Synthetic intelligence has progressed from dealing with atomic duties to addressing intricate, real-world issues requiring the mixing of a number of specialised fashions. This method, generally known as AI pipelines, permits for seamless process transitions by connecting totally different fashions to course of various knowledge inputs and outputs. These pipelines allow advanced functions like multilingual video dubbing, multimodal content material moderation, and superior speech translation. The rising sophistication of AI pipelines displays the rising want for automated options that simplify and streamline difficult computational duties in numerous domains.
Addressing advanced computational challenges requires coordinating a number of fashions to deal with totally different points of an issue. Present options usually fall quick when confronted with ambiguous person necessities, poorly outlined process parameters, and mismatched knowledge modalities. As an illustration, computational duties like multilingual dubbing demand cautious alignment of inputs and outputs, akin to matching audio transcription to translation fashions and text-to-speech synthesis. Such complexities make guide intervention essential, slowing progress and resulting in inefficiencies.
Current strategies for constructing AI pipelines usually depend on static frameworks and predefined fashions tailor-made to particular duties. Whereas these approaches can deal with remoted issues successfully, they lack adaptability. Guide changes are often required to deal with lacking data, guarantee semantic alignment, or resolve errors arising from mismatched modalities. Furthermore, the rigidity of present methods limits their means to cater to various person queries, leaving important room for enchancment in each flexibility and accuracy.
Researchers from aiXplain, Inc. and Los Gatos launched a novel AI framework known as Bel Esprit to beat these challenges. This multi-agent system facilitates constructing customizable AI mannequin pipelines tailor-made to person wants. Bel Esprit options specialised subagents, together with Mentalist for clarifying person queries, Builder for pipeline meeting, and Inspector for error detection and correction. By using a collaborative and iterative method, the framework ensures pipelines are correct and aligned with person intent. The system is designed to work dynamically, refining person inputs and optimizing the fashions chosen for particular duties.
Bel Esprit is a graph-based framework with nodes representing AI features and edges representing knowledge flows. The Mentalist subagent begins by analyzing person queries to make clear ambiguous particulars, changing them into complete process specs. Builder then constructs an preliminary pipeline, breaking the duty into manageable subgraphs. For instance, distinct branches are created for every language in a multilingual dubbing process. The inspector evaluations the pipeline for structural and semantic errors, making certain alignment with the refined person necessities. This iterative course of leverages strategies like chain-of-branches, the place smaller subgraphs are constructed sequentially, facilitating mannequin reuse and minimizing errors. Additional, Bel Esprit integrates superior massive language fashions (LLMs) to automate reasoning and guarantee seamless process execution.
The efficiency of Bel Esprit demonstrates its important potential for remodeling pipeline building. The system achieved appreciable outcomes utilizing precise match (EM) and graph edit distance (GED) metrics. The general EM charge elevated by 9.5%, indicating a better charge of completely constructed pipelines. GED errors decreased by 28.1%, showcasing enhancements in lowering discrepancies between generated and reference pipelines. As an illustration, when utilized to multilingual video dubbing, Bel Esprit optimized workflows by reusing AI nodes, akin to automated speech recognition (ASR) fashions, throughout branches for various languages. This led to a streamlined pipeline building course of with fewer errors. Additionally, Bel Esprit successfully dealt with ambiguous person queries, with efficiency enhancements being extra pronounced in instances the place person enter lacked readability. The system’s iterative course of ensured alignment with person intent, even in extremely advanced eventualities.
Bel Esprit considerably advances AI pipeline building, addressing key ambiguity points and error-prone meeting processes. Its progressive multi-agent collaboration, iterative refinement, and state-of-the-art fashions make it a strong resolution for advanced computational duties. Bel Esprit units a brand new benchmark for adaptability and precision within the subject by automating essential levels of pipeline constructing and making certain semantic accuracy. The framework’s demonstrated means to enhance effectivity and deal with advanced queries underscores its potential as a transformative device in AI functions.
Try the Paper. All credit score for this analysis goes to the researchers of this venture. Additionally, don’t overlook to comply with us on Twitter and be part of our Telegram Channel and LinkedIn Group. Don’t Neglect to hitch our 60k+ ML SubReddit.
🚨 Trending: LG AI Analysis Releases EXAONE 3.5: Three Open-Supply Bilingual Frontier AI-level Fashions Delivering Unmatched Instruction Following and Lengthy Context Understanding for International Management in Generative AI Excellence….
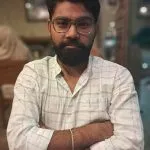
Nikhil is an intern marketing consultant at Marktechpost. He’s pursuing an built-in twin diploma in Supplies on the Indian Institute of Expertise, Kharagpur. Nikhil is an AI/ML fanatic who’s at all times researching functions in fields like biomaterials and biomedical science. With a robust background in Materials Science, he’s exploring new developments and creating alternatives to contribute.