Machine studying focuses on creating fashions that may study from giant datasets to enhance their predictions and decision-making skills. One of many core areas of growth inside machine studying is neural networks, that are particularly crucial for duties comparable to picture recognition, language processing, and autonomous decision-making. These fashions are ruled by scaling legal guidelines, suggesting that rising mannequin measurement and the quantity of coaching information enhances efficiency. Nevertheless, this enchancment will depend on the information high quality used, notably when artificial information is included within the coaching course of.
A rising downside in machine studying is the degradation of mannequin efficiency when artificial information is utilized in coaching. Not like real-world information, artificial information can fail to seize the complexity and richness of pure datasets. This problem results in what researchers seek advice from as “mannequin collapse,” the place the mannequin begins to overfit on artificial patterns. These patterns don’t totally symbolize the variability of real-world information, main the mannequin to strengthen errors and biases. This ends in a compromised capacity to generalize to new, unseen real-world information, making the mannequin much less dependable in sensible functions.
At the moment, machine studying fashions are sometimes skilled on datasets that mix actual and artificial information to scale the scale of the coaching set. Whereas this strategy permits for bigger datasets, the blended information technique presents a number of challenges. The analysis group highlights that whereas scaling fashions utilizing artificial information could appear useful, together with low-quality artificial information results in mannequin collapse, undermining the advantages of elevated information. Changes comparable to information weighting or selective information mixing have been explored however haven’t constantly prevented mannequin collapse.
Researchers from Meta, the NYU Middle for Information Science, NYU Courant Institute, and UCLA launched a complete evaluation of the mannequin collapse phenomenon. The examine explored whether or not mixing actual and artificial information may forestall collapse, and so they examined this utilizing numerous mannequin sizes and information proportions. The findings point out that even a small fraction of artificial information (as little as 1% of the full dataset) can result in mannequin collapse, with bigger fashions being extra prone to this problem. This implies that greater than conventional strategies of mixing actual and artificial information are wanted to counteract the issue.
The analysis group employed linear regression fashions and random projections to analyze how mannequin measurement and information high quality influence mannequin collapse. They demonstrated that bigger fashions are likely to exacerbate collapse when skilled on artificial information, considerably deviating from actual information distributions. Though there are conditions the place rising mannequin measurement could barely mitigate the collapse, it doesn’t fully forestall the issue. The outcomes are notably regarding given the rising reliance on artificial information in large-scale AI methods.
The experiments on image-based fashions utilizing the MNIST dataset and language fashions skilled on BabiStories confirmed that mannequin efficiency deteriorates when introducing artificial information. For instance, in exams involving language fashions, the group discovered that artificial information, even when blended with actual information, resulted in a considerable decline in efficiency. Because the proportion of artificial information elevated, the check error grew, confirming the severity of the mannequin collapse phenomenon. In some circumstances, bigger fashions displayed extra pronounced errors, amplifying the biases and errors inherent in artificial information, resulting in worse outcomes than smaller fashions.
In conclusion, the examine emphasizes the dangers of utilizing artificial information to coach giant fashions. Mannequin collapse presents a crucial problem affecting neural networks’ scalability and reliability. Whereas the analysis affords priceless insights into the mechanisms behind mannequin collapse, it additionally means that present strategies for mixing actual and artificial information are inadequate. Extra superior methods are wanted to make sure that fashions skilled on artificial information can nonetheless generalize successfully to real-world eventualities.
Take a look at the Paper. All credit score for this analysis goes to the researchers of this mission. Additionally, don’t overlook to comply with us on Twitter and be a part of our Telegram Channel and LinkedIn Group. If you happen to like our work, you’ll love our e-newsletter.. Don’t Neglect to hitch our 50k+ ML SubReddit.
[Upcoming Live Webinar- Oct 29, 2024] The Greatest Platform for Serving Superb-Tuned Fashions: Predibase Inference Engine (Promoted)
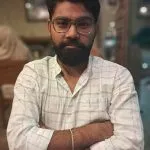
Nikhil is an intern guide at Marktechpost. He’s pursuing an built-in twin diploma in Supplies on the Indian Institute of Expertise, Kharagpur. Nikhil is an AI/ML fanatic who’s at all times researching functions in fields like biomaterials and biomedical science. With a robust background in Materials Science, he’s exploring new developments and creating alternatives to contribute.