Probabilistic diffusion fashions have turn into important for producing advanced knowledge constructions reminiscent of pictures & movies. These fashions remodel random noise into structured knowledge, attaining excessive realism and utility throughout varied domains. The mannequin operates via two phases: a ahead section that regularly corrupts knowledge with noise and a reverse section that systematically reconstructs coherent knowledge. Regardless of the promising outcomes, these fashions usually require quite a few denoising steps and face inefficiencies in balancing sampling high quality with computational velocity, motivating researchers to hunt methods to streamline these processes.
A significant drawback with current diffusion fashions is the necessity for extra environment friendly manufacturing of high-quality samples. This limitation primarily arises from the intensive variety of steps required within the reverse course of and the mounted or variably discovered covariance settings, which don’t adequately optimize output high quality relative to time and computational assets. Decreasing covariance prediction errors may velocity up the sampling course of whereas sustaining output integrity. Addressing this, researchers search to refine these covariance approximations for extra environment friendly and correct modeling.
Typical approaches like Denoising Diffusion Probabilistic Fashions (DDPM) deal with noise by making use of predetermined noise schedules or studying covariance by way of variational decrease bounds. Just lately, state-of-the-art fashions have moved in direction of straight studying covariance to reinforce output high quality. Nonetheless, these strategies include computational burdens, particularly in high-dimensional purposes the place the info requires intensive calculations. Such limitations hinder the fashions’ sensible software throughout domains needing high-resolution or advanced knowledge synthesis.
The analysis staff from Imperial Faculty London, College Faculty London, and the College of Cambridge launched an revolutionary method referred to as Optimum Covariance Matching (OCM). This methodology redefines the covariance estimation by straight deriving the diagonal covariance from the rating operate of the mannequin, eliminating the necessity for data-driven approximations. By regressing the optimum covariance analytically, OCM reduces prediction errors and enhances sampling high quality, serving to to beat limitations related to mounted or variably discovered covariance matrices. OCM represents a big step ahead by simplifying the covariance estimation course of with out compromising accuracy.
The OCM methodology affords a streamlined method to estimating covariance by coaching a neural community to foretell the diagonal Hessian, which permits for correct covariance approximation with minimal computational calls for. Conventional fashions usually require the calculation of a Hessian matrix, which might be computationally exhaustive in high-dimensional purposes, reminiscent of massive picture or video datasets. OCM bypasses these intensive calculations, decreasing each storage necessities and computation time. Utilizing a score-based operate to approximate covariance improves prediction accuracy whereas maintaining computational calls for low, making certain sensible viability for high-dimensional purposes. This score-based method in OCM not solely makes covariance predictions extra correct but in addition reduces the general time required for the sampling course of.
Efficiency assessments display the numerous enhancements OCM introduced within the high quality and effectivity of generated samples. As an example, when examined on the CIFAR10 dataset, OCM achieved a Frechet Inception Distance (FID) rating of 38.88 for 5 denoising steps, outperforming the normal DDPM, which recorded an FID rating of 58.28. With ten denoising steps, the OCM method additional improved, attaining a rating of 21.60 in comparison with DDPM’s 34.76. These outcomes point out that OCM enhances pattern high quality and reduces the computational load by requiring fewer steps to attain comparable or higher outcomes. The analysis additionally revealed that OCM’s probability analysis improved considerably. Utilizing fewer than 20 steps, OCM achieved a damaging log-likelihood (NLL) of 4.43, surpassing typical DDPMs, which generally require 20 steps or extra to succeed in an NLL of 6.06. This elevated effectivity means that OCM’s score-based covariance estimation could possibly be an efficient different in each Markovian and non-Markovian diffusion fashions, decreasing time and computational assets with out compromising high quality.
This analysis highlights an revolutionary methodology of optimizing covariance estimation to ship high-quality knowledge technology with decreased steps and enhanced effectivity. By leveraging the score-based method in OCM, the analysis staff gives a balanced resolution to the challenges in diffusion modeling, merging computational effectivity with excessive output high quality. This development might considerably influence purposes the place speedy, high-quality knowledge technology is important.
Try the Paper and GitHub. All credit score for this analysis goes to the researchers of this challenge. Additionally, don’t overlook to comply with us on Twitter and be a part of our Telegram Channel and LinkedIn Group. If you happen to like our work, you’ll love our publication.. Don’t Overlook to hitch our 55k+ ML SubReddit.
[Upcoming Live Webinar- Oct 29, 2024] The Greatest Platform for Serving Wonderful-Tuned Fashions: Predibase Inference Engine (Promoted)
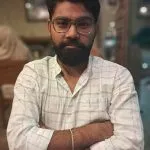
Nikhil is an intern marketing consultant at Marktechpost. He’s pursuing an built-in twin diploma in Supplies on the Indian Institute of Know-how, Kharagpur. Nikhil is an AI/ML fanatic who’s at all times researching purposes in fields like biomaterials and biomedical science. With a powerful background in Materials Science, he’s exploring new developments and creating alternatives to contribute.