Molecule discovery is essential in numerous scientific analysis fields, significantly prescribed drugs and supplies science. Whereas the emergence of Graph Neural Networks (GNNs) has revolutionized this discipline by enabling the illustration of molecules as graphs and facilitating property predictions, it faces difficulties in generalizing throughout totally different duties, requiring substantial task-specific information assortment. These approaches present limitations in producing molecules with custom-made properties. The combination of LLMs into molecule discovery faces hurdles in successfully aligning molecular and textual information together with challenges in dataset availability and analysis metrics that seize the elements of latest molecule discovery.
Numerous synthetic intelligence approaches have been developed to boost molecule discovery. Integration of machine studying, deep studying, and pure language processing has enabled extra advanced evaluation of organic and chemical information. Strategies like Convolutional Neural Networks (CNNs) for structural evaluation, Recurrent Neural Networks (RNNs) for sequential information processing, and Transformer-based networks for advanced sample recognition. Textual content-based Molecule Era (Text2Mol) emerged as a useful strategy, using pure language descriptions for molecule retrieval. Whereas fashions like MolT5 present preliminary success with SMILES string technology, subsequent developments like KVPLM, MoMu, and 3DMoLM enhanced capabilities utilizing molecular graphs and spatial configurations.
Researchers from The Hong Kong Polytechnic College, Shanghai Jiao Tong College, and Shanghai AI Lab have proposed TOMG-Bench (Textual content-based Open Molecule Era Benchmark), the primary complete benchmark designed to guage LLMs’ capabilities in open-domain molecule technology. It introduces three main duties: molecule modifying (MolEdit), molecule optimization (MolOpt), and customised molecule technology (MolCustom), with every job additional divided into three subtasks containing 5,000 check samples. Researchers additionally developed an automatic analysis system to guage the standard and accuracy of generated molecules. By way of in depth testing of 25 LLMs, TOMG-Bench reveals essential insights into present limitations in text-guided molecule discovery.
The TOMG-Bench analysis framework makes use of 4 distinct classes of fashions. The primary class, proprietary fashions, consists of industrial API-accessible techniques like GPT-4-turbo, GPT-3.5-turbo, Claude-3.5, Claude-3, and Gemini-1.5-pro. The second class options open-source basic LLMs with instruction-following capabilities, together with numerous variations of Llama-3, Mistral-7B, Qwen2-7B, yi-1.5-9B, and chatglm-9B. The third class consists of LLMs fine-tuned on the ChEBI-20 dataset, together with totally different variations of MolT5 and BioT5-base. The ultimate class focuses on OpenMolIns fine-tuned LLMs, that includes Galactica-125M, Llama3.2-1B-Instruct, and Llama-3.1-8B-Instruct, with Galactica-125M being examined throughout 5 totally different information sizes of OpenMolIns.
The analysis outcomes from TOMG-Bench present that Claude-3.5 emerged as the highest performer with a weighted common accuracy of 35.92%, adopted intently by Gemini-1.5-pro at 34.80%. Additional, open-source fashions present outstanding progress, with Llama-3-70B-Instruct reaching 23.93% accuracy, outperforming GPT-3.5-turbo’s 18.58%. Nonetheless, fashions educated particularly on the ChEBI-20 dataset present restricted effectiveness, with BioT5-base, regardless of being the claimed state-of-the-art mannequin for text-based molecule technology, reaching solely 4.21% weighted common accuracy. These fashions significantly struggled with molecular modifying operations and customised molecule technology duties.
On this paper, the researchers launched TOMG-Bench, a benchmark for evaluating LLMs’ capabilities in open-domain molecule technology. By way of complete testing of 25 LLMs, the benchmark has successfully highlighted each the restrictions of current molecule technology approaches and the promising potential of basic LLMs on this discipline. The profitable implementation of OpenMolIns instruction tuning has proven outstanding enhancements, enabling fashions to attain efficiency ranges similar to GPT-3.5-turbo. Nonetheless, it faces sure limitations, like inadequate immediate range which may result in instruction overfitting, and potential inaccuracies within the distribution of molecular elements comparable to atoms, bonds, and practical teams in comparison with real-world situations.
Try the Paper. All credit score for this analysis goes to the researchers of this challenge. Additionally, don’t overlook to comply with us on Twitter and be part of our Telegram Channel and LinkedIn Group. Don’t Overlook to affix our 60k+ ML SubReddit.
🚨 Trending: LG AI Analysis Releases EXAONE 3.5: Three Open-Supply Bilingual Frontier AI-level Fashions Delivering Unmatched Instruction Following and Lengthy Context Understanding for International Management in Generative AI Excellence….
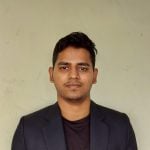
Sajjad Ansari is a remaining yr undergraduate from IIT Kharagpur. As a Tech fanatic, he delves into the sensible functions of AI with a deal with understanding the impression of AI applied sciences and their real-world implications. He goals to articulate advanced AI ideas in a transparent and accessible method.