Synthetic intelligence has considerably superior by integrating organic rules, resembling evolution, into machine studying fashions. Evolutionary algorithms, impressed by pure choice and genetic mutation, are generally used to optimize advanced methods. These algorithms refine populations of potential options over generations based mostly on health, resulting in environment friendly adaptation in difficult environments. Equally, diffusion fashions in AI work by progressively denoising noisy enter information to realize structured outputs. These fashions iteratively enhance preliminary noisy information factors, guiding them towards extra coherent outcomes that match the coaching information distribution. Combining these two domains has the potential to provide novel strategies that make the most of the strengths of each approaches, thereby enhancing their effectiveness.
One of many main challenges in evolutionary computation is its tendency to converge prematurely on single options in advanced, high-dimensional areas. Conventional evolutionary algorithms resembling Covariance Matrix Adaptation Evolution Technique (CMA-ES) and Parameter-Exploring Coverage Gradients (PEPG) successfully optimize less complicated issues however are likely to get trapped in native optima when utilized to extra advanced situations. This limitation makes it tough for these algorithms to discover numerous potential options. In consequence, they typically fail to keep up the range wanted to resolve multi-modal optimization duties successfully, highlighting the necessity for extra superior strategies able to balancing exploration and exploitation.
Conventional evolutionary strategies like CMA-ES and PEPG use choice, crossover, and mutation processes to boost the inhabitants’s health over successive generations. Nonetheless, these algorithms need assistance to carry out effectively when confronted with high-dimensional or multi-modal health landscapes. For example, CMA-ES is liable to converge on a single resolution even when a number of high-fitness areas are within the search area. This limitation is basically because of its lack of ability to keep up resolution range, making it difficult to adapt to advanced optimization issues with many attainable options. To beat these limitations, there’s a want for extra versatile and sturdy evolutionary algorithms that may deal with advanced and numerous optimization duties.
Researchers from the Allen Discovery Heart at Tufts College, the Institute for Theoretical Physics at TU Wien, and the Wyss Institute at Harvard College launched a novel strategy referred to as Diffusion Evolution. This algorithm merges evolutionary rules with diffusion fashions by treating evolution as a denoising course of. The analysis group demonstrated that the algorithm may incorporate evolutionary ideas like pure choice, mutation, and reproductive isolation. Constructing on this concept, the group launched the Latent House Diffusion Evolution technique, which reduces computational prices by mapping high-dimensional parameter areas into lower-dimensional latent areas. This course of permits for extra environment friendly searches whereas sustaining the power to search out numerous and optimum options throughout advanced health landscapes.
The Diffusion Evolution algorithm uniquely integrates the iterative denoising steps of diffusion fashions with pure choice processes. By mapping high-dimensional parameter areas into lower-dimensional latent areas, the tactic performs extra environment friendly searches, figuring out a number of numerous options inside the identical search area. This strategy enhances the algorithm’s robustness, making it appropriate for addressing varied optimization challenges. Utilizing latent area diffusion helps overcome conventional strategies’ sluggish convergence and native optima points, resulting in quicker convergence and better resolution range. This technique permits the algorithm to keep up flexibility in exploring advanced search areas with out compromising efficiency.
In a sequence of experiments, the researchers in contrast Diffusion Evolution with different outstanding evolutionary algorithms like CMA-ES, OpenES, and PEPG utilizing a number of benchmark features: Rosenbrock, Beale, Himmelblau, Ackley, and Rastrigin. On features like Himmelblau and Rastrigin, which have a number of optimum factors, Diffusion Evolution demonstrated its functionality to search out numerous options whereas sustaining excessive health scores. For instance, on the Ackley operate, Diffusion Evolution achieved a mean entropy of two.49 with a health rating of 1.00, outperforming CMA-ES, which achieved a better entropy of three.96 however with decrease health scores, indicating it was distracted by a number of high-fitness peaks. Moreover, the experiments demonstrated that Diffusion Evolution required fewer iterations to realize optimum options than CMA-ES and PEPG. For example, Latent House Diffusion Evolution diminished the computational steps considerably in high-dimensional areas, dealing with duties with as much as 17,410 parameters successfully.

In reinforcement studying duties, resembling balancing a cart-pole system, the Diffusion Evolution algorithm confirmed promising outcomes. The system consists of a cart with a pole hinged to it, and the target is to maintain the pole vertical so long as attainable by shifting the cart left or proper based mostly on inputs like place and velocity. The analysis group used a two-layer neural community with 58 parameters to regulate the cart, and the algorithm achieved a cumulative reward of 500 persistently, indicating profitable efficiency. This demonstrates that Diffusion Evolution can successfully deal with advanced reinforcement studying environments, highlighting its sensible functions in real-world situations.
In conclusion, the Diffusion Evolution algorithm considerably advances evolutionary computation by integrating diffusion fashions’ strengths. This strategy improves the power to keep up resolution range and enhances total problem-solving capabilities in advanced optimization duties. By introducing Latent House Diffusion Evolution, the researchers supplied a strong framework able to fixing high-dimensional issues with diminished computational prices. The algorithm’s success in numerous benchmark features and reinforcement studying duties signifies its potential to revolutionize evolutionary computation in AI and past.
Take a look at the Paper. All credit score for this analysis goes to the researchers of this challenge. Additionally, don’t overlook to comply with us on Twitter and be a part of our Telegram Channel and LinkedIn Group. In case you like our work, you’ll love our publication.. Don’t Neglect to affix our 50k+ ML SubReddit
[Upcoming Event- Oct 17 202] RetrieveX – The GenAI Information Retrieval Convention (Promoted)
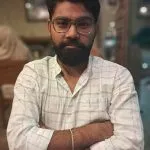
Nikhil is an intern advisor at Marktechpost. He’s pursuing an built-in twin diploma in Supplies on the Indian Institute of Expertise, Kharagpur. Nikhil is an AI/ML fanatic who’s all the time researching functions in fields like biomaterials and biomedical science. With a powerful background in Materials Science, he’s exploring new developments and creating alternatives to contribute.