing such techniques for industrial settings must be explored.
Researchers at LMU Munich, in a collaborative effort with Siemens, have developed a multimodal RAG system particularly designed to handle these challenges inside industrial environments. Their proposed resolution incorporates two multimodal LLMs—GPT-4 Imaginative and prescient and LLaVA—and makes use of two distinct methods to deal with picture information: multimodal embeddings and image-based textual summaries. These methods enable the system to not solely retrieve related pictures based mostly on textual queries but in addition to offer extra contextually correct responses by leveraging each modalities. The multimodal embedding method, using CLIP, aligns textual content and picture information in a shared vector house, whereas the image-summary method converts visuals into descriptive textual content saved alongside different textual information, making certain that each kinds of info can be found for synthesis.
The multimodal RAG system employs these methods to maximise accuracy in retrieving and deciphering information. Within the text-only RAG setting, textual content from industrial paperwork is embedded utilizing a vector-based mannequin and matched to essentially the most related sections for response technology. For image-only RAG, researchers employed CLIP to embed pictures alongside textual questions, making it potential to compute cross-modal similarities and find essentially the most related pictures. In the meantime, the mixed RAG method leverages each modalities, making a extra built-in retrieval course of. The image-summary approach processes pictures into concise textual summaries, facilitating retrieval whereas retaining the unique visuals for reply synthesis. Every method was fastidiously designed to optimize the RAG system’s efficiency, making certain the supply of each textual content and pictures for the LLM to generate a complete response.
The efficiency of the proposed multimodal RAG system demonstrated substantial enhancements, notably in its capability to deal with advanced industrial queries. Outcomes indicated that the multimodal method achieved considerably greater accuracy than text-only or image-only RAG setups, with mixed approaches displaying distinct benefits. As an illustration, accuracy elevated by practically 80% when pictures had been included alongside textual content within the retrieval course of, in comparison with text-only accuracy charges. Moreover, the image-summary methodology proved notably efficient, surpassing the multimodal embedding approach in contextual relevance. The system’s efficiency was measured throughout six key analysis metrics: reply accuracy and contextual alignment. The outcomes confirmed that picture summaries supplied enhanced flexibility and potential for refining the retrieval and technology elements. Additional, the system confronted challenges in picture retrieval high quality, with additional enhancements wanted for totally optimized multimodal RAG.
The analysis group’s work demonstrates that the mixing of multimodal RAG for industrial functions can considerably improve AI efficiency in fields requiring visible and textual interpretation. By addressing the constraints of text-only techniques and introducing progressive strategies for picture processing, the researchers have offered a framework that helps extra correct and contextually applicable solutions to advanced, multimodal queries. The outcomes underscore the potential of multimodal RAG as a crucial software in AI-driven industrial functions, notably as developments in picture retrieval and processing proceed. This potential opens up thrilling potentialities for the way forward for the sphere, inspiring additional analysis and improvement on this space.
Take a look at the Paper.. All credit score for this analysis goes to the researchers of this undertaking. Additionally, don’t overlook to observe us on Twitter and be a part of our Telegram Channel and LinkedIn Group. Should you like our work, you’ll love our publication.. Don’t Overlook to affix our 55k+ ML SubReddit.
[Trending] LLMWare Introduces Mannequin Depot: An In depth Assortment of Small Language Fashions (SLMs) for Intel PCs
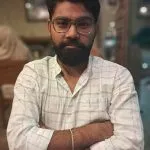
Nikhil is an intern guide at Marktechpost. He’s pursuing an built-in twin diploma in Supplies on the Indian Institute of Expertise, Kharagpur. Nikhil is an AI/ML fanatic who’s all the time researching functions in fields like biomaterials and biomedical science. With a powerful background in Materials Science, he’s exploring new developments and creating alternatives to contribute.