Hypergraphs, which lengthen conventional graphs by permitting hyperedges to attach a number of nodes, provide a richer illustration of advanced relationships in fields like social networks, bioinformatics, and recommender techniques. Regardless of their versatility, producing lifelike hypergraphs is difficult attributable to their complexity and the necessity for efficient generative fashions. Whereas conventional strategies deal with algorithmic technology with predefined properties, deep studying for hypergraph technology nonetheless must be explored. On account of their variable hyperedge sizes, present graph technology strategies, corresponding to one-shot and iterative fashions, need assistance with hypergraphs. Current developments goal to deal with these challenges by leveraging spectral equivalences and hierarchical enlargement strategies to seize hypergraph constructions higher.
Researchers from LTCI, Télécom Paris, and Institut Polytechnique de Paris have developed a hypergraph technology methodology known as HYGENE, which addresses the challenges of making lifelike hypergraphs via a diffusion-based strategy. HYGENE operates on a bipartite hypergraph illustration, beginning with a fundamental pair of related nodes and increasing iteratively utilizing a denoising diffusion course of. This methodology constructs the worldwide hypergraph construction whereas refining native particulars. HYGENE is the primary deep learning-based hypergraph technology mannequin validated on each artificial and real-world datasets. Key contributions embrace pioneering deep studying strategies for hypergraphs, adapting graph ideas to hypergraphs, and offering strong theoretical and empirical validations.
Graph technology utilizing deep studying started with GraphVAE, which makes use of autoencoders to embed and generate graphs. Subsequent developments included utilizing recurrent neural networks to enhance adjacency matrix technology and adapting diffusion fashions for graph technology. A notable departure concerned reversing a coarsening course of, the place graphs are progressively simplified and reconstructed. In distinction to those strategies, HYGENE addresses hypergraph technology, extending the idea to higher-order constructions. Not like sequential edge prediction, HYGENE employs a hierarchical strategy that focuses on predicting the quantity and composition of hyperedges, providing a extra nuanced methodology for producing advanced hypergraphs.
The tactic outlined entails producing hypergraphs by studying from present hypergraph datasets. The strategy begins with a bipartite graph illustration, utilizing a weighted clique and star enlargement. The method contains coarsening, simplifying the hypergraph by merging nodes and edges whereas preserving spectral properties, and increasing, which entails duplicating nodes and refining connections to reconstruct the hypergraph. The mannequin employs a denoising diffusion framework to get better unique options from noisy knowledge and makes use of spectral conditioning to make sure correct reconstruction. The tactic iteratively refines the bipartite illustration to realize high-quality hypergraph technology.
The examine outlines the experimental setup, together with datasets and analysis metrics. HYGENE is in contrast with baselines corresponding to HyperPA, a Variational Autoencoder (VAE), a Generative Adversarial Community (GAN), and a regular 2D diffusion mannequin. The experiments goal to exhibit that HYGENE can generate the specified hyperedge distributions, replicate structural properties, and validate the significance of elements like spectrum-preserving coarsening and hyperedge higher bounds. Analysis entails 4 artificial hypergraph datasets and three ModelNet40 subsets. Outcomes point out that HYGENE excels in structural accuracy and compliance with hypergraph properties. Ablation research spotlight some great benefits of the proposed strategy.
In conclusion, HYGENE represents the primary deep learning-based strategy for hypergraph technology, enhancing earlier iterative native enlargement and coarsening strategies. It employs a diffusion-based method, beginning with related nodes and increasing them iteratively to assemble hypergraphs. The method makes use of a denoising diffusion mannequin so as to add nodes and hyperedges, progressively refining world and native constructions. HYGENE successfully generates hypergraphs from particular distributions, addressing the challenges of their inherent complexity. This work marks a big development in graph technology, offering a basis for future analysis in hypergraph modeling throughout numerous domains.
Try the Paper. All credit score for this analysis goes to the researchers of this venture. Additionally, don’t overlook to observe us on Twitter and LinkedIn. Be a part of our Telegram Channel. For those who like our work, you’ll love our publication..
Don’t Overlook to hitch our 50k+ ML SubReddit
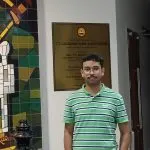
Sana Hassan, a consulting intern at Marktechpost and dual-degree scholar at IIT Madras, is obsessed with making use of know-how and AI to deal with real-world challenges. With a eager curiosity in fixing sensible issues, he brings a recent perspective to the intersection of AI and real-life options.