Synthetic intelligence (AI) has develop into a transformative know-how in lots of fields, significantly by way of chatbots in numerous customer support, schooling, and leisure purposes. These chatbots work together with thousands and thousands of customers every day, producing huge quantities of dialog information. Learning this information presents important alternatives for understanding consumer habits, enhancing chatbot algorithms, and enhancing the general interplay expertise. Nevertheless, analyzing such massive datasets is a posh job, requiring superior instruments to handle and extract significant insights from the overwhelming info effectively.
One of many key challenges researchers face on this space is the issue of analyzing large-scale chat logs generated by thousands and thousands of interactions. With such huge datasets, it turns into virtually unimaginable to manually evaluation particular person conversations and even determine patterns by way of typical strategies. Necessary insights into consumer habits, chatbot efficiency, and potential misuse are more likely to stay hidden with out acceptable instruments. Environment friendly evaluation of this information is important to uncover traits, enhance system designs, and guarantee accountable utilization of AI applied sciences.
At present, instruments out there for analyzing chatbot logs are restricted of their capability to deal with million-scale datasets. Many current strategies concentrate on smaller-scale information, which is insufficient for the dimensions and complexity of interactions generated by fashionable chatbots like ChatGPT. Whereas instruments corresponding to ConvoKit and others present some performance for analyzing dialogue, they’re typically not scalable or user-friendly sufficient for analyzing huge datasets. Moreover, they lack superior options like interactive visualizations that permit researchers to discover massive datasets simply.
Researchers from the College of Waterloo, Cornell College, Samaya AI, the College of Southern California, the College of Washington, and Nvidia, in a collaborative effort, have developed WILDVIS, a brand new open-source device for analyzing large-scale chat logs. The researchers launched WILDVIS as an interactive visualizer able to managing thousands and thousands of chatbot conversations. With WILDVIS, researchers can search, filter, and visualize conversations primarily based on standards like geographical information, language, toxicity, and mannequin kind. This analyzes large-scale chatbot datasets extra accessible and effectively, opening up new alternatives for analysis into consumer chatbot interactions.
WILDVIS is constructed utilizing a number of key applied sciences that allow its scalability and responsiveness. The device makes use of Elasticsearch for scalable search performance, effectively retrieving related conversations from huge datasets. Additional, the system implements precomputed embeddings and caching mechanisms to make sure that searches and visualizations could be carried out inside seconds, even when coping with thousands and thousands of information factors. The structure of WILDVIS consists of each frontend and backend optimizations, making certain easy consumer interactions. Customers can discover conversations by way of a filter-based search interface or an embedding-based visualization web page, the place related discussions are positioned shut collectively on a 2D map. This method offers high-level overviews of datasets and the power to drill down into particular dialog particulars.
When it comes to efficiency, WILDVIS has demonstrated outstanding effectivity in dealing with large-scale information. Throughout testing, search queries executed on the filter-based search web page had a mean execution time of 0.47 seconds, and the embedding visualization web page processed queries in a mean of 0.43 seconds. The system has been designed to scale successfully, with optimizations corresponding to pagination and embedding precomputation decreasing the computational load. WILDVIS can visualize as much as 1,500 conversations in a single view whereas sustaining readability and responsiveness. In a single case research, the device analyzed thousands and thousands of conversations from two massive datasets—WildChat and LMSYS-Chat-1M—inside seconds, highlighting its scalability.
One key discovering from WILDVIS’s software in real-world analysis is its potential to uncover distinct patterns and anomalies in dialog information. For instance, when evaluating two datasets, researchers discovered that WildChat had extra artistic writing-focused conversations, whereas LMSYS-Chat-1M contained a better focus of chemistry-related discussions. This potential to rapidly determine and examine subject clusters makes WILDVIS a strong device for researchers finding out chatbot misuse, user-specific behaviors, and subject distributions throughout totally different datasets. By filtering conversations primarily based on particular standards corresponding to IP deal with or consumer location, researchers may additionally monitor patterns in particular person consumer interactions, resulting in new insights into how chatbots are used throughout totally different demographics.
In conclusion, WILDVIS represents a big development in analyzing large-scale chatbot datasets. By introducing highly effective search and visualization instruments, researchers from establishments such because the College of Waterloo, Cornell College, Nvidia, and the College of Washington have created a system that’s not solely scalable but additionally extremely responsive. The device’s potential to uncover patterns, examine datasets, and monitor user-specific behaviors makes it a invaluable useful resource for researchers seeking to deepen their understanding of consumer chatbot interactions. By addressing the challenges of large-scale information evaluation, WILDVIS opens up new avenues for exploring the dynamics of human-AI interplay and enhancing the efficiency and accountability of chatbot techniques.
Take a look at the Paper. All credit score for this analysis goes to the researchers of this venture. Additionally, don’t overlook to comply with us on Twitter and be part of our Telegram Channel and LinkedIn Group. For those who like our work, you’ll love our e-newsletter..
Don’t Neglect to affix our 50k+ ML SubReddit
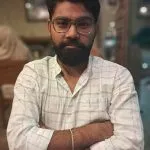
Nikhil is an intern guide at Marktechpost. He’s pursuing an built-in twin diploma in Supplies on the Indian Institute of Know-how, Kharagpur. Nikhil is an AI/ML fanatic who’s all the time researching purposes in fields like biomaterials and biomedical science. With a powerful background in Materials Science, he’s exploring new developments and creating alternatives to contribute.