Pure Language Processing (NLP) has superior considerably with deep studying, pushed by improvements like phrase embeddings and transformer architectures. Self-supervised studying makes use of huge quantities of unlabeled knowledge to create pretraining duties and has turn into a key strategy for coaching fashions, particularly in high-resource languages like English and Chinese language. The disparity in NLP assets and efficiency ranges from high-resource language programs, corresponding to English and Chinese language, to low-resource language programs, corresponding to Portuguese, and greater than 7000 languages worldwide. Such a spot hinders the flexibility of NLP purposes of low-resource languages to develop and be extra sturdy and accessible. Additionally, low-resource monolingual fashions stay small-scale and undocumented, and so they lack commonplace benchmarks, which makes growth and analysis troublesome.
Present growth strategies typically make the most of huge quantities of information and computational assets available for high-resource languages like English and Chinese language. Portuguese NLP largely makes use of multilingual fashions like mBERT, mT5, and BLOOM or fine-tunes English-trained fashions. Nevertheless, these strategies typically miss the distinctive facets of Portuguese. The analysis benchmarks are both previous or based mostly on English datasets, making them much less helpful for Portuguese.
To deal with this, researchers from the College of Bonn have developed GigaVerbo, a large-scale Portuguese textual content corpus of 200 billion tokens, and skilled a collection of decoder-transformers named Tucano. These fashions intention to enhance the efficiency of Portuguese language fashions by leveraging a considerable and high-quality dataset.
The GigaVerbo dataset is a concatenation of a number of high-quality Portuguese textual content corpora, refined utilizing customized filtering methods based mostly on GPT-4 evaluations. The filtering course of improved textual content preprocessing, retaining 70% of the dataset for the mannequin. Primarily based on the Llama structure, the Tucano fashions had been applied utilizing Hugging Face for simple neighborhood entry. Strategies corresponding to RoPE embeddings, root imply sq. normalization, and Silu activations as a substitute of SwiGLU had been used. The coaching was finished utilizing a causal language modeling strategy and cross-entropy loss. The fashions vary from 160M to 2.4B parameters, with the biggest skilled on 515 billion tokens.
The analysis of those fashions exhibits that they carry out equal to or higher than different Portuguese and multilingual language fashions of comparable measurement on a number of Portuguese benchmarks. The coaching loss and validation perplexity curves for the 4 base fashions confirmed that bigger fashions typically lowered loss and perplexity extra successfully, with the impact amplified by bigger batch sizes. Checkpoints had been saved each 10.5 billion tokens, and efficiency was tracked throughout a number of benchmarks. Pearson correlation coefficients indicated blended outcomes: some benchmarks, like CALAME-PT, LAMBADA, and HellaSwag, improved with scaling, whereas others, such because the OAB Exams, confirmed no correlation with token ingestion. Inverse scaling was noticed in sub-billion parameter fashions, suggesting potential limitations. Efficiency benchmarks additionally reveal that Tucano outperforms multilingual and prior Portuguese fashions on native evaluations like CALAME-PT and machine-translated assessments like LAMBADA.
In conclusion, the GigaVerbo and the Tucano collection improve the efficiency of Portuguese language fashions. The proposed work lined the event pipeline, which included dataset creation, filtration, hyperparameter tuning, and analysis, with a give attention to openness and reproducibility. It additionally confirmed the potential for bettering low-resource language fashions by large-scale knowledge assortment and superior coaching methods. The contribution of those researchers will show helpful in offering these essential assets to information future research.
Try the Paper and Hugging Face Web page. All credit score for this analysis goes to the researchers of this undertaking. Additionally, don’t overlook to comply with us on Twitter and be part of our Telegram Channel and LinkedIn Group. Should you like our work, you’ll love our e-newsletter.. Don’t Overlook to hitch our 55k+ ML SubReddit.
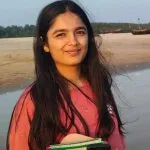
Nazmi Syed is a consulting intern at MarktechPost and is pursuing a Bachelor of Science diploma on the Indian Institute of Expertise (IIT) Kharagpur. She has a deep ardour for Knowledge Science and actively explores the wide-ranging purposes of synthetic intelligence throughout numerous industries. Fascinated by technological developments, Nazmi is dedicated to understanding and implementing cutting-edge improvements in real-world contexts.