Massive language fashions (LLMs) have quickly superior multimodal massive language fashions (LMMs), significantly in vision-language duties. Movies characterize complicated, information-rich sources essential for understanding real-world eventualities. Nonetheless, present video-language fashions encounter important challenges in temporal localization and exact second detection. Regardless of in depth coaching in video captioning and question-answering datasets, these fashions wrestle to determine and reference particular temporal segments inside video content material. The basic limitation lies of their incapacity to exactly search and extract related info from massive redundant video supplies. This problem turns into more and more crucial because the demand for evidence-based, moment-specific video evaluation will increase.
Present analysis on video-language fashions has explored a number of approaches to bridge visible and language understanding. Massive image-language fashions initially targeted on using picture encoders in language fashions, with strategies like BLIP utilizing learnable question transformers to attach visible and language domains. Preliminary strategies, like Video-LLaVA’s 8-frame sampling approach, uniformly chosen a set variety of frames however struggled with processing longer movies. Superior methods like LongVU and Kangaroo developed adaptive compression mechanisms to cut back visible tokens throughout spatial and temporal dimensions. Nonetheless, present fashions nonetheless face important challenges in precisely capturing and representing temporal nuances in video content material.
To this finish, researchers from Meituan Inc. have proposed TimeMarker, a novel video-language mannequin designed to handle temporal localization challenges in video understanding. TimeMarker introduces modern methods to reinforce semantic notion and temporal consciousness in video content material. The mannequin integrates Temporal Separator Tokens to mark particular moments inside movies exactly and implements an AnyLength mechanism for dynamic body sampling. TimeMarker can successfully course of brief and lengthy video sequences utilizing adaptive token merging. Furthermore, it makes use of numerous datasets, together with reworked temporal-related video question-answering datasets, to enhance the mannequin’s understanding of temporal nuances.
TimeMarker’s structure is basically primarily based on the LLaVA framework, using a Imaginative and prescient Encoder to course of video frames and a cross-modality Projector to translate visible tokens into the language area. The mannequin introduces two key modern elements: Temporal Separator Tokens Integration and the AnyLength mechanism. Temporal Separator Tokens are strategically inserted with video body tokens, enabling the LLM to acknowledge and encode absolute temporal positions inside the video. The AnyLength mechanism coupled with an Adaptive Token Merge module, permits the mannequin to deal with movies of various lengths effectively. This method ensures versatile and exact temporal understanding throughout completely different video content material varieties.
TimeMarker demonstrates distinctive efficiency throughout numerous temporal understanding duties. Researchers included the experimental outcomes of Quick and Basic Video Analysis, Lengthy Video Analysis, and the Results of Temporal Separator Tokens. The mannequin reveals superior temporal consciousness in experimental evaluations by precisely figuring out clock digits, finding particular occasions, and reasoning about temporal contexts in multi-turn dialogues from a 2-minute life-record video. It precisely identifies clock digits, finding related occasions, and reasoning about one thing unusual. Furthermore, TimeMarker can carry out OCR duties sequentially inside a specified time interval.
On this paper, researchers from Meituan Inc. launched TimeMarker which represents a major development in video-language fashions, addressing crucial challenges in temporal localization and video understanding. By introducing Temporal Separator Tokens and the AnyLength mechanism, the mannequin successfully encodes temporal positions and adapts to movies of various lengths. Its modern method permits exact occasion detection, temporal reasoning, and complete video evaluation throughout completely different content material varieties. The mannequin’s superior efficiency throughout a number of benchmarks demonstrates its potential to rework video-language interplay, setting a brand new commonplace for temporal understanding in multimodal AI methods.
Try the Paper and GitHub Web page. All credit score for this analysis goes to the researchers of this challenge. Additionally, don’t neglect to comply with us on Twitter and be part of our Telegram Channel and LinkedIn Group. Should you like our work, you’ll love our e-newsletter.. Don’t Neglect to affix our 60k+ ML SubReddit.
🚨 [Must Attend Webinar]: ‘Remodel proofs-of-concept into production-ready AI purposes and brokers’ (Promoted)
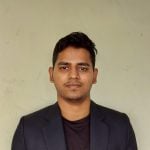
Sajjad Ansari is a last yr undergraduate from IIT Kharagpur. As a Tech fanatic, he delves into the sensible purposes of AI with a concentrate on understanding the influence of AI applied sciences and their real-world implications. He goals to articulate complicated AI ideas in a transparent and accessible method.